Clasificador de Fresa Usando Técnicas de Inteligencia Artificial
dc.contributor.advisor | Pardo Beainy, Camilo Ernesto | |
dc.contributor.advisor | Gutiérrez Cáceres, Edgar Andrés | |
dc.contributor.author | Camargo Robles, Juan José | |
dc.contributor.corporatename | Universidad Santo Tomás | spa |
dc.coverage.campus | CRAI-USTA Tunja | spa |
dc.date.accessioned | 2024-10-01T14:22:32Z | |
dc.date.available | 2024-10-01T14:22:32Z | |
dc.date.issued | 2024 | |
dc.description | En el desarrollo de esta investigación, se implementan varios algoritmos de inteligencia artificial para la clasificación y detección del nivel de madurez de fresa. Las imágenes adquiridas por el sistema de sensado de una banda transportadora, fueron sometidas, por una parte; a algoritmos de Machine Learning y Deep Learning, orientados a la clasificación del fruto en dos categorías, definidas por sus características de madurez y deficiencias nutricionales (afectaciones visuales), y, por otra parte; a algoritmos de Deep Learning, orientados a la extracción de características para determinar su nivel de madurez. Se creó un dataset público desde cero con el objetivo de que esté disponible para cualquiera que lo necesite. Para alcanzar una clasificación satisfactoria, se entrenaron modelos utilizando la arquitectura YOLO, específicamente la versión ocho, lo que permitió realizar la clasificación del fruto con éxito. Posteriormente, se llevaron a cabo pruebas sobre las imágenes previamente clasificadas, empleando técnicas como segmentación de fondo por umbrales y segmentación cromática. Estas técnicas de ingeniería de características facilitaron la detección del nivel de madurez del fruto. | spa |
dc.description.abstract | In the development of this research, several artificial intelligence algorithms are implemented for the classification and detection of strawberry maturity level. The images acquired by the sensing system of a conveyor belt were subjected, on the one hand, to Machine Learning and Deep Learning algorithms, oriented to the classification of the fruit into two categories, defined by their maturity characteristics and nutritional deficiencies (visual affectations), and, on the other hand, to Deep Learning algorithms, oriented to the extraction of features to determine their maturity level. A public dataset was created from scratch with the aim of making it available to anyone who needs it. To achieve a satisfactory classification, models were trained using the YOLO architecture, specifically the YOLO architecture, specifically version eight, which allowed us to successfully classify the fruit. fruit classification successfully. Subsequently, tests were carried out on the previously classified images, using techniques such as segmentation were then tested on the previously classified images, using techniques such as thresholded background segmentation and chromatic segmentation. chromatic segmentation. These feature engineering techniques facilitated the detection of fruit maturity level. maturity level of the fruit. | spa |
dc.description.degreelevel | Pregrado | spa |
dc.description.degreename | Ingeniero Electronico | spa |
dc.format.mimetype | application/pdf | spa |
dc.identifier.citation | Camargo, J. (2024). Clasificador de Fresa Usando Técnicas de Inteligencia Artificial. [Trabajo de Grado, Universidad Santo Tomás]. Repositorio Institucional | spa |
dc.identifier.instname | instname:Universidad Santo Tomás | spa |
dc.identifier.reponame | reponame:Repositorio Institucional Universidad Santo Tomás | spa |
dc.identifier.repourl | repourl:https://repository.usta.edu.co | spa |
dc.identifier.uri | http://hdl.handle.net/11634/58025 | |
dc.language.iso | spa | spa |
dc.publisher | Universidad Santo Tomás | spa |
dc.publisher.faculty | Facultad de Ingeniería Electrónica | spa |
dc.publisher.program | Pregrado Ingeniería Electrónica | spa |
dc.relation.references | D. N. Baker, J. R. Lambert, J. M. McKinion, and S. C. A. E. Station, GOSSYM: A Simulator of Cotton Crop Growth and Yield. in Technical bulletin (South Carolina Agricultural Experiment Station). S.C. Agricultural Experiment Station, 1983. [Online]. Available: https://books.google.com.co/books?id=Y7SErgEACAAJ | spa |
dc.relation.references | G. Bannerjee, U. Sarkar, S. Das, and I. Ghosh, “Artificial intelligence in agriculture: A literature survey,” Int J Sci Res Comput Sci Appl Manag Stud, vol. 7, no. 3, pp. 1–6, 2018. | spa |
dc.relation.references | N. C. Eli-Chukwu, “Applications of artificial intelligence in agriculture: A review.,” Engineering, Technology & Applied Science Research, vol. 9, no. 4, 2019. | spa |
dc.relation.references | T. M. Mitchell, Machine Learning. in McGraw-Hill International Editions. McGraw-Hill, 1997. [Online]. Available: https://books.google.com.co/books?id=EoYBngEACAAJ | spa |
dc.relation.references | Minagricultura, “Cadena de la Fresa,” Dirección de Cadenas Agrícolas y Forestales, Mar. 2021. | spa |
dc.relation.references | Gobernación de Boyacá, “Caracterización de la Producción Agrícola del Departamento de Boyacá,” Boletín Red de Observatorios de Boyacá, vol. 1, no. 4, 2022. | spa |
dc.relation.references | D. A. Forsyth and J. Ponce, Computer Vision: A Modern Approach: International Edition. Pearson Education, 2015. https://books.google.com.co/books?id=pAWpBwAAQBAJ | spa |
dc.relation.references | V. Por Computador Editado, E. Alegre, G. Pajares, and A. De La Escalera, “Conceptos y Métodos en,” 2016. | spa |
dc.relation.references | L. Enrique Sucar and M. Giovani Gómez, “Visión Computacional.” | spa |
dc.relation.references | M. Jordan, J. Kleinberg, and B. Schölkopf, “Pattern Recognition and Machine Learning.” | spa |
dc.relation.references | N. L. Montes Castrillón, “Segmentación de imágenes de frutos de café en el proceso de beneficio.” [Online]. Available: https://repositorio.unal.edu.co/handle/unal/2846 | spa |
dc.relation.references | I. Goodfellow, Y. Bengio, and A. Courville, Deep Learning. in Adaptive Computation and Machine Learning series. MIT Press, 2016. https://books.google.com.co/books?id=Np9SDQAAQBAJ | spa |
dc.relation.references | J. Bobadilla, Machine Learning y Deep Learning: Usando Python, Scikit y Keras. Ediciones de U, 2021. https://books.google.com.co/books?id=iAAyEAAAQBAJ | spa |
dc.relation.references | F. Chollet, Deep Learning with Python, 1st ed. USA: Manning Publications Co., 2017. | spa |
dc.relation.references | M. Z. Rodriguez et al., “Clustering algorithms: A comparative approach,” PLoS One, vol. 14, no. 1, Jan. 2019, doi: 10.1371/journal.pone.0210236. | spa |
dc.relation.references | G. Zaccone and M. R. Karim, Deep Learning with TensorFlow: Explore neural networks and build intelligent systems with Python, 2nd Edition. Packt Publishing, 2018. [Online]. Available: https://books.google.com.co/books?id=zZlUDwAAQBAJ | spa |
dc.relation.references | Glenn Jocher and Muhammad Rizwan Munawar, “Ultralytics YOLO Docs,” Ultralitycs Inc. | spa |
dc.relation.references | N. Ismail and O. A. Malik, “Real-time visual inspection system for grading fruits using computer vision and deep learning techniques,” Information Processing in Agriculture, vol. 9, no. 1, pp. 24–37, Mar. 2022, doi: 10.1016/j.inpa.2021.01.005. | spa |
dc.relation.references | H. Zhou, Z. Zhuang, Y. Liu, Y. Liu, and X. Zhang, “Defect classification of green plums based on deep learning,” Sensors (Switzerland), vol. 20, no. 23, pp. 1–15, Dec. 2020, doi: 10.3390/s20236993. | spa |
dc.relation.references | D. Heras, “Clasificador de imágenes de frutas basado en inteligencia artificial,” Killkana Técnica, vol. 1, no. 2, p. 21, Nov. 2017, doi: 10.26871/killkana_tecnica.v1i2.79. | spa |
dc.relation.references | P. Constante, A. Gordon, O. Chang, E. Pruna, F. Acuna, and I. Escobar, “Artificial Vision Techniques to Optimize Strawberry’s Industrial Classification,” IEEE Latin America Transactions, vol. 14, no. 6, pp. 2576–2581, 2016, doi: 10.1109/TLA.2016.7555221. | spa |
dc.relation.references | J. Victor Aguilar-Alvarado and M. Alfredo Campoverde-Molina, “Classification of fruits based on convolutional neural networks Classificação de frutos com base em redes neurais convolucionais Ciencias de la ingeniería Artículo de investigación,” vol. 5, no. 01, pp. 3 22, 2019, doi: 10.23857/pc.v5i01.1210. | spa |
dc.relation.references | E. De Postgrado, “UNIVERSIDAD PRIVADA ANTENOR ORREGO "ESPECTROSCOPIA CON INFRARROJO Y TECNICAS DE MACHINE LEARNING Y DEEP LEARNING PARA LA DETECCIÓN Y CLASIFICACIÓN DE FRUTAS PARA LA AGROINDUSTRIA. CASO: ARÁNDANOS-EMPRESA TalSA-2018 ".” | spa |
dc.relation.references | A. Pajaziti, F. Basholli, and Y. Zhaveli, “Identification and classification of fruits through robotic system by using artificial intelligence,” Engineering Applications, vol. 2, no. 2, pp. 154–163, May 2023, [Online]. https://publish.mersin.edu.tr/index.php/enap/article/view/974 | spa |
dc.relation.references | Y. Fonseca, C. Bautista, C. Pardo-Beainy, and C. Parra, “A plum selection system that uses a multi-class Convolutional Neural Network (CNN),” J Agric Food Res, vol. 14, p. 100793, 2023, doi: https://doi.org/10.1016/j.jafr.2023.100793. | spa |
dc.relation.references | J. P. Bonilla-González and F. A. Prieto-Ortiz, “Determinación del estado de maduración de frutos de feijoa mediante un sistema de visión por computador utilizando información de color,” REVISTA DE INVESTIGACIÓN, DESARROLLO E INNOVACIÓN, vol. 7, no. 1, p. 111, Dec. 2016, doi: 10.19053/20278306.v7.n1.2016.5603. | spa |
dc.relation.references | S. Yang, W. Wang, S. Gao, and Z. Deng, “Strawberry ripeness detection based on YOLOv8 algorithm fused with LW-Swin Transformer,” Comput Electron Agric, vol. 215, p. 108360, 2023, doi: https://doi.org/10.1016/j.compag.2023.108360. | spa |
dc.relation.references | A. Almutairi, J. Alharbi, S. Alharbi, H. F. Alhasson, S. S. Alharbi, and S. Habib, “Date Fruit Detection and Classification based on Its Variety Using Deep Learning Technology,” IEEE Access, p. 1, 2024, doi: 10.1109/ACCESS.2024.3433485. | spa |
dc.relation.references | C. Dewi, O. M. Kamlasi, G. Chhabra, G. Dai, K. Kaushik, and I. U. Khan, “Automated Fruit Classification Based on Deep Learning Utilizing Yolov8,” in 2023 10th IEEE Uttar Pradesh Section International Conference on Electrical, Electronics and Computer Engineering (UPCON), 2023, pp. 801–807. doi: 10.1109/UPCON59197.2023.10434542. | spa |
dc.relation.references | T. S. Gunawan, M. Kartiwi, H. Mansor, and N. M. Yusoff, “Palm Fruit Ripeness Detection and Classification Using Various YOLOv8 Models,” in 2023 IEEE 9th International Conference on Smart Instrumentation, Measurement and Applications (ICSIMA), 2023, pp. 193–198. doi: 10.1109/ICSIMA59853.2023.10373435. | spa |
dc.relation.references | D.-L. Nguyen, X.-T. Vo, A. Priadana, M. D. Putro, and K.-H. Jo, “Fruit Ripeness Detector for Automatic Fruit Classification Systems,” in 2024 IEEE 33rd International Symposium on Industrial Electronics (ISIE), 2024, pp. 1–6. doi: 10.1109/ISIE54533.2024.10595723. | spa |
dc.relation.references | C. Tang et al., “A fine recognition method of strawberry ripeness combining Mask R-CNN and region segmentation,” Front Plant Sci, vol. 14, 2023, doi: 10.3389/fpls.2023.1211830. | spa |
dc.relation.references | C. C. Tran, D. T. Nguyen, H. D. Le, Q. B. Truong, and Q. D. Truong, “Automatic dragon fruit counting using adaptive thresholds for image segmentation and shape analysis,” in 2017 4th NAFOSTED Conference on Information and Computer Science, 2017, pp. 132 137. doi: 10.1109/NAFOSTED.2017.8108052. | spa |
dc.relation.references | P. Constante, A. Gordon, O. Chang, E. Pruna, F. Acuna, and I. Escobar, “Artificial Vision Techniques to Optimize Strawberry’s Industrial Classification,” IEEE Latin America Transactions, vol. 14, no. 6, pp. 2576–2581, 2016, doi: 10.1109/TLA.2016.7555221. | spa |
dc.relation.references | Y.-T. Kim, “Contrast enhancement using brightness preserving bi-histogram equalization,” IEEE Transactions on Consumer Electronics, vol. 43, no. 1, pp. 1–8, 1997, doi: 10.1109/30.580378. | spa |
dc.relation.references | R. G. Apaza, C. E. Portugal-Zambrano, J. C. Gutiérrez-Cáceres, and C. A. Beltrán Castañón, “An approach for improve the recognition of defects in coffee beans using retinex algorithms,” in 2014 XL Latin American Computing Conference (CLEI), 2014, pp. 1–9. doi: 10.1109/CLEI.2014.6965102. | spa |
dc.relation.references | L. Yang, D. Mu, Z. Xu, and K. Huang, “Apple Surface Defect Detection Based on Gray Level Co-Occurrence Matrix and Retinex Image Enhancement,” Applied Sciences (Switzerland), vol. 13, no. 22, Nov. 2023, doi: 10.3390/app132212481. | spa |
dc.relation.references | D. Haba, Data Augmentation with Python: Enhance deep learning accuracy with data augmentation methods for image, text, audio, and tabular data. Packt Publishing, 2023. [Online]. Available: http://ieeexplore.ieee.org/document/10251193 | spa |
dc.relation.references | J. T. Viñals, Python Deep Learning : introducción práctica con Keras y TensorFlow 2. Marcombo, 2020. [Online]. https://books.google.com.co/books?id=5vpmzQEACAAJ | spa |
dc.relation.references | X. Guo, Y. Yang, C. Wang, and J. Ma, “Image dehazing via enhancement, restoration, and fusion: A survey,” Information Fusion, vol. 86–87, pp. 146–170, 2022, doi: https://doi.org/10.1016/j.inffus.2022.07.005. | spa |
dc.rights | Atribución-NoComercial-SinDerivadas 2.5 Colombia | * |
dc.rights.accessrights | info:eu-repo/semantics/openAccess | |
dc.rights.coar | http://purl.org/coar/access_right/c_abf2 | spa |
dc.rights.local | Abierto (Texto Completo) | spa |
dc.rights.uri | http://creativecommons.org/licenses/by-nc-nd/2.5/co/ | * |
dc.subject.keyword | Artificial Intelligence | spa |
dc.subject.keyword | Classification | spa |
dc.subject.keyword | YOLO | spa |
dc.subject.keyword | Maturity detection | spa |
dc.subject.proposal | Inteligencia Artificial | spa |
dc.subject.proposal | Clasificación | spa |
dc.subject.proposal | YOLO | spa |
dc.subject.proposal | Detección de madurez | spa |
dc.title | Clasificador de Fresa Usando Técnicas de Inteligencia Artificial | spa |
dc.type.coar | http://purl.org/coar/resource_type/c_7a1f | |
dc.type.coarversion | http://purl.org/coar/version/c_ab4af688f83e57aa | |
dc.type.drive | info:eu-repo/semantics/bachelorThesis | |
dc.type.local | Trabajo de grado | spa |
dc.type.version | info:eu-repo/semantics/acceptedVersion |
Archivos
Bloque original
1 - 3 de 3
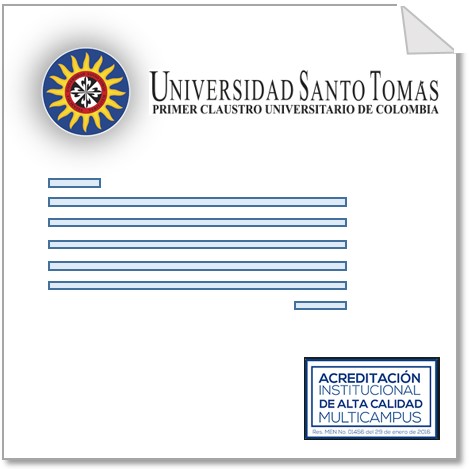
- Nombre:
- 2024cartaderechosdeautor.pdf
- Tamaño:
- 222.84 KB
- Formato:
- Adobe Portable Document Format
- Descripción:
Cargando...
- Nombre:
- 2024juancamargo.pdf
- Tamaño:
- 1.82 MB
- Formato:
- Adobe Portable Document Format
- Descripción:
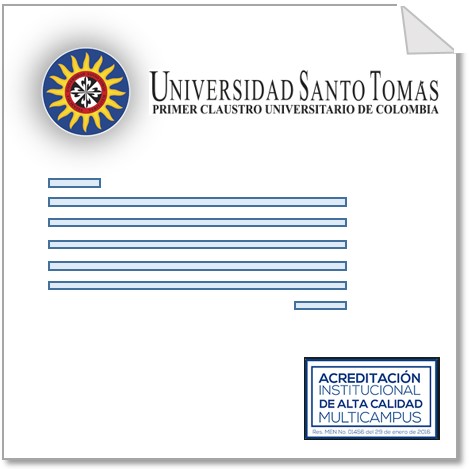
- Nombre:
- APROBACIÓN TRABAJOS DE GRADO CRAI2.pdf
- Tamaño:
- 376.97 KB
- Formato:
- Adobe Portable Document Format
- Descripción:
Bloque de licencias
1 - 1 de 1
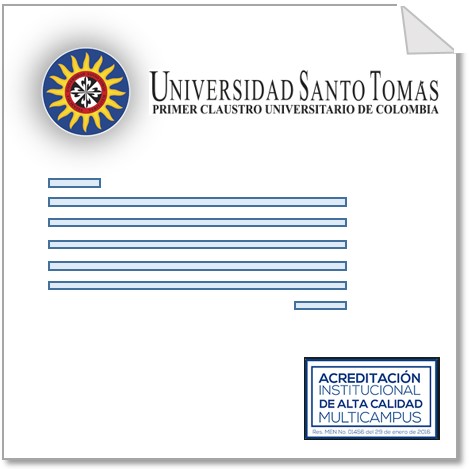
- Nombre:
- license.txt
- Tamaño:
- 807 B
- Formato:
- Item-specific license agreed upon to submission
- Descripción: