Desarrollo de un Algoritmo de Navegación Autónoma Basado en Técnicas de Aprendizaje por Refuerzo Usando Información Visual
dc.contributor.advisor | Calderón Chávez, Juan Manuel | |
dc.contributor.author | Aponte Vargas, Daniel Felipe | |
dc.contributor.author | Martínez Méndez, Erika Dayanna | |
dc.contributor.corporatename | Universidad Santo Tomás | spa |
dc.contributor.cvlac | https://scienti.minciencias.gov.co/cvlac/visualizador/generarCurriculoCv.do?cod_rh=0000380938 | spa |
dc.contributor.cvlac | https://scienti.minciencias.gov.co/cvlac/visualizador/generarCurriculoCv.do?cod_rh=0001723305 | spa |
dc.contributor.orcid | https://orcid.org/0000-0002-4471-3980 | spa |
dc.coverage.campus | CRAI-USTA Bogotá | spa |
dc.date.accessioned | 2023-02-02T17:22:29Z | |
dc.date.available | 2023-02-02T17:22:29Z | |
dc.date.issued | 2023-01-31 | |
dc.description | En este proyecto se realiza la implementación de un algoritmo de navegación autónoma basado en información visual, usando aprendizaje profundo por refuerzo (DRL, por sus siglas en inglés Deep Reinforcement Learning). El algoritmo le enseña a un agente a identificar patrones visuales para navegar hacia un objetivo en un entorno cerrado y desconocido. El proceso de aprendizaje se compone de tres etapas: clasificación, imitación y entrenamiento, y un sistema de Replay Memory. Las etapas de aprendizaje brindan al agente diferentes herramientas para categorizar la información y tomar una decisión, transfiriendo el conocimiento adquirido en cada una. Por su parte, el sistema de Replay Memory le proveé información al agente de experiencias pasadas para entender y resolver entornos desconocidos. A su vez, el algoritmo se basa en un modelo de entrenamiento redes Q profundas (DQN, por sus siglas en inglés Deep Q Network), con una recompensa hacia el agente en cada interacción con el entorno. La evaluación del algoritmo se realiza a través de experimentos basados en la interacción con entornos simulados de diferentes tamaños, rutas y caracteracterísticas. | spa |
dc.description.abstract | This project proposes the implementation of an algorithm autonomous navigation based on visual information using deep reinforcement learning. The algorithm aims to teach an agent to identify visual patterns to navigate to a goal in closed and unknown environments. The learning process is made out of three stages: Classification, Imitation and Training, and a Replay Memory system. The Learning stages provide the agent with different tools to classify the information and make a decision, transferring the knowledge acquired in each one. Meanwhile, the replay memory provides the agent information from past experiences to understand and solve unfamiliar environments. At the same time, the algorithm is based on a Deep Q Network (DQN) model, with a reward to the agent in each interaction with the environment. The evaluation of the algorithm is performed through experiments based on the interaction with simulated environments of different sizes, routes and features. | spa |
dc.description.degreelevel | Pregrado | spa |
dc.description.degreename | Ingeniero Electronico | spa |
dc.format.mimetype | application/pdf | spa |
dc.identifier.citation | Aponte Vargas, D. F., y Martínez Méndez, E. D. (2023). Desarrollo de un Algoritmo de Navegación Autónoma Basado en Técnicas de Aprendizaje por Refuerzo Usando Información Visual. [Trabajo de Grado, Universidad Santo Tomás]. Repositorio Institucional. | spa |
dc.identifier.instname | instname:Universidad Santo Tomás | spa |
dc.identifier.reponame | reponame:Repositorio Institucional Universidad Santo Tomás | spa |
dc.identifier.repourl | repourl:https://repository.usta.edu.co | spa |
dc.identifier.uri | http://hdl.handle.net/11634/49272 | |
dc.language.iso | spa | spa |
dc.publisher | Universidad Santo Tomás | spa |
dc.publisher.faculty | Facultad de Ingeniería Electrónica | spa |
dc.publisher.program | Pregrado Ingeniería Electrónica | spa |
dc.relation.references | G. Tesauro, “Temporal difference learning and td-gammon,” Commun. ACM, vol. 38, no. 3, p. 58–68, mar 1995. [Online]. Available: https://doi.org/10.1145/203330.203343 | spa |
dc.relation.references | K. Arulkumaran, M. Deisenroth, M. Brundage, and A. Bharath, “A brief survey of deep reinforcement learning,” IEEE Signal Processing Magazine, vol. 34, 08 2017. | spa |
dc.relation.references | S. J. Russell, P. Norvig, M. C. R. Juan, and J. L. Aguilar. Pearson Educacion, 2011. | spa |
dc.relation.references | “Banco de bogotá y otras organizaciones lideran el proyecto inteligencia artificial colombia: Actualícese,” Aug 2022. [Online]. Available: https://actualicese.com/banco-de-bogota-y-otras-organizaciones-lideran-el-proyecto-inteligencia-artificial-colombia/ | spa |
dc.relation.references | J. Zhong, C. Ling, A. Cangelosi, A. Lotfi, and X. Liu, “On the gap between domestic robotic applications and computational intelligence,” Electronics, vol. 10, no. 7, 2021. [Online]. Available: https://www.mdpi.com/2079-9292/10/7/793 | spa |
dc.relation.references | F. Zeng, C. Wang, and S. Ge, “A survey on visual navigation for artificial agents with deep reinforcement learning,” IEEE Access, vol. PP, 07 2020. | spa |
dc.relation.references | G. o. J. METI, “Japan’s new robot strategy,” p. 6, 04 2018. | spa |
dc.relation.references | M. D. G. V. A. L. P. E. N. G. C. S. F. N. T. J. CRISTINA URDIALES GARCÍA, JUAN ANTONIO FERNÁNDEZ BERNAT, “https://sd2.ugr.es/wpcontent/uploads/2019/10/losrobotsparaelcuidadodelosmayores.pdf,” p. 13, 2017 | spa |
dc.relation.references | C.-A. Smarr, T. Mitzner, J. Beer, A. Prakash, T. Chen, C. Kemp, and W. Rogers, “Domestic robots for older adults: Attitudes, preferences, and potential,” International journal of social robotics, vol. 6, pp. 229– 247, 04 2014. | spa |
dc.relation.references | DANE’, “Personas mayores en colombia.” [Online]. Available: https://www.dane.gov.co/files/investigaciones/notas-estadisticas/ nov-2021-nota-estadistica-personas-mayores-en-colombia.pdf | spa |
dc.relation.references | W. Quesada, “Generación de comportamientos de enjambre en robots móviles a través del uso del aprendizaje por refuerzo.” 03 2019 | spa |
dc.relation.references | P. Mirowski, R. Pascanu, F. Viola, H. Soyer, A. Ballard, A. Banino, M. Denil, R. Goroshin, L. Sifre, K. Kavukcuoglu, D. Kumaran, and R. Hadsell, “Learning to navigate in complex environments,” 11 2016. | spa |
dc.relation.references | V. Mnih, K. Kavukcuoglu, D. Silver, A. Graves, I. Antonoglou, D. Wierstra, and M. Riedmiller, “Playing atari with deep reinforcement learning,” 12 2013. | spa |
dc.relation.references | A. Perez, A. Gomez Garcia, E. Rojas-Martínez, C. Rodríguez-Rojas, J. Lopez-Jimenez, and J. Calderon, “Edge detection algorithm based on fuzzy logic theory for a local vision system of robocup humanoid league,” Tecno Lógicas, vol. 30, pp. 33–50, 06 2013. | spa |
dc.relation.references | J. Calderon, A. Obando, and D. Jaimes, “Road detection algorithm for an autonomous ugv based on monocular vision,” in Proceedings of the Electronics, Robotics and Automotive Mechanics Conference, ser. CERMA ’07. USA: IEEE Computer Society, 2007, p. 253–259. | spa |
dc.relation.references | G. Cardona and J. Calderon, “Robot swarm navigation and victim detection using rendezvous consensus in search and rescue operations,” Applied Sciences, vol. 9, p. 1702, 04 2019. | spa |
dc.relation.references | J. Leon Leon, G. Cardona, A. Botello, and J. Calderon, “Robot swarms theory applicable to seek and rescue operation,” 12 2016. | spa |
dc.relation.references | G. A. Cardona, C. Bravo, W. Quesada, D. Ruiz, M. Obeng, X. Wu, and J. M. Calderon, “Autonomous navigation for exploration of unknown environments and collision avoidance in mobile robots using reinforcement learning,” in 2019 SoutheastCon, 2019, pp. 1–7. | spa |
dc.relation.references | F. S. Caparrini and W. W. Work, “Introducción al aprendizaje automático.” [Online]. Available: http://www.cs.us.es/~fsancho/?e=75 | spa |
dc.relation.references | R. S. Sutton, F. Bach, and A. G. Barto, 1. MIT Press Ltd, 2018. | spa |
dc.relation.references | T. Matiisen, “Demystifying deep reinforcement learning,” Dec 2015. [Online]. Available: https://neuro.cs.ut.ee/ demystifying-deep-reinforcement-learning/ | spa |
dc.relation.references | M. Vallejo del Moral, 2021. [Online]. Available: https://academica-e.unavarra.es/bitstream/handle/2454/40521/ TFG_Mikel_Vallejo.pdf?sequence=1&isAllowed=y | spa |
dc.relation.references | F. Zhuang, Z. Qi, K. Duan, D. Xi, Y. Zhu, H. Zhu, H. Xiong, and Q. He, “A comprehensive survey on transfer learning,” Proceedings of the IEEE, vol. PP, pp. 1–34, 07 2020. | spa |
dc.relation.references | J. Hua, L. Zeng, G. Li, and Z. Ju, “Learning for a robot: Deep reinforcement learning, imitation learning, transfer learning,” Sensors, vol. 21, no. 4, 2021. [Online]. Available: https://www.mdpi.com/ 1424-8220/21/4/1278 | spa |
dc.relation.references | Z. Lőrincz, “A brief overview of imitation learning,” Sep 2019. [Online]. Available: https://smartlabai.medium.com/ a-brief-overview-of-imitation-learning-8a8a75c44a9c | spa |
dc.relation.references | M. Lahtela and P. P. Kaplan, “¿qué es una red neuronal?” 1966. [Online]. Available: https://aws.amazon.com/es/what-is/ neural-network/ | spa |
dc.rights | Atribución-NoComercial-SinDerivadas 2.5 Colombia | * |
dc.rights.accessrights | info:eu-repo/semantics/openAccess | |
dc.rights.coar | http://purl.org/coar/access_right/c_abf2 | spa |
dc.rights.local | Abierto (Texto Completo) | spa |
dc.rights.uri | http://creativecommons.org/licenses/by-nc-nd/2.5/co/ | * |
dc.subject.keyword | Deep Reinforcement Learning | spa |
dc.subject.keyword | Replay Memory | spa |
dc.subject.keyword | Deep Q Networks | spa |
dc.subject.keyword | Autonomous Navigation | spa |
dc.subject.keyword | Visual Information | spa |
dc.subject.lemb | Robótica | spa |
dc.subject.lemb | Machine Learning | spa |
dc.subject.lemb | Ingeniería Electrónica | spa |
dc.subject.proposal | Aprendizaje Profundo por Refuerzo | spa |
dc.subject.proposal | Redes Q Profundas | spa |
dc.subject.proposal | Replay Memory | spa |
dc.subject.proposal | Navegación Autónoma | spa |
dc.subject.proposal | Información Visual | spa |
dc.title | Desarrollo de un Algoritmo de Navegación Autónoma Basado en Técnicas de Aprendizaje por Refuerzo Usando Información Visual | spa |
dc.type | bachelor thesis | |
dc.type.coar | http://purl.org/coar/resource_type/c_7a1f | |
dc.type.coarversion | http://purl.org/coar/version/c_ab4af688f83e57aa | |
dc.type.drive | info:eu-repo/semantics/bachelorThesis | |
dc.type.local | Tesis de pregrado | spa |
dc.type.version | info:eu-repo/semantics/acceptedVersion |
Archivos
Bloque original
1 - 3 de 3
Cargando...
- Nombre:
- 2023DanielAponteErikaMartinez.pdf
- Tamaño:
- 15.65 MB
- Formato:
- Adobe Portable Document Format
- Descripción:
- Trabajo de Grado
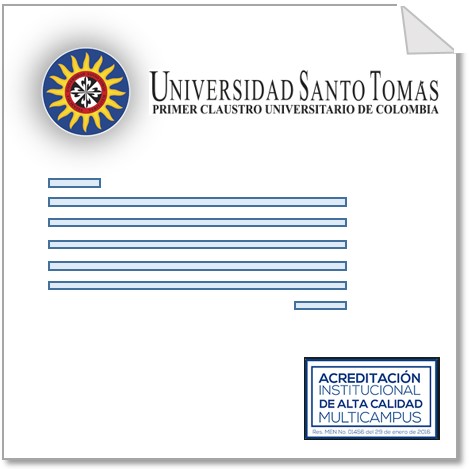
- Nombre:
- Carta_aprobacion_Biblioteca APONTE Y MARTINEZ.pdf
- Tamaño:
- 344.25 KB
- Formato:
- Adobe Portable Document Format
- Descripción:
- Carta Aprobacion Facultad
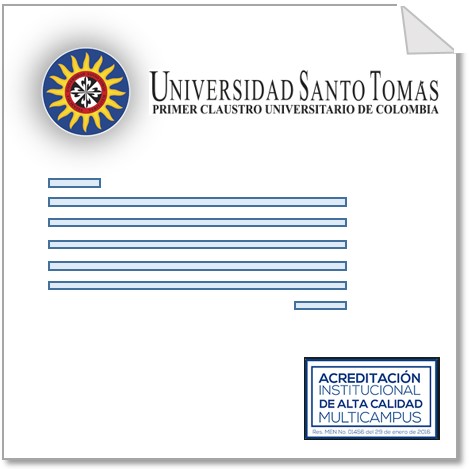
- Nombre:
- CartaDerechosAutorDanielAponteErikaMartinez.pdf
- Tamaño:
- 1.25 MB
- Formato:
- Adobe Portable Document Format
- Descripción:
- Carta Derechos de Autor
Bloque de licencias
1 - 1 de 1
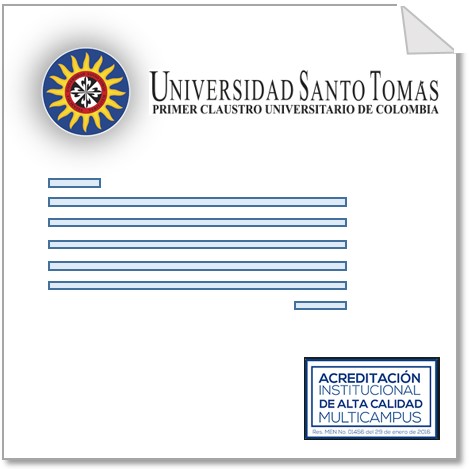
- Nombre:
- license.txt
- Tamaño:
- 807 B
- Formato:
- Item-specific license agreed upon to submission
- Descripción: