Mecanismos de Atención e Interpretabilidad en el Aprendizaje Automático para la Detección de Enfermedades Oculares a través del Uso de Tomografías
dc.contributor.advisor | Gil Romero, Robert | spa |
dc.contributor.author | Alba Acosta, David Camilo | spa |
dc.contributor.corporatename | Universidad Santo Tomás | spa |
dc.contributor.cvlac | https://scienti.minciencias.gov.co/cvlac/visualizador/generarCurriculoCv.do?cod_rh=0000157060 | spa |
dc.contributor.googlescholar | https://scholar.google.com/citations?user=LUEZqaYAAAAJ&hl=es | spa |
dc.contributor.orcid | https://orcid.org/0000-0001-8602-6890 | spa |
dc.coverage.campus | CRAI-USTA Bogotá | spa |
dc.date.accessioned | 2021-02-04T21:01:10Z | spa |
dc.date.available | 2021-02-04T21:01:10Z | spa |
dc.date.issued | 2021-02-04 | spa |
dc.description | El Deep learning o “aprendizaje profundo” vino para quedarse por mucho tiempo; es así que, en los últimos años producto del desarrollo de la inteligencia artificial, su actuar ha venido aplicándose en varias áreas del conocimiento principalmente en las ciencias de la salud. Ante este fenómeno señalado, la medicina tradicionalmente ha venido desarrollando los diagnósticos de enfermedades como de traumatologías, incorporando el uso de rayos x, resonancias magnéticas y tomografías entre otras. En ese sentido, la ciencia de la medicina ha venido implementando sistemas autónomos, los cuales, han venido a complementar la forma de analizar la información médica. La ciencia médica hoy en día viene desarrollando en sus diferentes áreas del conocimiento la aplicación de la inteligencia artificial mediante el uso del deep learning, como es el caso de la oftalmología y de la optometría. De conformidad con lo explicitado, el trabajo propuesto tiene como objetivo principal: Encontrar la mejor arquitectura CNN “Convolutional neural network” que se adapte a un conjunto de datos que contiene tomografías oculares en la región de la retina, y que sea capaz de clasificar estos cuatro tipos de diagnóstico ocular: Normal, Drusen, CNV (Neurovascularización coroidea) y DME(Edema Macular diabético). | spa |
dc.description.abstract | Deep learning is here to stay for a long time; thus, in recent years, as a result of the development of artificial intelligence, it has been applied in several areas of knowledge, mainly in the health sciences. Faced with this phenomenon, medicine has traditionally been developing the diagnosis of diseases such as traumatology, incorporating the use of x-rays, magnetic resonance imaging and tomography, among others. In this sense, the science of medicine has been implementing autonomous systems, which have come to complement the way of analyzing medical information. Medical science today is developing in its different areas of knowledge the application of artificial intelligence through the use of deep learning, as is the case of ophthalmology and optometry. In accordance with the above, the main objective of the proposed work is: To find the best CNN architecture "Convolutional neural network" that adapts to a dataset containing ocular tomographies in the retina region, and that is able to classify these four types of ocular diagnosis: Normal, Drusen, CNV (Choroidal Neurovascularization) and DME (Diabetic Macular Edema). | Eng |
dc.description.degreelevel | Pregrado | spa |
dc.description.degreename | Profesional en estadística | spa |
dc.description.domain | http://unidadinvestigacion.usta.edu.co | spa |
dc.format.mimetype | application/pdf | spa |
dc.identifier.citation | Alba Acosta, D. C. (2021). Mecanismos de Atención e Interpretabilidad en el Aprendizaje Automático para la Detección de Enfermedades Oculares a través del Uso de Tomografías. [Trabajo de Grado, Universidad Santo Tomás]. Repositorio Institucional. | spa |
dc.identifier.instname | instname:Universidad Santo Tomás | spa |
dc.identifier.reponame | reponame:Repositorio Institucional Universidad Santo Tomás | spa |
dc.identifier.repourl | repourl:https://repository.usta.edu.co | spa |
dc.identifier.uri | http://hdl.handle.net/11634/31964 | |
dc.language.iso | spa | spa |
dc.publisher | Universidad Santo Tomás | spa |
dc.publisher.faculty | Facultad de Estadística | spa |
dc.publisher.program | Pregrado Estadística | spa |
dc.relation.references | Alom, M. Z., Taha, T. M., Yakopcic, C., Westberg, S., Sidike, P., Nasrin, M. S., Hasan, M., Van Essen, B. C., Awwal, A. A., and Asari, V. K. (2019). A state-of-the-art survey on deep learning theory and architectures. Electronics, 8(3):292. | spa |
dc.relation.references | Browning, D. J., Stewart, M. W., and Lee, C. (2018). Diabetic macular edema: evidence-based management. Indian journal of ophthalmology, 66(12):1737–1738. | spa |
dc.relation.references | Cortes, C. and Vapnik, V. (1995). Support-vector networks. Machine learning, 20(3):273–297. | spa |
dc.relation.references | Denker, J. S. and LeCun, Y. (1991). Transforming neural-net output levels to probability distributions. In Advances in neural information processing systems, pages 853–859. | spa |
dc.relation.references | Goodfellow, I., Bengio, Y., and Courville, A. (2016a). Deep learning. MIT press. | spa |
dc.relation.references | Goodfellow, I., Bengio, Y., and Courville, A. (2016b). Deep Learning. MIT Press. http://www .deeplearningbook.org. | spa |
dc.relation.references | Hu, J., Shen, L., and Sun, G. (2018). Squeeze-andexcitation networks. In Proceedings of the IEEE conference on computer vision and pattern recognition, pages 7133–7134. | spa |
dc.relation.references | Huang, G., Liu, Z., Van Der Maaten, L., and Weinberger, K. Q. (2017). Densely connected convolutional networks. In Proceedings of the IEEE conference on computer vision and pattern recognition, pages 4700–4708. | spa |
dc.relation.references | Ioffe, S. and Szegedy, C. (2015). Batch normalization: Accelerating deep network training by reducing internal covariate shift. arXiv preprint ar- Xiv:1502.03167. | spa |
dc.relation.references | Jaiswal, T. and Jaiswal, S. (2019). Deep learning in medicine. | spa |
dc.relation.references | Kermany, D. S., Goldbaum, M., Cai, W., Valentim, C. C., Liang, H., Baxter, S. L., McKeown, A., Yang, G., Wu, X., Yan, F., et al. (2018). Identifying medical diagnoses and treatable diseases by image-based deep learning. Cell, 172(5):1122– 1131. | spa |
dc.relation.references | Kingma, D. P. and Ba, J. (2014). Adam: A method for stochastic optimization. arXiv preprint ar- Xiv:1412.6980. | spa |
dc.relation.references | Nielsen, M. A. (2015). Neural networks and deep learning, volume 2018. Determination press San Francisco, CA. | spa |
dc.relation.references | Ravì, D., Wong, C., Deligianni, F., Berthelot, M., Andreu-Perez, J., Lo, B., and Yang, G.-Z. (2016). Deep learning for health informatics. IEEE journal of biomedical and health informatics, 21(1):4–21. | spa |
dc.relation.references | Razzak, M. I., Naz, S., and Zaib, A. (2018). Deep learning for medical image processing: Overview, challenges and the future. In Classification in Bio- Apps, pages 323–350. Springer. | spa |
dc.relation.references | Ribeiro, M. T., Singh, S., and Guestrin, C. (2016). "why should i trust you?.explaining the predictions of any classifier. In Proceedings of the 22nd ACM SIGKDD international conference on knowledge discovery and data mining, pages 1135–1144. | spa |
dc.relation.references | Ruder, S. (2016). An overview of gradient descent optimization algorithms. arXiv preprint ar- Xiv:1609.04747, page 4. | spa |
dc.relation.references | Sarkar, D. (2018). A comprehensive hands-on guide to transfer learning with real-world applications in deep learning. | spa |
dc.relation.references | Williams, M., Craig, D., Passmore, P., and Silvestri, G. (2009). Retinal drusen: harbingers of age, safe havens for trouble. Age and ageing, 38(6):648–649. | spa |
dc.relation.references | Woo, S., Park, J., Lee, J.-Y., and So Kweon, I. (2018). Cbam: Convolutional block attention module. In Proceedings of the European conference on computer vision (ECCV), pages 3–19. | spa |
dc.relation.references | Yeo, N. J. Y., Chan, E. J. J., and Cheung, C. (2019). Choroidal neovascularization: Mechanisms of endothelial dysfunction. Frontiers in Pharmacology, 10:1–2. | spa |
dc.relation.references | ZhangLabData (2018). Large dataset of labeled optical coherence tomography (oct) and chest x-ray images. | spa |
dc.rights | Atribución-NoComercial-SinDerivadas 2.5 Colombia | * |
dc.rights.accessrights | info:eu-repo/semantics/openAccess | |
dc.rights.coar | http://purl.org/coar/access_right/c_abf2 | |
dc.rights.local | Abierto (Texto Completo) | spa |
dc.rights.uri | http://creativecommons.org/licenses/by-nc-nd/2.5/co/ | * |
dc.subject.keyword | Deep learning | Eng |
dc.subject.keyword | CNN | Eng |
dc.subject.keyword | Neural networks | Eng |
dc.subject.keyword | Attention modules | Eng |
dc.subject.keyword | Ocular diseases | Eng |
dc.subject.lemb | Enfermedades de los ojos | spa |
dc.subject.lemb | Oftalmología | spa |
dc.subject.lemb | Estadística | spa |
dc.subject.lemb | Tomografía | spa |
dc.subject.proposal | Aprendizaje profundo | spa |
dc.subject.proposal | Redes neuronales | spa |
dc.subject.proposal | CNN | spa |
dc.subject.proposal | Módulos de atención | spa |
dc.subject.proposal | Enfermedades oculares | spa |
dc.title | Mecanismos de Atención e Interpretabilidad en el Aprendizaje Automático para la Detección de Enfermedades Oculares a través del Uso de Tomografías | spa |
dc.type | bachelor thesis | |
dc.type.category | Formación de Recurso Humano para la Ctel: Trabajo de grado de Pregrado | spa |
dc.type.coar | http://purl.org/coar/resource_type/c_7a1f | |
dc.type.coarversion | http://purl.org/coar/version/c_ab4af688f83e57aa | |
dc.type.drive | info:eu-repo/semantics/bachelorThesis | |
dc.type.local | Tesis de pregrado | spa |
dc.type.version | info:eu-repo/semantics/acceptedVersion |
Archivos
Bloque original
1 - 3 de 3
Cargando...
- Nombre:
- 2020davidalba
- Tamaño:
- 1.64 MB
- Formato:
- Adobe Portable Document Format
- Descripción:
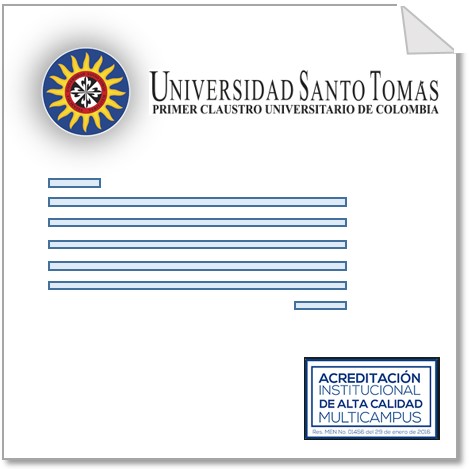
- Nombre:
- 2020cartaaprobaciónfacultad
- Tamaño:
- 301.06 KB
- Formato:
- Adobe Portable Document Format
- Descripción:
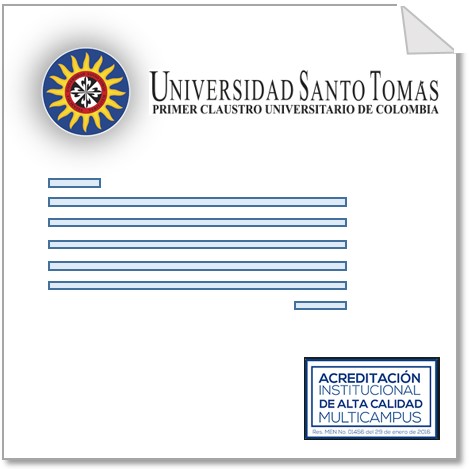
- Nombre:
- 2020cartaderechosautor
- Tamaño:
- 340.61 KB
- Formato:
- Adobe Portable Document Format
- Descripción:
Bloque de licencias
1 - 1 de 1
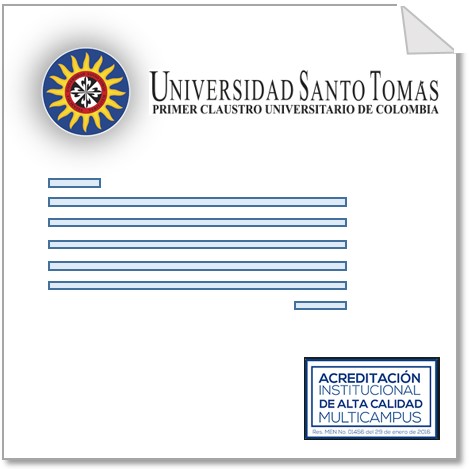
- Nombre:
- license.txt
- Tamaño:
- 807 B
- Formato:
- Item-specific license agreed upon to submission
- Descripción: