Sistema de Identificación de Objetos en Espacios Cerrados Basado en Segmentación Semántica
dc.contributor.advisor | Calderon Chávez, Juan Manuel | |
dc.contributor.author | Sarria Arteaga, Angela Maria | |
dc.contributor.author | Rojas Guayambuco, Angela Maria | |
dc.contributor.corporatename | Universidad Santo Tomás | spa |
dc.contributor.cvlac | https://scienti.minciencias.gov.co/cvlac/visualizador/generarCurriculoCv.do?cod_rh=0000380938 | spa |
dc.contributor.orcid | https://orcid.org/0000-0002-4471-3980 | spa |
dc.coverage.campus | CRAI-USTA Bogotá | spa |
dc.date.accessioned | 2023-11-28T20:47:17Z | |
dc.date.available | 2023-11-28T20:47:17Z | |
dc.date.issued | 2023-07 | |
dc.description | Este proyecto propone realizar el reconocimiento de objetos en entornos cerrados basado en segmentación semántica utilizando redes neuronales profundas. Para lograr esto, se han selec- cionado dos arquitecturas de referencia ampliamente utilizadas en el campo de la visión: YOLO (You Only Look Once, YOLOv7) y Mask R-CNN. La elección de la arquitectura YOLOv7 para la detección de objetos se debe a su capacidad para identificar objetos de manera eficiente en tiempo real. YOLO utiliza una única iteración para detectar objetos en una imagen, lo que la hace especialmente adecuada para aplicaciones donde la velocidad es un factor crítico. Por otro lado, la arquitectura Mask R-CNN se seleccionó para abordar la tarea de la segmen- tación semántica. Esta permite asignar una máscara a cada objeto detectado, lo que brinda información detallada de la forma precisa de cada objeto en la imagen. Ambas arquitecturas, YOLO y Mask R-CNN, se han entrenado y evaluado utilizando la reco- nocida base de datos COCO (Common Objects in Context). COCO ofrece una amplia variedad de imágenes etiquetadas y anotadas, lo que permite entrenar las redes neuronales en un conjunto diverso de categorías de objetos y contextos. | spa |
dc.description.abstract | This project proposes to perform object recognition in indoor environments based on semantic segmentation using deep neural networks. To achieve this, two reference architectures widely used in the vision field have been selected: YOLO (YOLOv7) and Mask R-CNN. The choice of the YOLOv7 architecture for object detection is due to its ability to efficiently identify objects in real time. YOLO uses a single pass through the neural network to detect objects in an image, making it especially suitable for real-time applications where speed is a critical factor. On the other hand, the Mask R-CNN architecture was selected to address the task of semantic segmentation. It is used for mask assigment to each detected object, which provides detailed information on the precise shape of each object in the image. Both architectures, YOLO and Mask R-CNN, have been trained and evaluated using the well- known COCO (Common Objects in Context) database. COCO provides a wide variety of labeled and annotated images, allowing neural networks to be trained on a diverse set of object cate- gories and contexts. | spa |
dc.description.degreelevel | Pregrado | spa |
dc.description.degreename | Ingeniero Electronico | spa |
dc.format.mimetype | application/pdf | spa |
dc.identifier.citation | Sarria Arteaga, A. M. y Rojas Guayambuco, A. M. (2023). Sistema de Identificación de Objetos en Espacios Cerrados Basado en Segmentación Semántica. [Trabajo de Grado, Universidad Santo Tomas´]. Reportorio Institucional. | spa |
dc.identifier.instname | instname:Universidad Santo Tomás | spa |
dc.identifier.reponame | reponame:Repositorio Institucional Universidad Santo Tomás | spa |
dc.identifier.repourl | repourl:https://repository.usta.edu.co | spa |
dc.identifier.uri | http://hdl.handle.net/11634/53053 | |
dc.language.iso | spa | spa |
dc.publisher | Universidad Santo Tomás | spa |
dc.publisher.faculty | Facultad de Ingeniería Electrónica | spa |
dc.publisher.program | Pregrado Ingeniería Electrónica | spa |
dc.relation.references | James et al. Image Processing and Computer Vision. 1999. DOI: 10.1007/0-387-24579- 0_5. URL: https://doi.org/10.1007/0-387-24579-0_5. | spa |
dc.relation.references | Pedro Meseguer Gonzalez y Ramon Lopez de Mantaras Badia. Inteligencia artificial. Edito rial CSIC Consejo Superior de Investigaciones Cientificas, 2017, pág. 159. ISBN: 9788400102340. URL: https://elibro.net/es/lc/usta/titulos/42319. | spa |
dc.relation.references | Xingchao Yan et al. «RAFNet: RGB-D attention feature fusion network for indoor seman tic segmentation». En: Displays 70 (2021), pág. 102082. ISSN: 0141-9382. DOI: https:// doi.org/10.1016/j.displa.2021.102082. URL: https://www.sciencedirect. com/science/article/pii/S0141938221000883. | spa |
dc.relation.references | Jinming Cao et al. «RGBD: Learning Depth-Weighted RGB Patches for RGB-D Indoor Semantic Segmentation». En: Neurocomput. 462 (C oct. de 2021), págs. 568-580. ISSN: 0925- 2312. DOI: 10.1016/j.neucom.2021.08.009. URL: https://doi.org/10.1016/ j.neucom.2021.08.009. | spa |
dc.relation.references | «Segmentación semántica para reconocimiento de escenas». En: 6.19 (2019), págs. 6-7. URL: http://doi.org/10.1109/ICIP.2014.7025197. | spa |
dc.relation.references | Amin Valizadeh y Morteza Shariatee. «The Progress of Medical Image Semantic Segmen tation Methods for Application in COVID-19 Detection». En: Computational intelligence and neuroscience 2021 (nov. de 2021), pág. 7265644. ISSN: 1687-5273. DOI: 10.1155/2021/ 7265644. URL: https : / / pubmed . ncbi . nlm . nih . gov / 34840563 % 20https : //www.ncbi.nlm.nih.gov/pmc/articles/PMC8611358/. | spa |
dc.relation.references | Bike Chen, Chen Gong y Jian Yang. «Importance-Aware Semantic Segmentation for Au tonomous Vehicles». En: IEEE Transactions on Intelligent Transportation Systems 20.1 (2019), págs. 137-148. DOI: 10.1109/TITS.2018.2801309 | spa |
dc.relation.references | Junho Jo et al. «Handwritten Text Segmentation via End-to-End Learning of Convolutio nal Neural Networks». En: Multimed Tools Applications (jun. de 2019). | spa |
dc.relation.references | Mohamed Chouai, Mostefa Merah y Malika Mimi. «CH-Net: Deep adversarial autoenco ders for semantic segmentation in X-ray images of cabin baggage screening at airports». En: Journal of Transportation Security 13 (1 2020), págs. 71-89. ISSN: 1938-775X. DOI: 10. 1007/s12198-020-00211-5. URL: https://doi.org/10.1007/s12198-020- 00211-5. | spa |
dc.relation.references | Javier A Cardenas et al. «Intelligent Position Controller for Unmanned Aerial Vehicles (UAV) Based on Supervised Deep Learning». En: Machines 11.6 (2023), pág. 606. | spa |
dc.relation.references | Luis G Jaimes y Juan M Calderon. «An UAV-based incentive mechanism for Crowdsen sing with budget constraints». En: 2020 IEEE 17th Annual Consumer Communications & Networking Conference (CCNC). IEEE. 2020, págs. 1-6 | spa |
dc.relation.references | GA Cardona et al. «Autonomous navigation for exploration of unknown environments and collision avoidance in mobile robots using reinforcement learning». En: 2019 Southeast Con. IEEE. 2019, págs. 1-7. | spa |
dc.relation.references | Javier Alexis Cárdenas et al. «Optimal PID ø axis Control for UAV Quadrotor based on Multi-Objective PSO». En: IFAC-PapersOnLine 55.14 (2022), págs. 101-106. | spa |
dc.relation.references | Wilson O Quesada et al. «Leader-follower formation for UAV robot swarm based on fuzzy logic theory». En: Artificial Intelligence and Soft Computing: 17th International Confe rence, ICAISC 2018, Zakopane, Poland, June 3-7, 2018, Proceedings, Part II 17. Springer. 2018, págs. 740-751. | spa |
dc.relation.references | César Antonio Toro. «Algoritmos de segmentación semántica para anotación de imáge nes». En: (2019). URL: http://oa.upm.es/55407/1/CESAR_ANTONIO_ORTIZ_ TORO.pdf. | spa |
dc.relation.references | Olanda Prieto Ordaz y David Maloof Flores. «Segmentación Semántica para Reconoci miento de Escenas». En: FINGUACH. Revista de Investigación Científica de la Facultad de Ingeniería de la Universidad Autónoma de Chihuahua 6.19 (jun. de 2019), págs. 6, 7. URL: https://vocero.uach.mx/index.php/finguach/article/view/37 | spa |
dc.relation.references | Alejandro Barrera et al. Análisis, evaluación e implementación de algoritmos de segmentación semántica para su aplicación en vehículos inteligentes. Universidad Carlos III de Madrid, sep. de 2018. URL: https://github.com/tzutalin/ros_caffe. | spa |
dc.relation.references | Yu-Jin Zhang. «Image Segmentation in the Last 40 Years». En: https://services.igi-global.com/resolvedoi/resolve.aspx?doi=10.4018/978- 1-60566-026-4.ch286 (ene. de 2009), págs. 1818-1823. DOI: 10.4018/978- 1- 60566- 026-4.CH286. URL: https://www.igi-global.com/chapter/image-segmentation last-years/13824%20www.igi-global.com/chapter/image-segmentation last-years/13824 | spa |
dc.relation.references | Keith Foote. «A Brief History of Semantics - DATAVERSITY». En: (mayo de 2016). URL: https://www.dataversity.net/brief-history-semantics/. | spa |
dc.relation.references | Eai Fund Official. «History of image segmentation». En: (oct. de 2018). URL: https:// medium.com/@eaifundoffical/history-of-image-segmentation-655eb793559a. | spa |
dc.relation.references | Dhruv Parthasarathy. «A Brief History of CNNs in Image Segmentation: From R-CNN to Mask R-CNN | by Dhruv Parthasarathy | Athelas». En: Athelas (abr. de 2017). URL: https : / / blog . athelas . com / a - brief - history - of - cnns - in - image - segmentation-from-r-cnn-to-mask-r-cnn-34ea83205de4 | spa |
dc.relation.references | Xuming He, Richard S Zemel y Miguel Á Carreira-Perpiñán. «Multiscale Conditional Random Fields for Image Labeling». En: Proceedings of the 2004 IEEE Computer Society Conference on Computer Vision and Pattern Recognition (2004), págs. 695-703 | spa |
dc.relation.references | John et al. «TextonBoost: Joint Appearance, Shape and Context Modeling for Multi-class Object Recognition and Segmentation». En: Computer Vision – ECCV 2006 (2006). Ed. por Horst, Pinz Axel Leonardis Aleš y Bischof, págs. 1-15. | spa |
dc.relation.references | Torralba et al. «Context-based vision system for place and object recognition». En: Pro ceedings Ninth IEEE International Conference on Computer Vision (2003), 273-280 vol.1. DOI: 10.1109/ICCV.2003.1238354. | spa |
dc.relation.references | Lijun Ren y Yuansheng Liu. «Research on the Application of Semantic Segmentation of driverless vehicles in Park Scene». En: 2020, págs. 342-345. DOI: 10.1109/ISCID51228. 2020.00083. | spa |
dc.relation.references | Changshuo Wang et al. «A brief survey on RGB-D semantic segmentation using deep learning». En: Displays 70 (2021), pág. 102080. ISSN: 0141-9382. DOI: https : / / doi . org/10.1016/j.displa.2021.102080. URL: https://www.sciencedirect. com/science/article/pii/S014193822100086X | spa |
dc.relation.references | Pedro Ignacio Orellana Rueda. «SEGMENTACIÓN SEMÁNTICA Y RECONOCIMIEN TO DE LUGARES USANDO CARACTERÍSTICAS CNN PREENTRENADAS». Universi dad de chile, 2019. URL: https://repositorio.uchile.cl/bitstream/handle/ 2250/173733/cf-orellana_pr.pdf?sequence=1&isAllowed=y | spa |
dc.relation.references | Derek et al. «Indoor Segmentation and Support Inference from RGBD Images». En: Com puter Vision – ECCV 2012 (2012). Ed. por Svetlana et al., págs. 746-760. | spa |
dc.relation.references | Kaiming He et al. «Mask R-CNN». En: 2017 IEEE International Conference on Computer Vision (ICCV) (2017), págs. 2980-2988. DOI: 10.1109/ICCV.2017.322. | spa |
dc.relation.references | Xin Wang et al. «Path and Floor Detection in Outdoor Environments for Fall Prevention of the Visually Impaired Population». En: 2022 IEEE 19th Annual Consumer Communications & Networking Conference (CCNC). IEEE. 2022, págs. 1-6. | spa |
dc.relation.references | Gustavo A Cardona et al. «Visual victim detection and quadrotor-swarm coordination control in search and rescue environment». En: International Journal of Electrical and Com puter Engineering 11.3 (2021), pág. 2079. | spa |
dc.relation.references | Seungyong Lee, Seong-Jin Park y Ki-Sang Hong. «RDFNet: RGB-D Multi-level Residual Feature Fusion for Indoor Semantic Segmentation». En: 2017 IEEE International Conference on Computer Vision (ICCV) (2017), págs. 4990-4999. DOI: 10.1109/ICCV.2017.533. | spa |
dc.relation.references | Yang He et al. «STD2P: RGBD Semantic Segmentation Using Spatio-Temporal Data-Driven Pooling». En: (2017), págs. 7158-7167. DOI: 10.1109/CVPR.2017.757. | spa |
dc.relation.references | Laura J Padilla Reyes et al. «Adaptable Recommendation System for Outfit Selection with Deep Learning Approach». En: IFAC-PapersOnLine 54.13 (2021), págs. 605-610. | spa |
dc.relation.references | Steven Ricardo Castro Ramirez y Oscar Felipe Roncancio Avendaño. «Desarrollo de sis tema de navegación para un vehículo terrestre basado en segmentación semántica para ambientes internos controlados». Universidad Piloto de Colombia, ago. de 2021. URL: http://repository.unipiloto.edu.co/handle/20.500.12277/10841 | spa |
dc.relation.references | Wenbang Deng et al. «Semantic RGB-D SLAM for Rescue Robot Navigation». En: IEEE Access 8 (oct. de 2020), págs. 221320-221329. ISSN: 21693536. DOI: 10.1109/ACCESS. 2020.3031867. | spa |
dc.relation.references | Fude Cao y Qinghai Bao. «A Survey On Image Semantic Segmentation Methods With Convolutional Neural Network». En: 2020 International Conference on Communications, In formation System and Computer Engineering (CISCE). 2020, págs. 458-462. DOI: 10.1109/ CISCE50729.2020.00103. | spa |
dc.relation.references | Jiafan Zhuang, Zilei Wang y Bingke Wang. «Distortion-Aware Feature Correction». En: 31.8 (2021), págs. 3128-3139. | spa |
dc.relation.references | ZHIJIE LIN et al. «Image style transfer algorithm based on semantic segmentation». En: IEEE Access (ene. de 2021). URL: https://ieeexplore.ieee.org/stamp/stamp. jsp?arnumber=9336718. | spa |
dc.relation.references | Fahimeh Fooladgar y Shohreh Kasaei. «A survey on indoor RGB-D semantic segmenta tion: from hand-crafted features to deep convolutional neural networks». En: Multime dia Tools and Applications 79 (7 2020), págs. 4499-4524. ISSN: 1573-7721. DOI: 10.1007/ s11042-019-7684-3. URL: https://doi.org/10.1007/s11042-019-7684-3. | spa |
dc.relation.references | Alejandro de Nova Guerrero. «Detección y segmentación de objetos en imágenes pano rámicas». En: (). | spa |
dc.relation.references | Rui Zhang et al. «A survey on deep learning-based precise boundary recovery of seman tic segmentation for images and point clouds». En: International Journal of Applied Earth Observation and Geoinformation 102 (2021), pág. 102411. ISSN: 1569-8432. DOI: https:// doi.org/10.1016/j.jag.2021.102411. URL: https://www.sciencedirect. com/science/article/pii/S0303243421001185. | spa |
dc.relation.references | Yujian Mo et al. «Review the state-of-the-art technologies of semantic segmentation based on deep learning». En: Neurocomputing 493 (2022), págs. 626-646. ISSN: 0925-2312. DOI: https://doi.org/10.1016/j.neucom.2022.01.005. URL: https://www. sciencedirect.com/science/article/pii/S0925231222000054. | spa |
dc.relation.references | Íñigo Alonso Ruiz. «Segmentación Semántica con Modelos de Deep Learning y Etique tados No Densos». Universidad de Zaragoza, ene. de 2018. URL: https://zaguan. unizar.es/record/69800/files/TAZ-TFM-2018-012.pdf. | spa |
dc.relation.references | Alberto Garcia-Garcia et al. «A Review on Deep Learning Techniques Applied to Se mantic Segmentation». En: (abr. de 2017). URL: https://arxiv.org/abs/1704. 06857v1. | spa |
dc.relation.references | Jeremy Jordan. «An overview of semantic image segmentation.» En: (mayo de 2018). URL: https://www.jeremyjordan.me/semantic-segmentation/. | spa |
dc.relation.references | Larry Hardesty. «Neural networks». En: MIT News Office 14 (5 oct. de 2017), págs. 503-519. ISSN: 17518520. DOI: 10.1007/S11633- 017- 1054- 2. URL: https://news.mit. edu/2017/explained-neural-networks-deep-learning-0414 | spa |
dc.relation.references | Rahul Chauhan, Kamal Kumar Ghanshala y R. C. Joshi. «Convolutional Neural Network (CNN) for Image Detection and Recognition». En: ICSCCC 2018 - 1st International Con ference on Secure Cyber Computing and Communications (jul. de 2018), págs. 278-282. DOI: 10.1109/ICSCCC.2018.8703316. | spa |
dc.relation.references | Vitaly Bushaev. «How do we ‘train’ neural networks». En: Towards data science (nov. de 2017). URL: https://towardsdatascience.com/how-do-we-train-neural networks-edd985562b73. | spa |
dc.relation.references | Arden Dertat. Applied Deep Learning. Nov. de 2017. URL: https://towardsdatascience. com/applied-deep-learning-part-4-convolutional-neural-networks 584bc134c1e2. | spa |
dc.relation.references | Kevin Gurney y New York. An introduction to neural networks. 1997 | spa |
dc.relation.references | Niklas Donges. What Is Transfer Learning? A Guide for Deep Learning | Built In. Sep. de 2022. URL: https://builtin.com/data-science/transfer-learning. | spa |
dc.relation.references | Hyeok June Jeong, Kyeong Sik Park y Young Guk Ha. «Image Preprocessing for Efficient Training of YOLO Deep Learning Networks». En: Proceedings - 2018 IEEE International Conference on Big Data and Smart Computing, BigComp 2018 (mayo de 2018), págs. 635-637. DOI: 10.1109/BIGCOMP.2018.00113. | spa |
dc.relation.references | Rohit Kundu. YOLO Algorithm for Object Detection Explained. Ene. de 2023. URL: https: //www.v7labs.com/blog/yolo-object-detection. | spa |
dc.relation.references | Gaudenz Boesch. YOLOv7: The Fastest Object Detection Algorithm (2023) - viso.ai. URL: https://viso.ai/deep-learning/yolov7-guide/. | spa |
dc.relation.references | Learn OpenCV. YOLOv7 Object Detection Paper Explanation and Inference. 2023. URL: https: //learnopencv.com/yolov7-object-detection-paper-explanation-and inference/. | spa |
dc.relation.references | Zijia Yang et al. «Tea Tree Pest Detection Algorithm Based on Improved Yolov7-Tiny». En: Agriculture 13.5 (2023), pág. 1031. DOI: 10 . xxxx / agriculture13051031. URL: https://www.mdpi.com/2077-0472/13/5/103 | spa |
dc.relation.references | Li Ma et al. «Detection and Counting of Small Target Apples under Complicated Envi ronments by Using Improved YOLOv7-tiny». En: Agronomy 13.5 (2023), pág. 1419. DOI: 10.xxxx/agronomy13051419. URL: https://www.mdpi.com/2073-4395/13/5/ 1419. | spa |
dc.relation.references | Rocío Alvarez-Cedrón García-Zarandieta. Implementación de un modelo de detección y segui miento de jugadores de waterpolo para el análisis de modelos de juego. Grado en Ingeniería de Tecnologías y Servicios de Telecomunicación. Universidad Politécnica de Madrid (UPM). 2020. URL: https://oa.upm.es/62753/. | spa |
dc.relation.references | Kaiming He et al. «Mask R-CNN». En: Proceedings of the IEEE International Conference on Computer Vision (ICCV). Oct. de 2017. | spa |
dc.relation.references | Viso.AI. Everything about Mask R-CNN: A Beginner’s Guide. 2021. URL: https://viso. ai/deep-learning/mask-r-cnn/. | spa |
dc.relation.references | Jacob Solawetz. An Introduction to the COCO Dataset. Oct. de 2020. URL: https://blog. roboflow.com/coco-dataset/ | spa |
dc.relation.references | Amazon Web Services (AWS). Transformación de los conjuntos de datos de COCO. Fecha de acceso. URL: https://docs.aws.amazon.com/es_es/rekognition/latest/ customlabels-dg/md-transform-coco.html | spa |
dc.relation.references | Jacob Solawetz. An Introduction to the COCO Dataset. Oct. de 2020. URL: https://blog. roboflow.com/coco-dataset/#object-detection-with-coco. | spa |
dc.relation.references | Xiaolei Wang et al. «A deep learning method for optimizing semantic segmentation ac curacy of remote sensing images based on improved UNet». En: Scientific reports (2023), págs. 1-10. | spa |
dc.relation.references | Evan Shelhamer, Jonathan Long y Trevor Darrell. Fully Convolutional Networks for Seman tic Segmentation. 2016. arXiv: 1605.06211 [cs.CV]. | spa |
dc.relation.references | Liang-Chien Chen et al. «DeepLab: Semantic Image Segmentation with Deep Convolu tional Nets, Atrous Convolution, and Fully Connected CRFs». En: (mayo de 201 | spa |
dc.relation.references | Wei Liu et al. «SSD: Single Shot MultiBox Detector». En: (2016), págs. 21-37. DOI: 10. 1007/978-3-319-46448-0_2 | spa |
dc.relation.references | Jose León et al. «Robot swarms theory applicable to seek and rescue operation». En: In telligent Systems Design and Applications: 16th International Conference on Intelligent Systems Design and Applications (ISDA 2016) held in Porto, Portugal, December 16-18, 2016. Springer. 2017, págs. 1061-1070. | spa |
dc.relation.references | Gustavo A Cardona y Juan M Calderon. «Robot swarm navigation and victim detection using rendezvous consensus in search and rescue operations». En: Applied Sciences 9.8 (2019), pág. 1702 | spa |
dc.relation.references | David Paez et al. «Distributed particle swarm optimization for multi-robot system in search and rescue operations». En: IFAC-PapersOnLine 54.4 (2021), págs. 1-6. | spa |
dc.relation.references | Nicolás Gómez et al. «Leader-follower behavior in multi-agent systems for search and rescue based on pso approach». En: SoutheastCon 2022. IEEE. 2022, págs. 413-420. | spa |
dc.relation.references | Edgar C Camacho, Nestor I Ospina y Juan M Calderón. «COVID-Bot: UV-C based au tonomous sanitizing robotic platform for COVID-19». En: Ifac-papersonline 54.13 (2021), págs. 317-322. | spa |
dc.relation.references | Gustavo A Cardona et al. «Robust adaptive synchronization of interconnected hetero geneous quadrotors transporting a cable-suspended load». En: 2021 IEEE International Conference on Robotics and Automation (ICRA). IEEE. 2021, págs. 31-37 | spa |
dc.relation.references | Gustavo A Cardona et al. «Adaptive Multi-Quadrotor Control for Cooperative Transpor tation of a Cable-Suspended Load». En: 2021 European Control Conference (ECC). IEEE. 2021, págs. 696-701 | spa |
dc.rights | Atribución-NoComercial-SinDerivadas 2.5 Colombia | * |
dc.rights.accessrights | info:eu-repo/semantics/openAccess | |
dc.rights.coar | http://purl.org/coar/access_right/c_abf2 | spa |
dc.rights.local | Abierto (Texto Completo) | spa |
dc.rights.uri | http://creativecommons.org/licenses/by-nc-nd/2.5/co/ | * |
dc.subject.lemb | Visión Artificial | spa |
dc.subject.lemb | Inteligencia artificial | spa |
dc.subject.lemb | Ingeniería Electrónica | spa |
dc.subject.proposal | Redes neuronales profundas | spa |
dc.subject.proposal | Reconocimiento de objetos | spa |
dc.subject.proposal | Segmentación semántica | spa |
dc.subject.proposal | YOLO | spa |
dc.subject.proposal | Mask R-CNN | spa |
dc.subject.proposal | Detección de objetos | spa |
dc.title | Sistema de Identificación de Objetos en Espacios Cerrados Basado en Segmentación Semántica | spa |
dc.type.coar | http://purl.org/coar/resource_type/c_7a1f | |
dc.type.coarversion | http://purl.org/coar/version/c_ab4af688f83e57aa | |
dc.type.drive | info:eu-repo/semantics/bachelorThesis | |
dc.type.local | Trabajo de grado | spa |
dc.type.version | info:eu-repo/semantics/acceptedVersion |
Archivos
Bloque original
1 - 3 de 3
Cargando...
- Nombre:
- 2023AngelaSarriaAngelaRojas.pdf
- Tamaño:
- 21.72 MB
- Formato:
- Adobe Portable Document Format
- Descripción:
- Articulo trabajo de grado
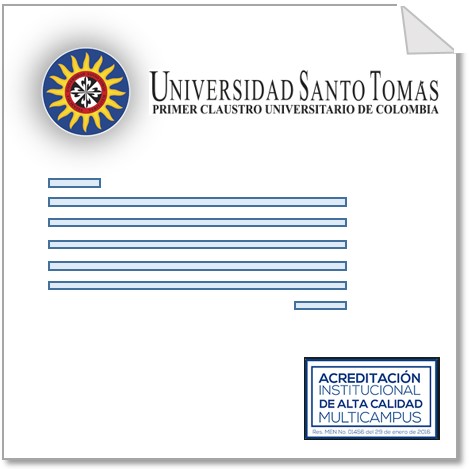
- Nombre:
- Carta_aprobacion_Biblioteca....pdf
- Tamaño:
- 157.37 KB
- Formato:
- Adobe Portable Document Format
- Descripción:
- Carta aprobación facultad
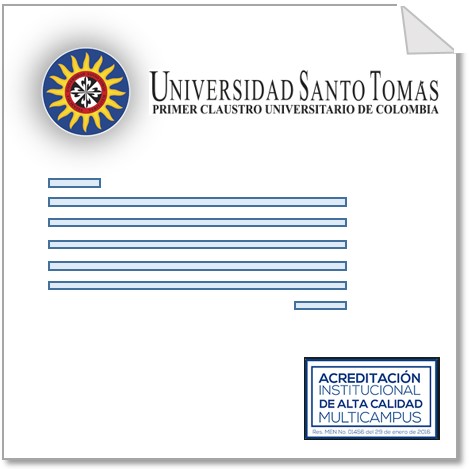
- Nombre:
- Carta_autorizacion_autoarchivo_autor_2021.pdf
- Tamaño:
- 904.01 KB
- Formato:
- Adobe Portable Document Format
- Descripción:
- Carta cesión de derechos
Bloque de licencias
1 - 1 de 1
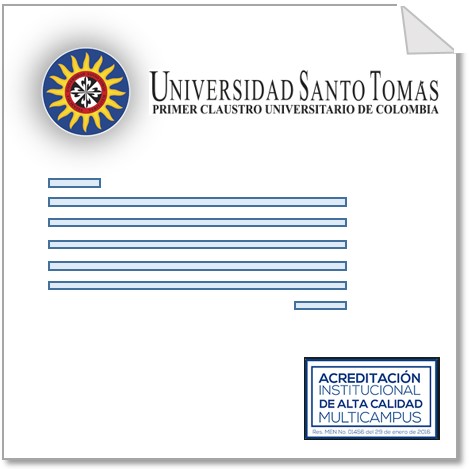
- Nombre:
- license.txt
- Tamaño:
- 807 B
- Formato:
- Item-specific license agreed upon to submission
- Descripción: