Uso de Drones en la Agricultura de Precisión
dc.contributor.advisor | Vela Beltrán, Diego Alejandro | |
dc.contributor.author | Otálora Moreno, William David | |
dc.contributor.corporatename | Universidad Santo Tomás | spa |
dc.contributor.cvlac | https://scienti.minciencias.gov.co/cvlac/visualizador/generarCurriculoCv.do?cod_rh=0001977029 | Spa |
dc.contributor.googlescholar | https://scholar.google.com/citations?hl=es&user=o-A8ASAAAAAJ | Spa |
dc.contributor.orcid | https://orcid.org/0009-0003-1809-4033 | Spa |
dc.coverage.campus | CRAI-USTA Tunja | spa |
dc.date.accessioned | 2024-06-19T19:41:05Z | |
dc.date.available | 2024-06-19T19:41:05Z | |
dc.date.issued | 2024 | |
dc.description | El artículo investiga el creciente papel de los drones en la agricultura de precisión, destacando sus aplicaciones y avances tecnológicos. Los drones permiten la monitorización detallada y eficiente de cultivos, mejorando la gestión agrícola. Se discuten desafíos técnicos como la integración de datos y la interoperabilidad del software, así como la necesidad de colaboración entre investigadores, empresas y agricultores para superar estos obstáculos. También se subraya la importancia de abordar cuestiones éticas y legales para una implementación responsable de esta tecnología. | spa |
dc.description.abstract | The article investigates the growing role of drones in precision agriculture, highlighting their applications and technological advancements. Drones enable detailed and efficient crop monitoring, improving agricultural management. Technical challenges such as data integration and software interoperability are discussed, along with the need for collaboration among researchers, companies, and farmers to overcome these obstacles. The importance of addressing ethical and legal issues for the responsible implementation of this technology is also emphasized. | spa |
dc.description.degreelevel | Pregrado | spa |
dc.description.degreename | Ingeniero Informático | spa |
dc.format.mimetype | application/pdf | spa |
dc.identifier.citation | Otálora, W. (2024). Uso de Drones en la Agricultura de Precisión- [Trabajo de Grado, Universidad Santo Tomás]. Repositorio Institucional. | spa |
dc.identifier.instname | instname:Universidad Santo Tomás | spa |
dc.identifier.reponame | reponame:Repositorio Institucional Universidad Santo Tomás | spa |
dc.identifier.repourl | repourl:https://repository.usta.edu.co | spa |
dc.identifier.uri | http://hdl.handle.net/11634/55664 | |
dc.language.iso | spa | spa |
dc.publisher | Universidad Santo Tomás | spa |
dc.publisher.faculty | Facultad de Ingeniería de Sistemas | spa |
dc.publisher.program | Ingeniería Informática | spa |
dc.relation.references | “Boletin+Introducción+a+la+agricultura+de+precisión+ganadería+de+leche+ener o”. | spa |
dc.relation.references | E. Pino V., “Los drones una herramienta para una agricultura eficiente: un futuro de alta tecnología,” Idesia (Arica), no. ahead, pp. 0–0, 2019, doi: 10.4067/s0718- 34292019005000402. | spa |
dc.relation.references | P. Fernández and P. Díaz, “Investigación: Investigación cuantitativa y cualitativa Investigación cuantitativa y cualitativa.” [Online]. Available: www.fisterra.com | spa |
dc.relation.references | H. Análisis Cualitativo Héctor Cárcamo Vásquez, “CINTA DE MOEBIO.” | spa |
dc.relation.references | Y. Liu et al., “Estimating potato above-ground biomass by using integrated unmanned aerial system-based optical, structural, and textural canopy measurements,” Comput Electron Agric, vol. 213, p. 108229, Oct. 2023, doi: 10.1016/J.COMPAG.2023.108229. | spa |
dc.relation.references | S. H. Gurmani, H. Garg, R. M. Zulqarnain, and I. Siddique, “Selection of Unmanned Aerial Vehicles for Precision Agriculture Using Interval-Valued q-Rung Orthopair Fuzzy Information based TOPSIS Method,” International Journal of Fuzzy Systems, vol. 25, no. 8, pp. 2939–2953, Nov. 2023, doi: 10.1007/s40815- 023-01568-0. | spa |
dc.relation.references | Y. Zhang et al., “CURI-YOLOv7: A Lightweight YOLOv7tiny Target Detector for Citrus Trees from UAV Remote Sensing Imagery Based on Embedded Device,” Remote Sens (Basel), vol. 15, no. 19, Oct. 2023, doi: 10.3390/rs15194647. | spa |
dc.relation.references | H. Huang, K. Li, W. Ni, H. Kurunathan, and E. Hossain, “Machine Learning-Aided Operations and Communications of Unmanned Aerial Vehicles: A Contemporary Survey”, doi: 10.13140/RG.2.2.10212.65928. | spa |
dc.relation.references | P. Marques, L. Pádua, J. J. Sousa, and A. Fernandes-Silva, “Assessing the Water Status and Leaf Pigment Content of Olive Trees: Evaluating the Potential and Feasibility of Unmanned Aerial Vehicle Multispectral and Thermal Data for Estimation Purposes,” Remote Sens (Basel), vol. 15, no. 19, Oct. 2023, doi: 10.3390/rs15194777. | spa |
dc.relation.references | F. Ecer, İ. Y. Ögel, R. Krishankumar, and E. B. Tirkolaee, “The q-rung fuzzy LOPCOW-VIKOR model to assess the role of unmanned aerial vehicles for precision agriculture realization in the Agri-Food 4.0 era,” Artif Intell Rev, vol. 56, no. 11, pp. 13373–13406, Nov. 2023, doi: 10.1007/s10462-023-10476-6. | spa |
dc.relation.references | W. Zhai, C. Li, Q. Cheng, F. Ding, and Z. Chen, “Exploring Multisource Feature Fusion and Stacking Ensemble Learning for Accurate Estimation of Maize Chlorophyll Content Using Unmanned Aerial Vehicle Remote Sensing,” Remote Sens (Basel), vol. 15, no. 13, Jul. 2023, doi: 10.3390/rs15133454. | spa |
dc.relation.references | A. V. Turukmane, M. Pradeepa, K. S. S. Reddy, R. Suganthi, Y. M. Riyazuddin, and V. V. S. Tallapragada, “Smart farming using cloud-based Iot data analytics,” Measurement: Sensors, vol. 27, p. 100806, Jun. 2023, doi: 10.1016/J.MEASEN.2023.100806. | spa |
dc.relation.references | M. S. Mahmud, L. He, P. Heinemann, D. Choi, and H. Zhu, “Unmanned aerial vehicle based tree canopy characteristics measurement for precision spray applications,” Smart Agricultural Technology, vol. 4, p. 100153, Aug. 2023, doi: 10.1016/J.ATECH.2022.100153. | spa |
dc.relation.references | É. A. S. Moriya, N. N. Imai, A. M. G. Tommaselli, E. Honkavaara, and D. L. Rosalen, “Design of Vegetation Index for Identifying the Mosaic Virus in Sugarcane Plantation: A Brazilian Case Study,” Agronomy, vol. 13, no. 6, Jun. 2023, doi: 10.3390/agronomy13061542. | spa |
dc.relation.references | B. Xu, J. Fan, J. Chao, N. Arsenijevic, R. Werle, and Z. Zhang, “Instance segmentation method for weed detection using UAV imagery in soybean fields,” Comput Electron Agric, vol. 211, p. 107994, Aug. 2023, doi: 10.1016/J.COMPAG.2023.107994. | spa |
dc.relation.references | L. Sánchez-Fernández, M. Barrera, J. Martínez-Guanter, and M. Pérez-Ruiz, “Drift reduction in orchards through the use of an autonomous UAV system,” Comput Electron Agric, vol. 211, p. 107981, Aug. 2023, doi: 10.1016/J.COMPAG.2023.107981. | spa |
dc.relation.references | Y. Wang et al., “Estimating Relative Chlorophyll Content in Rice Leaves Using Unmanned Aerial Vehicle Multi-Spectral Images and Spectral–Textural Analysis,” Agronomy, vol. 13, no. 6, Jun. 2023, doi: 10.3390/agronomy13061541. | spa |
dc.relation.references | C. Lin, C. Guo, W. Du, J. Deng, L. Wang, and G. Wu, “Maximizing Energy Efficiency of Period-Area Coverage with UAVs for Wireless Rechargeable Sensor Networks.” | spa |
dc.relation.references | C. Munghemezulu, Z. Mashaba-Munghemezulu, P. E. Ratshiedana, E. Economon, G. Chirima, and S. Sibanda, “Unmanned Aerial Vehicle (UAV) and Spectral Datasets in South Africa for Precision Agriculture,” Data (Basel), vol. 8, no. 6, Jun. 2023, doi: 10.3390/data8060098. | spa |
dc.relation.references | S. Sapkota and D. R. Paudyal, “Growth Monitoring and Yield Estimation of Maize Plant Using Unmanned Aerial Vehicle (UAV) in a Hilly Region,” Sensors, vol. 23, no. 12, Jun. 2023, doi: 10.3390/s23125432. | spa |
dc.relation.references | H. Pu et al., “Tassel-YOLO: A New High-Precision and Real-Time Method for Maize Tassel Detection and Counting Based on UAV Aerial Images,” Drones, vol. 7, no. 8, Aug. 2023, doi: 10.3390/drones7080492. | spa |
dc.relation.references | W. Zhai et al., “Enhancing Wheat Above-Ground Biomass Estimation Using UAV RGB Images and Machine Learning: Multi-Feature Combinations, Flight Height, and Algorithm Implications,” Remote Sens (Basel), vol. 15, no. 14, Jul. 2023, doi: 10.3390/rs15143653. | spa |
dc.relation.references | I. Blekanov, A. Molin, D. Zhang, E. Mitrofanov, O. Mitrofanova, and Y. Li, “Monitoring of grain crops nitrogen status from uav multispectral images coupled with deep learning approaches,” Comput Electron Agric, vol. 212, p. 108047, Sep. 2023, doi: 10.1016/J.COMPAG.2023.108047. | spa |
dc.relation.references | F. L. Macedo et al., “Estimation of Productivity and Above-Ground Biomass for Corn (Zea mays) via Vegetation Indices in Madeira Island,” Agriculture (Switzerland), vol. 13, no. 6, Jun. 2023, doi: 10.3390/agriculture13061115. | spa |
dc.relation.references | V. Amputu et al., “Unmanned aerial systems accurately map rangeland condition indicators in a dryland savannah,” Ecol Inform, vol. 75, p. 102007, Jul. 2023, doi: 10.1016/J.ECOINF.2023.102007. | spa |
dc.relation.references | C. Yan, Z. Li, Z. Zhang, Y. Sun, Y. Wang, and Q. Xin, “High-resolution mapping of paddy rice fields from unmanned airborne vehicle images using enhancedTransUnet,” Comput Electron Agric, vol. 210, p. 107867, Jul. 2023, doi: 10.1016/J.COMPAG.2023.107867. | spa |
dc.relation.references | B. Xu et al., “Improved weed mapping in corn fields by combining UAV-based spectral, textural, structural, and thermal measurements,” Pest Manag Sci, vol. 79, no. 7, pp. 2591–2602, Jul. 2023, doi: 10.1002/ps.7443. | spa |
dc.relation.references | Z. qi LIAO et al., “A double-layer model for improving the estimation of wheat canopy nitrogen content from unmanned aerial vehicle multispectral imagery,” J Integr Agric, vol. 22, no. 7, pp. 2248–2270, Jul. 2023, doi: 10.1016/J.JIA.2023.02.022. | spa |
dc.relation.references | P. K. S and N. K. K, “Drone-based apple detection: Finding the depth of apples using YOLOv7 architecture with multi-head attention mechanism,” Smart Agricultural Technology, vol. 5, p. 100311, Oct. 2023, doi: 10.1016/J.ATECH.2023.100311. | spa |
dc.relation.references | W. Sun, B. Fu, and Z. Zhang, “Maize Nitrogen Grading Estimation Method Based on UAV Images and an Improved Shufflenet Network,” Agronomy, vol. 13, no. 8, Aug. 2023, doi: 10.3390/agronomy13081974. | spa |
dc.relation.references | S. M. Wang et al., “Pixel-class prediction for nitrogen content of tea plants based on unmanned aerial vehicle images using machine learning and deep learning,” Expert Syst Appl, vol. 227, p. 120351, Oct. 2023, doi: 10.1016/J.ESWA.2023.120351. | spa |
dc.relation.references | X. Quan, Q. Guo, J. Ma, and R. Doluschitz, “The economic effects of unmanned aerial vehicles in pesticide application: evidence from Chinese grain farmers,” Precis Agric, vol. 24, no. 5, pp. 1965–1981, Oct. 2023, doi: 10.1007/s11119-023- 10025-9. | spa |
dc.relation.references | Y. Fan et al., “Using an optimized texture index to monitor the nitrogen content of potato plants over multiple growth stages,” Comput Electron Agric, vol. 212, p. 108147, Sep. 2023, doi: 10.1016/J.COMPAG.2023.108147. | spa |
dc.relation.references | S. Xiao et al., “High-throughput calculation of organ-scale traits with reconstructed accurate 3D canopy structures using a UAV RGB camera with an advanced cross-circling oblique route,” ISPRS Journal of Photogrammetry and Remote Sensing, vol. 201, pp. 104–122, Jul. 2023, doi: 10.1016/J.ISPRSJPRS.2023.05.016. | spa |
dc.relation.references | A. Escolà et al., “Mobile terrestrial laser scanner vs. UAV photogrammetry to estimate woody crop canopy parameters – Part 1: Methodology and comparison in vineyards,” Comput Electron Agric, vol. 212, p. 108109, Sep. 2023, doi: 10.1016/J.COMPAG.2023.108109. | spa |
dc.relation.references | A. Guo et al., “Inversion of maize leaf area index from UAV hyperspectral and multispectral imagery,” Comput Electron Agric, vol. 212, p. 108020, Sep. 2023, doi: 10.1016/J.COMPAG.2023.108020. | spa |
dc.relation.references | H. Puppala, P. R. T. Peddinti, J. P. Tamvada, J. Ahuja, and B. Kim, “Barriers to the adoption of new technologies in rural areas: The case of unmanned aerial vehicles for precision agriculture in India,” Technol Soc, vol. 74, p. 102335, Aug. 2023, doi: 10.1016/J.TECHSOC.2023.102335. | spa |
dc.relation.references | Z. Li, Z. Chen, Q. Cheng, S. Fei, and X. Zhou, “Deep Learning Models Outperform Generalized Machine Learning Models in Predicting Winter Wheat Yield Based on Multispectral Data from Drones,” Drones, vol. 7, no. 8, Aug. 2023, doi: 10.3390/drones7080505 | spa |
dc.relation.references | J. Xiao et al., “Enhancing assessment of corn growth performance using unmanned aerial vehicles (UAVs) and deep learning,” Measurement, vol. 214, p. 112764, Jun. 2023, doi: 10.1016/J.MEASUREMENT.2023.112764. | spa |
dc.relation.references | P. Battistoni et al., “A Cyber-Physical System for Wildfire Detection and Firefighting,” Future Internet, vol. 15, no. 7, Jul. 2023, doi: 10.3390/fi15070237. | spa |
dc.relation.references | M. Mamalis, E. Kalampokis, I. Kalfas, and K. Tarabanis, “Deep Learning for Detecting Verticillium Fungus in Olive Trees: Using YOLO in UAV Imagery,” Algorithms, vol. 16, no. 7, Jul. 2023, doi: 10.3390/a16070343. | spa |
dc.relation.references | X. Bai et al., “RPNet: Rice plant counting after tillering stage based on plant attention and multiple supervision network,” Crop J, vol. 11, no. 5, pp. 1586–1594, Oct. 2023, doi: 10.1016/J.CJ.2023.04.005. | spa |
dc.relation.references | Z. Longfei et al., “Improved Yield Prediction of Ratoon Rice Using Unmanned Aerial Vehicle-Based Multi-Temporal Feature Method,” Rice Sci, vol. 30, no. 3, pp. 247–256, May 2023, doi: 10.1016/J.RSCI.2023.03.008. | spa |
dc.relation.references | M. S. Mia et al., “Multimodal Deep Learning for Rice Yield Prediction Using UAVBased Multispectral Imagery and Weather Data,” Remote Sens (Basel), vol. 15, no. 10, May 2023, doi: 10.3390/rs15102511. | spa |
dc.relation.references | A. Tschand, “Semi-supervised machine learning analysis of crop color for autonomous irrigation,” Smart Agricultural Technology, vol. 3, p. 100116, Feb. 2023, doi: 10.1016/J.ATECH.2022.100116. | spa |
dc.relation.references | E. K. Raptis et al., “End-to-end Precision Agriculture UAV-Based Functionalities Tailored to Field Characteristics,” Journal of Intelligent and Robotic Systems: Theory and Applications, vol. 107, no. 2, Feb. 2023, doi: 10.1007/s10846-022- 01761-7. | spa |
dc.relation.references | A. Khanna and S. Kaur, “An empirical analysis on adoption of precision agricultural techniques among farmers of Punjab for efficient land administration,” Land use policy, vol. 126, p. 106533, Mar. 2023, doi: 10.1016/J.LANDUSEPOL.2022.106533. | spa |
dc.relation.references | C. McCarthy et al., “Can Drones Help Smallholder Farmers Improve Agriculture Efficiencies and Reduce Food Insecurity in Sub-Saharan Africa? Local Perceptions from Malawi,” Agriculture (Switzerland), vol. 13, no. 5, May 2023, doi: 10.3390/agriculture13051075. | spa |
dc.relation.references | A. Maulit, A. Nugumanova, K. Apayev, Y. Baiburin, and M. Sutula, “A Multispectral UAV Imagery Dataset of Wheat, Soybean and Barley Crops in East Kazakhstan,” Data (Basel), vol. 8, no. 5, May 2023, doi: 10.3390/data8050088. | spa |
dc.relation.references | A. Gkillas, D. Kosmopoulos, and K. Berberidis, “Cost-efficient coupled learning methods for recovering near-infrared information from RGB signals: Application in precision agriculture,” Comput Electron Agric, vol. 209, p. 107833, Jun. 2023, doi: 10.1016/J.COMPAG.2023.107833. | spa |
dc.relation.references | E. C. Nnadozie, O. N. Iloanusi, O. A. Ani, and K. Yu, “Detecting Cassava Plants under Different Field Conditions Using UAV-Based RGB Images and Deep Learning Models,” Remote Sens (Basel), vol. 15, no. 9, May 2023, doi: 10.3390/rs15092322. | spa |
dc.relation.references | K. Jindo et al., “Unmanned Aerial Vehicle (UAV) for Detection and Prediction of Damage Caused by Potato Cyst Nematode G. pallida on Selected Potato Cultivars,” Remote Sens (Basel), vol. 15, no. 5, Mar. 2023, doi: 10.3390/rs15051429. | spa |
dc.relation.references | L. Holtorf, I. Titov, F. Daschner, and M. Gerken, “UAV-Based Wireless Data Collection from Underground Sensor Nodes for Precision Agriculture,” AgriEngineering, vol. 5, no. 1, pp. 338–354, Mar. 2023, doi: 10.3390/agriengineering5010022. | spa |
dc.relation.references | Z. Li, X. Zhou, Q. Cheng, S. Fei, and Z. Chen, “A Machine-Learning Model Based on the Fusion of Spectral and Textural Features from UAV Multi-Sensors to Analyse the Total Nitrogen Content in Winter Wheat,” Remote Sens (Basel), vol. 15, no. 8, Apr. 2023, doi: 10.3390/rs15082152. | spa |
dc.rights | Atribución-NoComercial-SinDerivadas 2.5 Colombia | * |
dc.rights.accessrights | info:eu-repo/semantics/openAccess | |
dc.rights.coar | http://purl.org/coar/access_right/c_abf2 | spa |
dc.rights.local | Abierto (Texto Completo) | spa |
dc.rights.uri | http://creativecommons.org/licenses/by-nc-nd/2.5/co/ | * |
dc.subject.keyword | Drones | spa |
dc.subject.keyword | Agriculture | spa |
dc.subject.keyword | Precision Agriculture | spa |
dc.subject.keyword | Unmanned Aerial Vehicles | spa |
dc.subject.keyword | Artificial Intelligence | spa |
dc.subject.keyword | Big Data | spa |
dc.subject.keyword | Machine Learning | spa |
dc.subject.keyword | Deep Learning | spa |
dc.subject.proposal | Drones | spa |
dc.subject.proposal | Agricultura | spa |
dc.subject.proposal | Agricultura de Precisión | spa |
dc.subject.proposal | UAV | spa |
dc.subject.proposal | Vehiculos Aereos no Tripulados | spa |
dc.subject.proposal | Inteligencia Artificial | spa |
dc.subject.proposal | Big Data | spa |
dc.subject.proposal | Machine Learning | spa |
dc.subject.proposal | Deep Learning | spa |
dc.title | Uso de Drones en la Agricultura de Precisión | spa |
dc.type.coar | http://purl.org/coar/resource_type/c_7a1f | |
dc.type.coarversion | http://purl.org/coar/version/c_ab4af688f83e57aa | |
dc.type.drive | info:eu-repo/semantics/bachelorThesis | |
dc.type.local | Trabajo de grado | spa |
dc.type.version | info:eu-repo/semantics/acceptedVersion |
Archivos
Bloque original
1 - 3 de 3
Cargando...
- Nombre:
- 2024williamotalora
- Tamaño:
- 540.63 KB
- Formato:
- Adobe Portable Document Format
- Descripción:
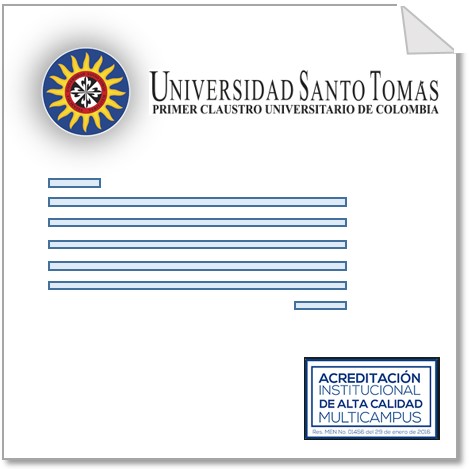
- Nombre:
- 2024cartaderechosdeautor
- Tamaño:
- 466.32 KB
- Formato:
- Adobe Portable Document Format
- Descripción:
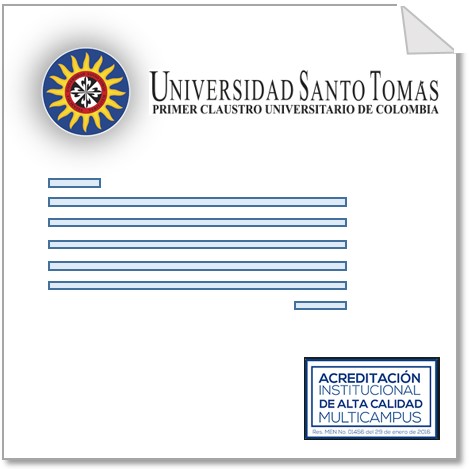
- Nombre:
- 2024cartaaprobaciónfacultad
- Tamaño:
- 280.62 KB
- Formato:
- Adobe Portable Document Format
- Descripción:
Bloque de licencias
1 - 1 de 1
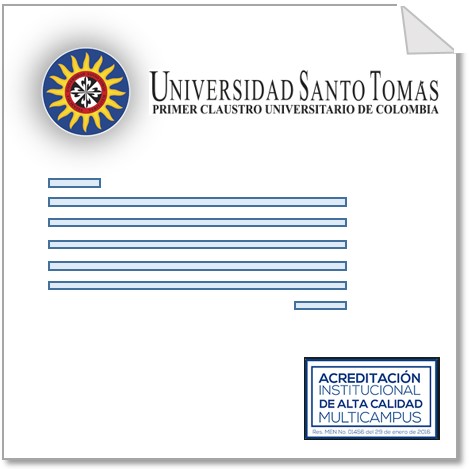
- Nombre:
- license.txt
- Tamaño:
- 807 B
- Formato:
- Item-specific license agreed upon to submission
- Descripción: