The use of working variables in the bayesian modeling of mean and dispersion parameters in generalized nonlinear models with random effects
dc.contributor.author | Gutiérrez, Andrés | spa |
dc.coverage.campus | CRAI-USTA Bogotá | spa |
dc.date.accessioned | 2019-12-17T16:57:01Z | spa |
dc.date.available | 2019-12-17T16:57:01Z | spa |
dc.date.issued | 2014-07-28 | spa |
dc.description.abstract | This article is aimed at reviewing a novel Bayesian approach to handle inference and estimation in the class of generalized nonlinear models. These models include some of the main techniques of statistical methodology, namely generalized linear models and parametric nonlinear regression. In addition, this proposal extends to methods for the systematic treatment of variation that is not explicitly predictedwithin themodel, through the inclusion of random effects, and takes into account the modeling of dispersion parameters in the class of two-parameter exponential family. The methodology is based on the implementation of a two-stage algorithm that induces a hybrid approach based on numerical methods for approximating the likelihood to a normal density using a Taylor linearization around the values of current parameters in an MCMC routine. | spa |
dc.description.domain | http://unidadinvestigacion.usta.edu.co | spa |
dc.format.mimetype | application/pdf | spa |
dc.identifier.doi | https://doi.org/10.1080/03610918.2013.770529 | spa |
dc.identifier.uri | http://hdl.handle.net/11634/20436 | |
dc.relation.references | Aitkin, M. (1987). Modelling variance heterogeneity in normal regression using GLIM. Applied Statistics 36:332–339. | spa |
dc.relation.references | Barndorf-Nielsen, O. E. (1978). Information and Exponential Families in Statistical Theory. New York: Wiley. | spa |
dc.relation.references | Cepeda, E., Achcar, J. A. (2010). Heteroscedastic nonlinear regression models. Comunnications in Statistics Simulation and Computation 39:405–419. | spa |
dc.relation.references | Dellaportas, P., Smith, A. F. M. (1993). Bayesian inference for generalized linear and proportional hazards model via Gibbs sampling. Applied Statistics 42:443–460. | spa |
dc.relation.references | Gammerman, D. (1997). Efficient sampling from the posterior distributions in generalized linear mixed models. Statistics and Computing 7:57–68. | spa |
dc.relation.references | Hastie, T., Tibshirani, R., Friedman, J. (2001). The Elements of Statistical Learning. New York: Springer. | spa |
dc.relation.references | Ibrahim, J. G., Laud, P.W. (1991). On Bayesian analysis of generalized linear models using Jeffreys’s prior. Journal of the American Statistical Association 86:981–986. | spa |
dc.relation.references | Jorgersen, B. (1987). Exponential dispersion models (with discussion). Journal of the Royal Statistical Association B 49:150. | spa |
dc.relation.references | Laird, N. M.,Ware, J. H. (1982).Random effects models for longitudinal data. Biometrics 38:963–974. | spa |
dc.relation.references | McCullagh, P., Nelder, J. A. (1996). Generalized Linear Models. Boca Raton, FL: Chapman and Hall. | spa |
dc.relation.references | Neykov, N. M., Filzmoser, P., Neytchev, P. N. (2012). Robust joint modeling of mean and dispersion through trimming. Computational Statistics and Data Analysis 56(1): 34–48. | spa |
dc.relation.references | Pfefferman, D. (2002). Small area estimation: New developments and directions. International Statistic Review 70:125–143. | spa |
dc.relation.references | R Development Core Team (2009). R: A Language and Environment for Statistical Computing, R Foundation for Statistical Computing, Vienna, Austria. ISBN 3-900051-07-0. | spa |
dc.relation.references | Smyth, G. K. (2002). An efficient algorithm for REML in heteroscedastic regression. Journal of Graphical and Computational Statistics 11:836–847. | spa |
dc.relation.references | Verbeke, G., Molenberghs, G. (2000). Linear Models for Longitudinal Data. New York: Springer- Verlag. | spa |
dc.relation.references | West, M. (1985). Generalized Linear Models: Outlier Acommodation, Scale Parameters and Prior distributions (with discussion). In: Bayesian Statistics 2, Eds., Bernardo, J. M., De Groot,M. H., Lindley, D. V., and Smith, A. F. M. Oxford: Oxford University Press. pp. 461–484. | spa |
dc.relation.references | Zhao, Y., Staudenmayer, J., Coull, B. A., Wand, M. P. (2006). General design Bayesian generalized linear mixed models. Statistical Science 21:35–51. | spa |
dc.rights | Atribución-NoComercial-CompartirIgual 2.5 Colombia | * |
dc.rights.uri | http://creativecommons.org/licenses/by-nc-sa/2.5/co/ | * |
dc.subject.keyword | Bayesian analysis | spa |
dc.subject.keyword | Generalized linear models | spa |
dc.subject.keyword | Heteroscedasticity | spa |
dc.subject.keyword | MCMC | spa |
dc.subject.keyword | Mixed effects models | spa |
dc.subject.keyword | Nonlinear regression | spa |
dc.title | The use of working variables in the bayesian modeling of mean and dispersion parameters in generalized nonlinear models with random effects | spa |
dc.type.category | Generación de Nuevo Conocimiento: Artículos publicados en revistas especializadas - Electrónicos | spa |
Archivos
Bloque original
1 - 1 de 1
Cargando...
- Nombre:
- The use of working variables in the bayesian modeling of mean and dispersion parameters in generalized nonlinear models with random effects.pdf
- Tamaño:
- 246.17 KB
- Formato:
- Adobe Portable Document Format
- Descripción:
- Artículo SCOPUS
Bloque de licencias
1 - 1 de 1
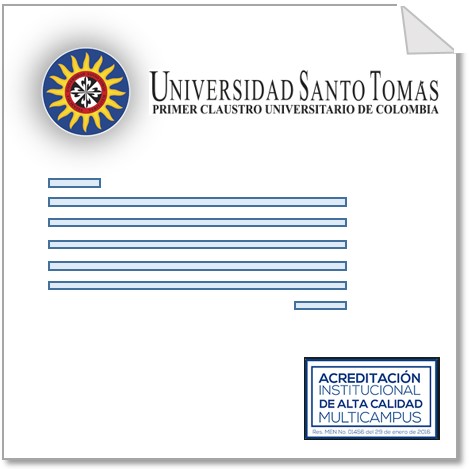
- Nombre:
- license.txt
- Tamaño:
- 807 B
- Formato:
- Item-specific license agreed upon to submission
- Descripción: