Extensión del Algoritmo ClustImpute para Variables Cualitativas y Mixtas: Una Aplicación al Capítulo de Cultivos de Bogotá D.C. del III Censo Nacional Agropecuario
dc.contributor.advisor | Pacheco Lopéz, Mario José | Spa |
dc.contributor.author | Rojas Pulido, William Camilo | Spa |
dc.contributor.corporatename | Universidad Santo Tomás | spa |
dc.contributor.cvlac | https://scienti.minciencias.gov.co/cvlac/visualizador/generarCurriculoCv.do?cod_rh=0000775479 | spa |
dc.contributor.googlescholar | https://scholar.google.com/citations?hl=es&user=a5SEoPgAAAAJ | spa |
dc.contributor.orcid | https://orcid.org/0000-0003-4752-703X | spa |
dc.coverage.campus | CRAI-USTA Bogotá | spa |
dc.date.accessioned | 2024-01-24T13:31:35Z | |
dc.date.available | 2024-01-24T13:31:35Z | |
dc.date.issued | 2023 | |
dc.description | En el actual contexto colombiano, caracterizado por cambios demográficos, económicos y ambientales, así como la importancia del sector agropecuario en la economía, la realización del III Censo Nacional Agropecuario en 2014 se presenta como una herramienta fundamental para comprender las complejas variables que impactan este sector. Con una cobertura operativa del 98.9%, este censo proporcionó información detallada y actualizada sobre el sector agropecuario en todo el país, incluyendo municipios, territorios indígenas, tierras de comunidades negras y parques nacionales. Sin embargo, el análisis de datos presenta desafíos, como el 4% de registros con datos faltantes, los cuales se abordan mediante enfoques estadísticos como la imputación de valores faltantes. En este contexto, el trabajo se enfoca en el capítulo de Cultivos en Bogotá, proponiendo la aplicación de una extensión del algoritmo ClustImpute. Este algoritmo, combinando técnicas de imputación con el método de k-medias, busca abordar tanto variables cuantitativas como cualitativas presentes en el censo, ofreciendo una alternativa innovadora a los métodos convencionales de imputación. El objetivo final es proporcionar un análisis de datos más completo y fiable para contribuir a la comprensión y mejora de las políticas y esfuerzos destinados al desarrollo rural y la calidad de vida en áreas rurales en Bogotá y, por extensión, en el conjunto del país. | spa |
dc.description.abstract | In the current Colombian context marked by demographic, economic, and environmental changes, coupled with the significant role of the agricultural sector in the economy, the implementation of the III National Agricultural Census in 2014 emerges as a crucial tool to comprehend the multifaceted variables impacting this sector. With an operational coverage of 98.9%, this census provided detailed and updated information on the agricultural sector nationwide, including municipalities, indigenous territories, lands of black communities, and national parks. However, data analysis presents challenges such as the existence of 4% of records with missing data, which are addressed through statistical approaches like missing value imputation. Focusing on the Cultivation chapter in Bogotá, this work proposes the application of an extended version of the ClustImpute algorithm. By combining imputation techniques with the k-means method, this algorithm aims to address both quantitative and qualitative variables present in the census, offering an innovative alternative to conventional imputation methods. The ultimate goal is to provide a more comprehensive and reliable data analysis to contribute to the understanding and improvement of policies and efforts related to rural development and the quality of life in rural areas in Bogotá and, consequently, throughout the country. | Eng |
dc.description.degreelevel | Pregrado | spa |
dc.description.degreename | Profesional en estadística | spa |
dc.format.mimetype | application/pdf | spa |
dc.identifier.citation | Rojas Pulido, W. C. (2023). Extensión del Algoritmo ClustImpute para Variables Cualitativas y Mixtas: Una Aplicación al Capítulo de Cultivos de Bogotá D.C. del III Censo Nacional Agropecuario. [Trabajo de Grado, Universidad Santo Tomás]. Repositorio Institucional. | spa |
dc.identifier.instname | instname:Universidad Santo Tomás | spa |
dc.identifier.reponame | reponame:Repositorio Institucional Universidad Santo Tomás | spa |
dc.identifier.repourl | repourl:https://repository.usta.edu.co | spa |
dc.identifier.uri | http://hdl.handle.net/11634/53694 | |
dc.language.iso | spa | spa |
dc.publisher | Universidad Santo Tomás | spa |
dc.publisher.faculty | Facultad de estadística | spa |
dc.publisher.program | Rregrado estadística | spa |
dc.relation.references | Alfonso, O. A. y Barrera, R. A. (2019). El ciclo mortal de los habitantes de calle en Bogotá. Revista de Economía Institucional, 21(41), julio-diciembre. | spa |
dc.relation.references | Arteaga, F. y Ferrer-Riquelme, A. (2009). "Missing data". En S.D. Brown, R. Tauler y B. Walczak (Eds.), Comprehensive Chemometrics. Elsevier, Oxford, pp. 285-314. | spa |
dc.relation.references | Azur, M. J. (2011). Multiple imputation by chained equations: what is it and how does it work? International Journal of Methods in Psychiatric Research, 20(1), 40-49. | spa |
dc.relation.references | Bai, L., Cao, F., & Liang, J. (2009). A new initialization method for categorical data grouping. Expert Systems with Applications, 36(3), 5992-5998. | spa |
dc.relation.references | Cao, L., & Zhao, X. (2016). A grouping-based imputation approach to missing data in a fault detection system. Neurocomputing, 173, 693-703. | spa |
dc.relation.references | Pfaffel, Oliver. (2020). CLUSTIMPUTE: AN R PACKAGE FOR K-MEANS CLUSTERING WITH BUILD-IN MISSING DATA IMPUTATION. | spa |
dc.relation.references | Hastie, T., Tibshirani, R., & Friedman, J. (2009). The Elements of Statistical Learning: Data Mining, Inference, and Prediction (2nd ed.). Springer. | spa |
dc.relation.references | Huang, Z. (1997). A fast grouping algorithm to group very large categorical data sets in data mining. Data Mining and Knowledge Discovery, 1(3), 275-288. | spa |
dc.relation.references | Huang, Z. (1998). Extensions to the k-modes algorithm for grouping large data sets with categorical values. Data Mining and Knowledge Discovery, 2(3), 283-304. | spa |
dc.relation.references | Hwang, J. T., & Lee, J. D. (2010). A grouping-based imputation method for missing data. Computational Statistics & Data Analysis, 54(12), 3095-3107. | spa |
dc.relation.references | Jain, A. K., Murty, M. N., & Flynn, P. J. (1999). Data clustering: A review. ACM Computing Surveys (CSUR), 31(3), 264-323. | spa |
dc.relation.references | Li, J. (2019). A novel grouping-based imputation algorithm for mixed data. Neurocomputing, 331, 322-329. | spa |
dc.relation.references | Little, R. J. A., D’Agostino, R., Cohen, M. L., Dickersin, K., Emerson, S. S., Farrar, J. T., ... Stern, H. (2012). The prevention and treatment of missing data in clinical trials. New England Journal of Medicine, 367(14), 1355–1360. | spa |
dc.relation.references | van Buuren, S. (2011). "mice: Multivariate imputation by chained equations in R". Journal of Statistical Software, 45, 1-67. | spa |
dc.relation.references | McClain, J. O., & Rao, V. R. (1975). Clustering and classification in marketing research. Journal of Marketing Research, 12(2), 129-134. | spa |
dc.relation.references | Xiao-Hua, Z. (2019). Challenges and strategies in analysis of missing data. Biostatistics Epidemiology, 4, 15–23 | spa |
dc.relation.references | Zhao, X., Zhang, S., Wu, X., & Chen, J. (2015). Group-based missing value imputation. Information Sciences, 314, 85-101. | spa |
dc.relation.references | Zhao, Y., & Liu, H. (2014). Grouping-based multiple imputations for missing data. Information Sciences, 265, 1-12. | spa |
dc.rights | Atribución-NoComercial-SinDerivadas 2.5 Colombia | * |
dc.rights | Atribución-NoComercial-SinDerivadas 2.5 Colombia | * |
dc.rights | Atribución-NoComercial-SinDerivadas 2.5 Colombia | * |
dc.rights.accessrights | info:eu-repo/semantics/openAccess | |
dc.rights.coar | http://purl.org/coar/access_right/c_abf2 | spa |
dc.rights.local | Abierto (Texto Completo) | spa |
dc.rights.local | Abierto (Texto Completo) | spa |
dc.rights.uri | http://creativecommons.org/licenses/by-nc-nd/2.5/co/ | * |
dc.subject.keyword | k-prototypes | spa |
dc.subject.keyword | k-means | spa |
dc.subject.keyword | Clustimpute | Eng |
dc.subject.keyword | Agriculture | Eng |
dc.subject.keyword | Variables | Eng |
dc.subject.keyword | Mixed | Eng |
dc.subject.keyword | Mixed | Eng |
dc.subject.keyword | missing values | Eng |
dc.subject.keyword | Imputation | Eng |
dc.subject.keyword | Algorithm | Eng |
dc.subject.keyword | Clustering | Eng |
dc.subject.keyword | Qualitative | Eng |
dc.subject.lemb | Estadística | spa |
dc.subject.lemb | Datos Estadísticos | spa |
dc.subject.lemb | Investigación | spa |
dc.subject.proposal | Algoritmo | spa |
dc.subject.proposal | Agrupamiento | spa |
dc.subject.proposal | Imputacion | spa |
dc.subject.proposal | Valores Faltantes | spa |
dc.subject.proposal | Cualitativas | spa |
dc.subject.proposal | Mixtas | spa |
dc.subject.proposal | Variables | spa |
dc.subject.proposal | Agropecuario | spa |
dc.subject.proposal | Clustimpute | spa |
dc.subject.proposal | k-means | spa |
dc.subject.proposal | k-prototipos | spa |
dc.title | Extensión del Algoritmo ClustImpute para Variables Cualitativas y Mixtas: Una Aplicación al Capítulo de Cultivos de Bogotá D.C. del III Censo Nacional Agropecuario | spa |
dc.type.coar | http://purl.org/coar/resource_type/c_7a1f | |
dc.type.coarversion | http://purl.org/coar/version/c_ab4af688f83e57aa | |
dc.type.drive | info:eu-repo/semantics/bachelorThesis | |
dc.type.version | info:eu-repo/semantics/acceptedVersion |
Archivos
Bloque original
1 - 3 de 3
Cargando...
- Nombre:
- 2023williamrojas
- Tamaño:
- 595.03 KB
- Formato:
- Adobe Portable Document Format
- Descripción:
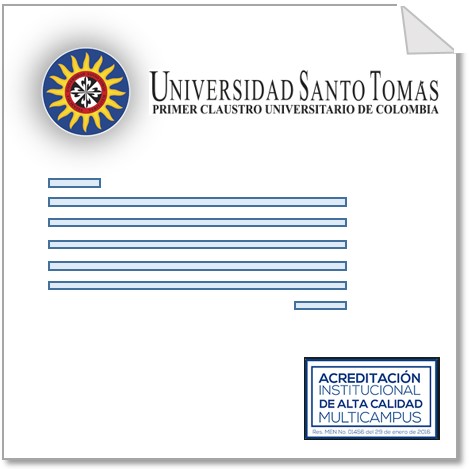
- Nombre:
- 2023cartaaprobaciónfacultad
- Tamaño:
- 140.59 KB
- Formato:
- Adobe Portable Document Format
- Descripción:
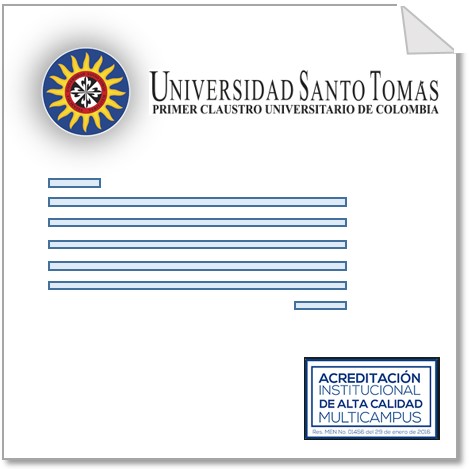
- Nombre:
- 2023cartaderechosautor
- Tamaño:
- 151.87 KB
- Formato:
- Adobe Portable Document Format
- Descripción:
Bloque de licencias
1 - 1 de 1
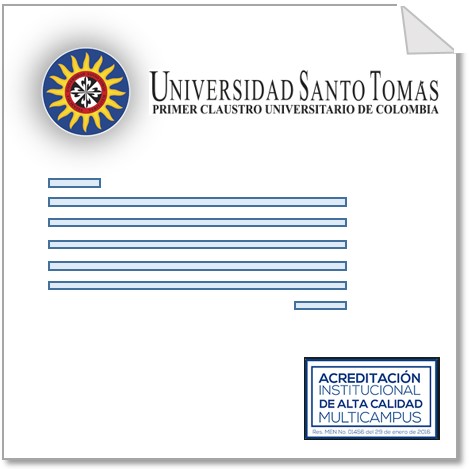
- Nombre:
- license.txt
- Tamaño:
- 807 B
- Formato:
- Item-specific license agreed upon to submission
- Descripción: