Diseño de un controlador aplicado a un péndulo invertido utilizando estrategias basadas en aprendizaje de máquina
dc.contributor.advisor | Guarnizo Marín, José Guillermo | |
dc.contributor.advisor | Camacho Poveda, Edgar Camilo | |
dc.contributor.author | Rincón Martínez, Julián | |
dc.contributor.author | Pineda Gonzalez, Gustavo Alonso | |
dc.contributor.corporatename | Universidad Santo Tomás | spa |
dc.contributor.cvlac | https://scienti.minciencias.gov.co/cvlac/visualizador/generarCurriculoCv.do?cod_rh=0001630084&lang=es | spa |
dc.contributor.cvlac | https://scienti.minciencias.gov.co/cvlac/visualizador/generarCurriculoCv.do?cod_rh=0000855847&lang=es | spa |
dc.contributor.googlescholar | https://scholar.google.com/citations?hl=es&user=3JSJ0C4AAAAJ | spa |
dc.contributor.googlescholar | https://scholar.google.com/citations?hl=es&user=tJG988kAAAAJ | spa |
dc.contributor.orcid | https://orcid.org/0000-0002-8401-4949 | spa |
dc.contributor.orcid | https://orcid.org/0000-0002-6084-2512 | spa |
dc.coverage.campus | CRAI-USTA Bogotá | spa |
dc.date.accessioned | 2022-01-28T20:11:28Z | |
dc.date.available | 2022-01-28T20:11:28Z | |
dc.date.issued | 2022-01-24 | |
dc.description | Este documento presenta el desarrollo de controlador aplicado a un péndulo invertido simple, utilizando estrategias basadas en aprendizaje de máquina. Para el desarrollo de este proyecto, se hace uso de una plataforma simulada en el software Simulink, la cual realiza una respectiva caracterización del sistema y una representación de la planta a partir de diagrama de bloques implementado en este Software. En este documento se muestran las ecuaciones no lineales de la planta para que, a partir del comportamiento de esta, se diseñe un control basado en redes neuronales que sea capaz de estabilizar la posición angular del péndulo invertido alrededor de un punto de trabajo específico. El desarrollo del proyecto se divide en 4 fases importantes. La primera fase consiste en conocer las variables de entrada y salida de la planta que se usarán para el diseño de la red neuronal. La variable de entrada hace referencia al voltaje inyectado a la planta, mientras que las variables de salida hacen referencia a los ángulos del péndulo y del brazo giratorio. También se muestran las ecuaciones que describen el comportamiento del sistema de péndulo invertido. En la segunda fase se hace una revisión en el estado del arte, esto con el fin de observar metodologías implementadas en trabajos anteriores, tomando como partida algunos métodos de aprendizaje de máquina que puedan ser usados para el control de posición angular en un péndulo. La tercera fase consiste en la obtención de los datos de la planta que serán los usados en el diseño de las redes neuronales como conjunto de datos de entrenamiento y pruebas. A partir de los datos obtenidos en la tercera fase, en la cuarta fase se implementa la red neuronal con su respectivo entrenamiento y será la encargada de estabilizar el péndulo. Se presentan los resultados experimentales que se llevaron a cabo sobre las redes neuronales implementadas, teniendo en cuenta diferentes pruebas realizadas, haciendo cambios en parámetros como los retardos, los ciclos, la frecuencia de muestreo o los datos de entrada. También se hace un análisis de estas pruebas y de las gráficas obtenidas del sistema a partir de parámetro de error, con el fin observar el comportamiento del péndulo una vez la red neuronal ha sido entrenada. | spa |
dc.description.abstract | This document presents the development of a controller applied to a simple inverted pendulum, using strategies based on machine learning. For the development of this project, a simulated platform is used in the Simulink software, which performs a respective characterization of the system and a representation of the plant from a block diagram implemented in this Software. In this document, the non-linear equations of the plant are shown so that, based on its behavior, a control based on neural networks is designed that is capable of stabilizing the angular position of the inverted pendulum around a specific work point. The development of the project is divided into 4 important phases. The first phase consists of knowing the input and output variables of the plant that will be used for the design of the neural network. The input variable refers to the voltage injected into the plant, while the output variables refer to the angles of the pendulum and the rotating arm. The equations that describe the behavior of the inverted pendulum system are also shown. In the second phase, a review of the state of the art is made, this in order to observe methodologies implemented in previous works, taking as a starting point some machine learning methods that can be used for angular position control in a pendulum. The third phase consists of obtaining the plant data that will be used in the design of the neural networks as a set of training and testing data. From the data obtained in the third phase, in the fourth phase the neural network is implemented with its respective training and it will be in charge of stabilizing the pendulum. The experimental results that were carried out on the implemented neural networks are presented, taking into account different tests carried out, making changes in parameters such as delays, cycles, sampling frequency or input data. An analysis of these tests and of the graphs obtained from the system from the error parameter is also made, in order to observe the behavior of the pendulum once the neural network has been trained. | spa |
dc.description.degreelevel | Pregrado | spa |
dc.description.degreename | Ingeniero Electronico | spa |
dc.format.mimetype | application/pdf | spa |
dc.identifier.citation | Rincón Martínez, J. y Pineda Gonzalez, G. A. (2022). Diseño de un Controlador Aplicado a un Péndulo Invertido Utilizando Estrategias Basadas en Aprendizaje de Máquina [Tesis de pregrado, Universidad Santo Tomás] Repositorio institucional | spa |
dc.identifier.instname | instname:Universidad Santo Tomás | spa |
dc.identifier.reponame | reponame:Repositorio Institucional Universidad Santo Tomás | spa |
dc.identifier.repourl | repourl:https://repository.usta.edu.co | spa |
dc.identifier.uri | http://hdl.handle.net/11634/42773 | |
dc.language.iso | spa | spa |
dc.publisher | Universidad Santo Tomás | spa |
dc.publisher.faculty | Facultad de Ingeniería Electrónica | spa |
dc.publisher.program | Pregrado Ingeniería Electrónica | spa |
dc.relation.references | Ethem Alpaydin. Introduction to Machine Learning. 4.a ed. MIT Press Academic, 2020. | spa |
dc.relation.references | María M. Seron. «Sistemas No Lineales». En: Universidad Nacional de Rosario (2000), págs. 7-8. | spa |
dc.relation.references | M. J. G. Guarnizo, R. C. L. Trujillo y M. J. A. Guacaneme. «Modeling and control of a two DOF helicopter using a robust control design based on DK iteration». En: IECON 2010 - 36th Annual Conference on IEEE Industrial Electronics Society. 2010, págs. 162-167. | spa |
dc.relation.references | Gerson. Figueroa. «Análisis de un péndulo invertido mediante técnicas de control inteligente ». En: Instituo Politécnico Nacional (2013), págs. 1-172. | spa |
dc.relation.references | Geun Hyeong y Seul Jung. «Control of inverted pendulum system using a neuro-fuzzy controller for intelligent control education». En: 2008 IEEE International Conference on Mechatronics and Automation. IEEE, 2008, págs. 965-970. | spa |
dc.relation.references | Alexander Winkler y Jozef Suchý. «Identification and controller design for the inverted pendulum actuated by a position controlled robot». En: 18th International Conference on Methods Models in Automation Robotics (MMAR)". IEEE, 2013, págs. 285-263. | spa |
dc.relation.references | Richard F Chidzonga y Fredson. A. Phiri. «Stabilizing an inverted pendulum on an airbed track». En: AFRICON Conference 2007. IEEE, 2007, págs. 1-7. | spa |
dc.relation.references | Javier Aracil y Francisco Gordillo. «The inverted pendulum: a benchmark in nonlinear control». En: Proceedings World Automation Congress, 2004. IEEE, 2004, págs. 468-482. | spa |
dc.relation.references | Nenad Muskinja y Boris Tovornik. «Swinging up and stabilization of a real inverted pendulum ». En: IEEE Transactions on Industrial Electronics 53.2 (2006), págs. 631-639. | spa |
dc.relation.references | Warren N. White y col. «Design, build, and test of an autonomous inverted pendulum cart». En: 2013 American Control Conference. IEEE, 2013, págs. 5893-5898. | spa |
dc.relation.references | Khizir Mahmud. «Design and analysis of the control of an inverted pendulum system by Matlab». En: 2013 IEEE Global High Tech Congress on Electronics. IEEE, 2013, págs. 207-211. | spa |
dc.relation.references | Samatthachai Panya y col. «Hybrid Controller for Inverted Pendulum System». En: 2008 International Symposium on Communications and Information Technologies (ISCIT). IEEE, 2008, págs. 385-388. | spa |
dc.relation.references | Ju-Bong Kim y col. «Imitation Reinforcement Learning-Based Remote Rotary Inverted Pendulum Control in OpenFlow Network». En: IEEE Access 7 (2019), págs. 36682-36690. | spa |
dc.relation.references | Takanori Shibata y col. «Skill based control by using fuzzy neural network for hierarchical intelligent control». En: 1992 IJCNN International Joint Conference on Neural Networks. IEEE, 1992, págs. 81-86. | spa |
dc.relation.references | Bavarian B. «Introduction to neural networks for intelligent control». En: IEEE Control Systems Magazine 8.2 (1988), págs. 3-7. | spa |
dc.relation.references | Kumpati S Narendra y Snehasis Mukhopadhyay. «Intelligent control using neural networks ». En: IEEE Control Systems Magazine 12.2 (1992), págs. 11-18. | spa |
dc.relation.references | Chrysostomos Stylios y Peter Groumpos. «Fuzzy cognitive maps: a soft computing technique for intelligent control». En: 2000 IEEE International Symposium on Intelligent Control. Held jointly with the 8th IEEE Mediterranean Conference on Control and Automation (Cat. No.00CH37147). IEEE, 2000, págs. 97-102. | spa |
dc.relation.references | Jyoti Krishen y Victor M Becerra. «Efficient fuzzy control of a rotary inverted pendulum based on LQR mapping». En: 2006 IEEE Conference on Computer Aided Control System Design, 2006 IEEE International Conference on Control Applications, 2006 IEEE International Symposium on Intelligent Control. IEEE, 2006, págs. 2701-2706. | spa |
dc.relation.references | Ammar Al-Jodah, Hassan Zargarzadeh y Maythem K Abbas. «Experimental verification and comparison of different stabilizing controllers for a rotary inverted pendulum». En: 2013 IEEE International Conference on Control System, Computing and Engineering. IEEE, 2013, págs. 417-423. | spa |
dc.relation.references | Monica Roman, Eugen Bobasu y Dorin Sendrescu. «Modelling of the rotary inverted pendulum system». En: 2008 IEEE International Conference on Automation, Quality and Testing, Robotics. IEEE, 2008, págs. 141-146. | spa |
dc.relation.references | P. Karam J. Apkarian y M. Lévis. «Rotary Inverted Pendulum Experiment for MATLAB R /Simulink R Users». En: Quanser Inc. (2012), págs. 1-6. | spa |
dc.relation.references | Tianxiao Liu. «U.S. Pandemic Prediction Using Regression and Neural Network Models». En: 2020 International Conference on Intelligent Computing and Human-Computer Interaction (ICHCI). IEEE, 2020, págs. 351-354. | spa |
dc.relation.references | Tushar Verma y col. «Data Analysis to Generate Models Based on Neural Network and Regression for Solar Power Generation Forecasting». En: 2016 7th International Conference on Intelligent Systems, Modelling and Simulation (ISMS). IEEE, 2016, págs. 97-100. | spa |
dc.relation.references | Aakanksha Sharaff y Samuel Robin Roy. «Comparative Analysis of Temperature Prediction Using Regression Methods and Back Propagation Neural Network». En: 2018 2nd International Conference on Trends in Electronics and Informatics (ICOEI). IEEE, 2018, págs. 739-742. | spa |
dc.relation.references | R. C. L. Trujillo M. J. G. Guarnizo y M. J. A. Guacaneme. «General Inverse Neural Current Control for Buck Converter». En: Novel Algorithms and Techniques In Telecommunications, Automation and Industrial Electronics. Springer, Dordrech (2008), págs. 117-122. | spa |
dc.relation.references | Razeef Mohd, Muheet Ahmed Butt y Majid Zaman Baba. «SALM-NARX: Self Adaptive LM- based NARX model for the prediction of rainfall». En: 2018 2nd International Conference on I-SMAC (IoT in Social, Mobile, Analytics and Cloud) (I-SMAC)I-SMAC (IoT in Social, Mobile, Analytics and Cloud) (I-SMAC), 2018 2nd International Conference on. IEEE, 2018, págs. 580-585. DOI: 10.1109/I-SMAC.2018.8653747. | spa |
dc.relation.references | Osorio Zúñiga Carlos Andrés. «DISEÑO, CONSTRUCCION Y CONTROL DE UN PENDULO INVERTIDO ROTACIONAL UTILIZANDO TECNICAS LINEALES Y NO LINEALES ». En: Universidad Nacional de Colombia (2009), págs. 13-14. | spa |
dc.relation.references | Hill Rick. «Inverted Pendulum Control». En: MATLAB Central File Exchange (2013). | spa |
dc.relation.references | Atria Innovation. Qué son las redes neuronales y sus funciones. Oct. de 2019. URL: https: //www.atriainnovation.com/que-son-las-redes-neuronales-y-susfunciones/#:~: text=Las-redes-neuronales-artificiales-son,entrada20hastagenerar- una-salida. | spa |
dc.relation.references | C. Luis y M. Diego. «Redes Neuronales». En: Cinvestav (2020). | spa |
dc.relation.references | Interactive Chaos. Backpropagation | Interactive Chaos. URL: https://interactivechaos. com/es/manual/tutorial-de-machine-learning/backpropagation. | spa |
dc.relation.references | Isis Bonet y col. «Redes neuronales recurrentes para el análisis de secuencias». En: 2007 Revista Cubana de Ciencias Informáticas 1.2 (2007), págs. 48-57. | spa |
dc.relation.references | Jordi Torres. Redes Neuronales Recurrentes. Mar. de 2021. URL: https://torres.ai/ redes-neuronales-recurrentes/. | spa |
dc.relation.references | Galán Pablo. «INCORPORACIÓN DE INTELIGENCIA ARTIFICIAL EN GEMELO VIRTUAL BÁSICO DE ROBOT INDUSTRIAL». En: UNIVERSIDAD DE CANTABRIA (2019). | spa |
dc.relation.references | Calvo Diego. «Red Neuronal Recurrente – RNN». En: Diego Calvo (2018). | spa |
dc.relation.references | R. Aguilar y A. Martín. «RED NEURONAL AUTORREGRESIVA NO LINEAL CON ENTRADAS EXÓGENAS PARA LA PREDICCIÓN DEL ELECTROENCEFALOGRAMAFETAL ». En: Department of Computer and Systems Engineering, University of La Laguna (2017), pág. 2. | spa |
dc.relation.references | Victor Rodriguez, Jaime Garzón y Jesús López. «Control Neuronal por Modelo Inverso de un Servosistema Usando Algoritmos de Aprendizaje Levenberg-Marquardt y Bayesiano ». En: VIII Congreso de la Asociación Colombiana de Automática. Universidad Tecnológica de Bolívar, 2009, págs. 1-6. | spa |
dc.relation.references | Jaime Durán. Todo lo que Necesitas Saber sobre el Descenso del Gradiente Aplicado a Redes Neuronales. Sep. de 2019. URL: https : / / medium . com / metadatos / todo - lo - que- necesitas- saber- sobre- el- descenso- del- gradiente- aplicadoa- redes-neuronales-19bdbb706a78. | spa |
dc.relation.references | Sergio Ledesma. «Las Redes Neuronales implementación y consideraciones prácticas». En: Fifth Mexican International Conference on Artificial Intelligence. Universidad de Guanajuato, 2016, págs. 1-50. | spa |
dc.relation.references | Jose Martinez Heras. Error Cuadrático Medio para Regresión. Oct. de 2020. URL: https: //www.iartificial.net/error-cuadratico-medio-para-regresion/#: ~:text=El-Error-Cuadr%C3%A1tico-Medio-es,podremos-indicar-elresultado- correcto. | spa |
dc.relation.references | IBM. Acerca de la regresión lineal. 2020. URL: https://www.ibm.com/co-es/analytics/ learn/linear-regression#:~:text=%C2%BFQu%C3%A9-es-la-regresi%C3% B3n-lineal,variable-se-denomina-variable-independiente. | spa |
dc.relation.references | Luis Garrido. «Identificación, estimación y control de sistemas no-lineales mediante RGO». En: Universidad Carlos III de Madrid (1999). | spa |
dc.relation.references | National Instruments. Adquirir una Señal Analógica: Ancho de Banda, Teorema de Muestreo de Nyquist y Aliasing. 2019. URL: https://www.ni.com/es- co/innovations/ white-papers/06/acquiring-an-analog-signal--bandwidth--nyquistsampling- theorem-.html. | spa |
dc.relation.references | U. Francisco. «Estrategia de identificación dinámica no lineal basada en NARX para fuentes de generación distribuida acompladas electrónicamente a Micro-Redes AC/DC». En: Universidad Politécnica Salesina - SEDE QUITO (2020), pág. 34. | spa |
dc.relation.references | M. Moumouni Hamidou y N. Talibi Soumaïla. «Electrical Charge of Niamey City Modelisation by Neural Network». En: Sci. J. Energy Eng., vol. 7 (2019), pág. 13. | spa |
dc.relation.references | Zina Boussaada y col. «A nonlinear autoregressive exogenous (NARX) neural network model for the prediction of the daily direct solar radiation». En: The 10th International Conference on Sustainable Energy and Environmental Protection. Vol. 11. 3. IEEE, 2018, pág. 620. | spa |
dc.relation.references | Luis Gonzaga Baca Ruiz y col. «An Application of Non-Linear Autoregressive Neural Networks to Predict Energy Consumption in Public Buildings». En: Energies 9.9 (2016). | spa |
dc.relation.references | Casimiro Rocha y Josér Escorcia. «Sistema de Visión Artificial para la Detección y el Reconocimiento de Señales de Tráfico Basado en Redes Neuronales». En: 2010 LACCEI Latin American and Caribbean Conference for Engineering and Technology. 2010. | spa |
dc.relation.references | Llano. L y Hoyos. A. «Comparación del Desempeño de Funciones de Activación en Redes Feedforward para aproximar Funciones de Datos con y sin Ruido.» En: Universidad Nacional de Colombia (2007). | spa |
dc.relation.references | I. Maldonado y A. Zabidi. «Estimación de los parámetros de un modelo haciendo uso de correspondencias con incertidumbre». En: Centro de Investigacion en Matematicas, A.C - CIMAT (2011), págs. 20-25. | spa |
dc.relation.references | Nadiah Mohamad y col. «Comparison between Levenberg-Marquardt and Scaled Conjugate Gradient training algorithms for Breast Cancer Diagnosis using MLP». En: 6th International Colloquium on Signal Processing its Applications. IEEE, 2010, págs. 1-7. | spa |
dc.relation.references | J. Maria y R. Diego. «Desarrollo y evaluación de un control Neuro-Difuso tipo ANFIS frente a un control PID convencional aplicado al péndulo invertido». En: Universidad Politécnica Salesiana (2016). | spa |
dc.relation.references | k. Maher. «Estudio comparativo entre el control PID Clásico y el Control PID Fraccionario alpicado al sistema del péndulo invertido». En: Universidad Técnológica de Pereria (2020), págs. 98-106. | spa |
dc.relation.references | Khushboo Barya, Sheela Tiwari y Rameshwar Jha. «Comparison of LQR and robust controllers for stabilizing inverted pendulum system». En: 2010 INTERNATIONAL CONFERENCE ON COMMUNICATION CONTROL AND COMPUTING TECHNOLOGIES. IEEE, 2010, págs. 300-304. | spa |
dc.relation.references | Faiber Robayo, Ana Barrera y Laura Polanco. «Desarrollo de un controlador basado en redes neuronales para un sistema multivariable de nivel y caudal». En: Revista Ingeniería y Región 14.2 (2015), págs. 43-54. | spa |
dc.rights | Atribución-NoComercial-SinDerivadas 2.5 Colombia | * |
dc.rights.accessrights | info:eu-repo/semantics/openAccess | |
dc.rights.coar | http://purl.org/coar/access_right/c_abf2 | |
dc.rights.local | Abierto (Texto Completo) | spa |
dc.rights.uri | http://creativecommons.org/licenses/by-nc-nd/2.5/co/ | * |
dc.subject.keyword | Controller | spa |
dc.subject.keyword | Levenberg-Marquardt | spa |
dc.subject.keyword | Machine Learning | spa |
dc.subject.keyword | No lineal System | spa |
dc.subject.keyword | NARX | spa |
dc.subject.keyword | Neuronal Network | spa |
dc.subject.keyword | Simple Inverted Pendulum | spa |
dc.subject.keyword | Simulink | spa |
dc.subject.lemb | Aprendizaje-- Clasificación-- Colombia | spa |
dc.subject.lemb | Maquinas-- Ingeniería Electrónica-- Colombia | spa |
dc.subject.lemb | Red Neuronal-- Ingeniería Electrónica | spa |
dc.subject.proposal | Controlador | spa |
dc.subject.proposal | Aprendizaje de máquina | spa |
dc.subject.proposal | Levenberg-Marquardt | spa |
dc.subject.proposal | NARX | spa |
dc.subject.proposal | Péndulo Invertido Simple | spa |
dc.subject.proposal | Sistema no lineal | spa |
dc.subject.proposal | Simulink | spa |
dc.subject.proposal | Red Neuronal | spa |
dc.title | Diseño de un controlador aplicado a un péndulo invertido utilizando estrategias basadas en aprendizaje de máquina | spa |
dc.type | bachelor thesis | |
dc.type.category | Formación de Recurso Humano para la Ctel: Trabajo de grado de Pregrado | spa |
dc.type.coar | http://purl.org/coar/resource_type/c_7a1f | |
dc.type.coarversion | http://purl.org/coar/version/c_ab4af688f83e57aa | |
dc.type.drive | info:eu-repo/semantics/bachelorThesis | |
dc.type.local | Tesis de pregrado | spa |
dc.type.version | info:eu-repo/semantics/acceptedVersion |
Archivos
Bloque original
1 - 3 de 3
Cargando...
- Nombre:
- 2022pinedagustavo.pdf
- Tamaño:
- 2.87 MB
- Formato:
- Adobe Portable Document Format
- Descripción:
- Trabajo de grado
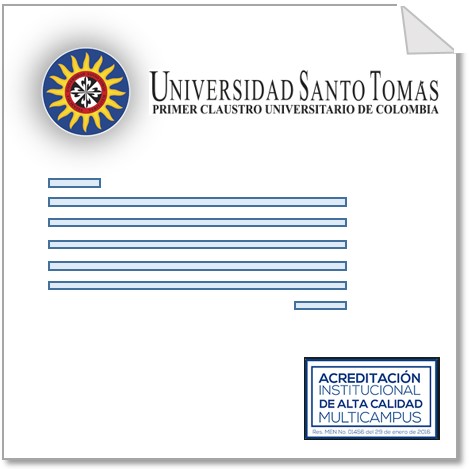
- Nombre:
- 2022pinedagustavo1.pdf
- Tamaño:
- 21.92 KB
- Formato:
- Adobe Portable Document Format
- Descripción:
- Carta de Aprobación
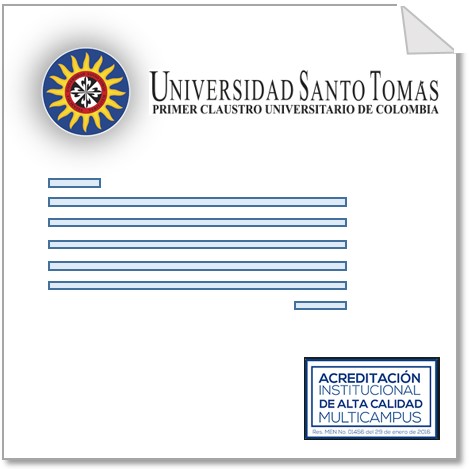
- Nombre:
- 2022pinedagustavo2.pdf
- Tamaño:
- 1.05 MB
- Formato:
- Adobe Portable Document Format
- Descripción:
- Acuerdo de Publicación
Bloque de licencias
1 - 1 de 1
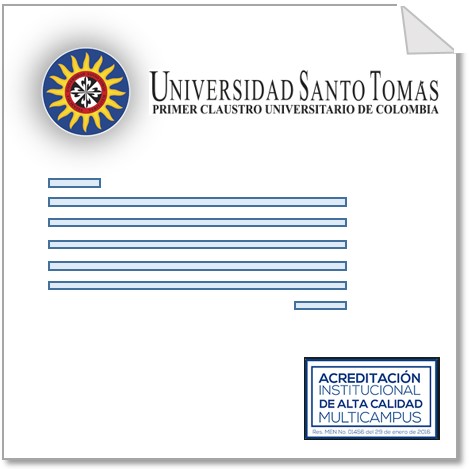
- Nombre:
- license.txt
- Tamaño:
- 807 B
- Formato:
- Item-specific license agreed upon to submission
- Descripción: