Sistema de Navegación en Vehículos Autónomos a Partir del Control Predictivo por Modelo en un Escenario con Obstáculos
dc.contributor.advisor | Calderon Chávez, Juan Manuel | |
dc.contributor.advisor | Amaya, Sindy Paola | |
dc.contributor.author | Corredor Cely, Jorge Luis | |
dc.contributor.author | Garcia Carrillo, Juan Camilo | |
dc.contributor.corporatename | Universidad Santo Tomás | spa |
dc.contributor.cvlac | https://scienti.minciencias.gov.co/cvlac/visualizador/generarCurriculoCv.do?cod_rh=0000380938 | spa |
dc.contributor.cvlac | https://scienti.minciencias.gov.co/cvlac/visualizador/generarCurriculoCv.do?cod_rh=0000796425 | spa |
dc.contributor.cvlac | https://scienti.minciencias.gov.co/cvlac/visualizador/generarCurriculoCv.do?cod_rh=0001767648 | spa |
dc.contributor.cvlac | https://scienti.minciencias.gov.co/cvlac/visualizador/generarCurriculoCv.do?cod_rh=0001767652 | spa |
dc.contributor.googlescholar | https://scholar.google.com/citations?hl=es&user=Gg2sofAAAAAJ | spa |
dc.contributor.orcid | https://orcid.org/0000-0002-4471-3980 | spa |
dc.contributor.orcid | https://orcid.org/0000-0002-1714-1593 | spa |
dc.contributor.orcid | https://orcid.org/0000-0002-3210-6833 | spa |
dc.coverage.campus | CRAI-USTA Bogotá | spa |
dc.date.accessioned | 2024-04-11T17:04:50Z | |
dc.date.available | 2024-04-11T17:04:50Z | |
dc.date.issued | 2023 | |
dc.description | El presente proyecto de grado se enfoca en el diseño de un sistema de navegación para un vehículo autónomo utilizando el enfoque de Control predictivo por modelo en un ambiente de simulación. El MPC es una técnica de control avanzada que utiliza un modelo matemático del sistema y un horizonte de predicción para calcular y aplicar de manera óptima las acciones de control. El objetivo principal de este proyecto es diseñar y evaluar un sistema de navegación utilizando MPC en un entorno simulado para un vehículo autónomo. Si bien la implementación en el mundo real conlleva desafíos prácticos adicionales, la simulación ofrece un entorno seguro y controlado para probar y perfeccionar el sistema antes de considerar su implementación práctica. El sistema de navegación propuesto se basará en una combinación de sensores avanzados proveídos por el ambiente integrado de simulación, como cámaras, LiDAR y radar, para obtener información precisa sobre el entorno circundante. Esta información se fusionará y procesará utilizando algoritmos de percepción y localización, permitiendo al sistema construir un modelo dinámico del entorno y tomar decisiones informadas en función de los objetivos y las restricciones definidas. El diseño del sistema de navegación se llevará a cabo utilizando herramientas y lenguajes de programación adecuados. Se considerarán aspectos de seguridad, rendimiento y eficiencia para garantizar la viabilidad y la aplicabilidad práctica del sistema, incluso en un ambiente simulado. | spa |
dc.description.degreelevel | Pregrado | spa |
dc.description.degreename | Ingeniero Electronico | spa |
dc.format.mimetype | application/pdf | spa |
dc.identifier.citation | Corredor Cely, J. L. y García Carrillo, J. C. (2023). Sistema de Navegación en Vehículos Autónomos a Partir del Control Predictivo por Modelo en un Escenario con Obstáculos. [Trabajo de Grado, Universidad Santo Tomás]. Repositorio Institucional. | spa |
dc.identifier.instname | instname:Universidad Santo Tomás | spa |
dc.identifier.reponame | reponame:Repositorio Institucional Universidad Santo Tomás | spa |
dc.identifier.repourl | repourl:https://repository.usta.edu.co | spa |
dc.identifier.uri | http://hdl.handle.net/11634/54539 | |
dc.language.iso | spa | spa |
dc.publisher | Universidad Santo Tomás | spa |
dc.publisher.faculty | Facultad de Ingeniería Electrónica | spa |
dc.publisher.program | Pregrado Ingeniería Electrónica | spa |
dc.relation.references | Zhang, H., & Braun, S. (2017). Signal processing and control challenges for smart vehicles. Mechanical Systems And Signal Processing, 87, 1-3. https://doi.org/10.1016/j.ymssp.2016.11.016 | spa |
dc.relation.references | Toquica Giraldo, E, Pérez Mojica, F y Rincón Figueroa, M. (2020). La seguridad vial en Bogotá D.C. desde la teoría de la organización y administración pública periodo 2010 – 2019. Universidad Santo tomás. | spa |
dc.relation.references | Guerrero-ibanez, J. y Zeadally, S. (2015). Integration challenges of intelligent transportation systems with connected vehicle, cloud computing, and internet of things technologies. in IEEE Wireless Communications, vol. 22, no. 6, pp. 122-128. https://doi.org/10.1109/MWC.2015.7368833. | spa |
dc.relation.references | Yoon, J., Cho, W., Yi, K., & Koo, B. (2008). Unified Chassis Control for Vehicle Rollover Prevention. IFAC Proceedings Volumes, 41(2), 5682-5687. https://doi.org/10.3182/20080706-5-kr-1001.00958 | spa |
dc.relation.references | Katrakazas, C., Quddus, M., & Deka, L. (2015). Real-time motion planning methods for autonomous on-road driving: State-of-the-art and future research directions. Transportation Research Part C: Emerging Technologies, 60, 416-442. https://doi.org/10.1016/j.trc.2015.09.011 | spa |
dc.relation.references | Shen, C., Shi, Y., & Buckham, B. (2016, 22 septiembre). Integrated Path Planning and Tracking Control of an AUV: A Unified Receding Horizon Optimization Approach. IEEE Journals & Magazine | IEEE Xplore. https://ieeexplore.ieee.org/abstract/document/7574368 | spa |
dc.relation.references | Bulsara, A., Raman, A., Kamarajugadda, S., Schmid, M., & Krovi, V. (2020). Obstacle Avoidance Using Model Predictive Control: An Implementation and Validation Study Using Scaled Vehicles. SAE Technical Paper Series. https://doi.org/10.4271/2020-01-0109 | spa |
dc.relation.references | Hussain, R., & Zeadally, S. (2018). Autonomous cars: Research results, issues, and future challenges. IEEE Communications Surveys & Tutorials, 21(2), 1275-1313. | spa |
dc.relation.references | Yu-Geng, X., De-Wei, L., & Shu, L. (2013). Model predictive control—status and challenges. Acta Automatica Sinica, 39(3), 222-236. | spa |
dc.relation.references | Damtew, A., Erega, Y., Ebrahim, H., Tsegaye, S., & Msigie, D. (2018). The effect of long distance transportation stress on cattle: a review. Biomedical Journal, 2(5). | spa |
dc.relation.references | Claypool, H., Bin-Nun, A., & Gerlach, J. (2017). Self-driving cars: The impact on people with disabilities. Newton, MA: Ruderman Family Foundation. | spa |
dc.relation.references | Worland, J. (2016, 8 septiembre). Self-Driving Cars Could Help Save the Environment—Or Ruin It. It Depends on Us. TIME. https://time.com/4476614/self-driving-cars-environment/ | spa |
dc.relation.references | Ahmadi, B. (2021). Design a Reliable Model Predictive Control for Path Following with Application to the Autonomous Vehicle and Considering Different Vehicle Models (Master's thesis, University of Waterloo). | spa |
dc.relation.references | Qian, X. (2016). Model predictive control for autonomous and cooperative driving (Doctoral dissertation, Université Paris sciences et lettres). | spa |
dc.relation.references | De La Fortelle, A., Qian, X., Diemer, S., Grégoire, J., Moutarde, F., Bonnabel, S., ... & Sjöberg, K. (2014, September). Network of automated vehicles: the AutoNet 2030 vision. In ITS World Congress. | spa |
dc.relation.references | Ziegler, J., Bender, P., Schreiber, M., Lategahn, H., Strauss, T., Stiller, C., ... & Zeeb, E. (2014). Making bertha drive—an autonomous journey on a historic route. IEEE Intelligent transportation systems magazine, 6(2), 8-20. | spa |
dc.relation.references | Piatkowski, D. P., Pitla, S., Luck, J. D., & Hazelton, J. K. (2020). Preparing for a Driverless Future (No. MO50). Nebraska. Department of Transportation. | spa |
dc.relation.references | Ziegler, J., Bender, P., Schreiber, M., Lategahn, H., Strauss, T., Stiller, C., ... & Zeeb, E. (2014). Making bertha drive—an autonomous journey on a historic route. IEEE Intelligent transportation systems magazine, 6(2), 8-20. | spa |
dc.relation.references | Lima, P. F. (2018). Optimization-based motion planning and model predictive control for autonomous driving: With experimental evaluation on a heavy-duty construction truck (Doctoral dissertation, KTH Royal Institute of Technology). | spa |
dc.relation.references | Rosolia, U., Carvalho, A., & Borrelli, F. (2017, May). Autonomous racing using learning model predictive control. In 2017 American control conference (ACC) (pp. 5115-5120). IEEE. | spa |
dc.relation.references | Bimbraw, K. (2015, July). Autonomous cars: Past, present and future a review of the developments in the last century, the present scenario and the expected future of autonomous vehicle technology. In 2015 12th international conference on informatics in control, automation and robotics (ICINCO) (Vol. 1, pp. 191-198). IEEE. | spa |
dc.relation.references | Brockett, R. W. (1983). Asymptotic stability and feedback stabilization. Differential geometric control theory, 27(1), 181-191. | spa |
dc.relation.references | Andersen, H., Schwarting, W., Naser, F., Eng, Y. H., Ang, M. H., Rus, D., & Alonso-Mora, J. (2017, October). Trajectory optimization for autonomous overtaking with visibility maximization. In 2017 IEEE 20th International Conference on Intelligent Transportation Systems (ITSC) (pp. 1-8). IEEE. | spa |
dc.relation.references | Agrawal, M. (2020, 12 agosto). What is Model Predictive Control (MPC)? Technical Articles. https://control.com/technical-articles/what-is-model-predictive-control-mpc/ | spa |
dc.relation.references | Ji, J., Khajepour, A., Melek, W. W., & Huang, Y. (2016). Path planning and tracking for vehicle collision avoidance based on model predictive control with multiconstraints. IEEE Transactions on Vehicular Technology, 66(2), 952-964. | spa |
dc.relation.references | Du, X., Htet, K. K. K., & Tan, K. K. (2016). Development of a genetic-algorithm-based nonlinear model predictive control scheme on velocity and steering of autonomous vehicles. IEEE Transactions on Industrial Electronics, 63(11), 6970-6977. | spa |
dc.relation.references | Lima, P. F., Nilsson, M., Trincavelli, M., Mårtensson, J., & Wahlberg, B. (2017). Spatial model predictive control for smooth and accurate steering of an autonomous truck. IEEE Transactions on Intelligent Vehicles, 2(4), 238-250. | spa |
dc.relation.references | Liu, J., Jayakumar, P., Stein, J. L., & Ersal, T. (2017). Combined speed and steering control in high-speed autonomous ground vehicles for obstacle avoidance using model predictive control. IEEE Transactions on Vehicular Technology, 66(10), 8746-8763. | spa |
dc.relation.references | Andersen, H., Schwarting, W., Naser, F., Eng, Y. H., Ang, M. H., Rus, D., & Alonso-Mora, J. (2017, October). Trajectory optimization for autonomous overtaking with visibility maximization. In 2017 IEEE 20th International Conference on Intelligent Transportation Systems (ITSC) (pp. 1-8). IEEE. | spa |
dc.relation.references | Choi, Y., Lee, W., Kim, J., & Yoo, J. (2021). A variable-sampling time model predictive control algorithm for improving path-tracking performance of a vehicle. Sensors, 21(20), 6845. | spa |
dc.relation.references | Boyd, S. P., & Vandenberghe, L. (2004). Convex optimization. Cambridge university press. | spa |
dc.relation.references | Leyffer, S., & Mahajan, A. (2010). Nonlinear constrained optimization: methods and software. Argonee National Laboratory, Argonne, Illinois, 60439. | spa |
dc.relation.references | IPOPT: Documentation. (s. f.). https://coin-or.github.io/Ipopt/ | spa |
dc.relation.references | Koren, Y., & Borenstein, J. (1991, April). Potential field methods and their inherent limitations for mobile robot navigation. In Icra (Vol. 2, No. 1991, pp. 1398-1404). | spa |
dc.relation.references | ArmyTanker. (s. f.). Slash 4X4 VXL: 1/10 Scale 4WD Electric Short Course Truck with TQiTM Traxxas LinkTM Enabled 2.4GHz Radio System & Traxxas Stability Management (TSM)®. Traxxas.com. https://traxxas.com/products/models/electric/slash-4x4-tsm?t=specs | spa |
dc.relation.references | Resource & Documentation Center. (s. f.). Intel. https://www.intel.com/content/www/us/en/resources-documentation/developer.html#gs.77uyt8 | spa |
dc.relation.references | VESC 6 MkVI PCB - The next generation - Benjamin Vedder Electronic Speed Controller. (s. f.). VESC 6 MkV - The Next Generation Benjamin Vedder Electronic Speed Controller. https://trampaboards.com/vesc-6-mkv--the-next-generation--benjamin-vedder-electronic-speed-controller-p-27517.html | spa |
dc.relation.references | ntel® NUC Products NUC11PA[x]i3/NUC11PA[x]i5/ NUC11PA[x]i7 Technical Product Specification Regulatory Models: NUC11PAK (Slim Kit/Mini PC) NUC11PAH (Tall Ki- t/Mini PC) NUC11PAQ (Kit/Mini PC Wireless Charging). (2022). | spa |
dc.rights | Atribución-NoComercial-SinDerivadas 2.5 Colombia | * |
dc.rights.accessrights | info:eu-repo/semantics/openAccess | |
dc.rights.coar | http://purl.org/coar/access_right/c_abf2 | spa |
dc.rights.local | Abierto (Texto Completo) | spa |
dc.rights.uri | http://creativecommons.org/licenses/by-nc-nd/2.5/co/ | * |
dc.subject.keyword | Model predictive control | spa |
dc.subject.keyword | FGM | spa |
dc.subject.keyword | Path planning | spa |
dc.subject.keyword | Autonomous vehicles | spa |
dc.subject.lemb | Ingeniería Electrónica | spa |
dc.subject.lemb | Diseño, Navegación | spa |
dc.subject.lemb | Vehículo Autónomo | spa |
dc.subject.proposal | Control predictivo por modelo | spa |
dc.subject.proposal | FGM | spa |
dc.subject.proposal | Planificación de rutas | spa |
dc.subject.proposal | Vehículos autónomos | spa |
dc.title | Sistema de Navegación en Vehículos Autónomos a Partir del Control Predictivo por Modelo en un Escenario con Obstáculos | spa |
dc.type.coar | http://purl.org/coar/resource_type/c_7a1f | |
dc.type.coarversion | http://purl.org/coar/version/c_ab4af688f83e57aa | |
dc.type.drive | info:eu-repo/semantics/bachelorThesis | |
dc.type.local | Trabajo de grado | spa |
dc.type.version | info:eu-repo/semantics/acceptedVersion |
Archivos
Bloque original
1 - 3 de 3
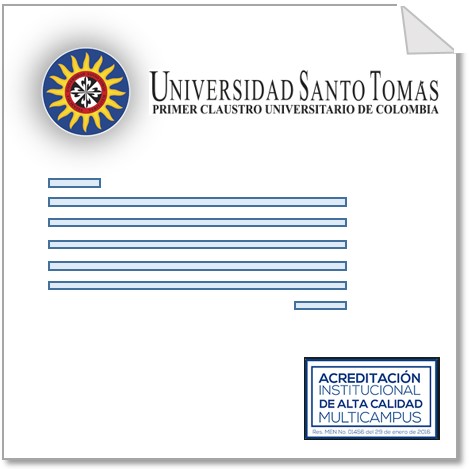
- Nombre:
- 2024cartadefacultad.pdf
- Tamaño:
- 160.36 KB
- Formato:
- Adobe Portable Document Format
- Descripción:
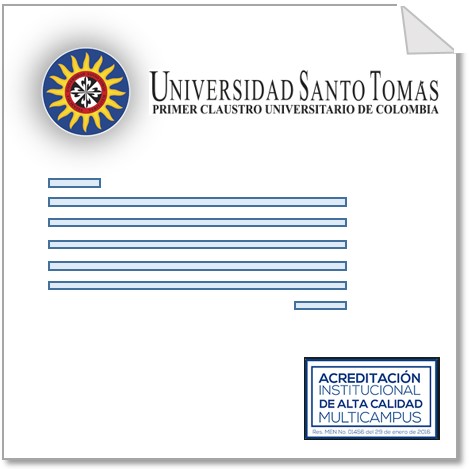
- Nombre:
- 2024cartaderechosdeautor.pdf
- Tamaño:
- 393.96 KB
- Formato:
- Adobe Portable Document Format
- Descripción:
Cargando...
- Nombre:
- 2024jorgecorredor.pdf
- Tamaño:
- 7.55 MB
- Formato:
- Adobe Portable Document Format
- Descripción:
Bloque de licencias
1 - 1 de 1
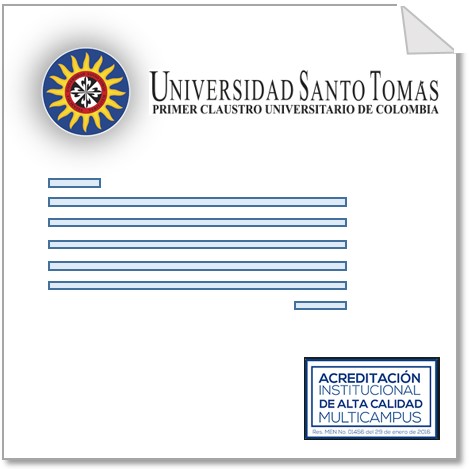
- Nombre:
- license.txt
- Tamaño:
- 807 B
- Formato:
- Item-specific license agreed upon to submission
- Descripción: