On the use of neuroevolutive methods as support tools for diagnosing appendicitis and tuberculosis
dc.contributor.author | Orjuela-Cañón, Alvaro David | spa |
dc.contributor.author | Posada-Quintero, Hugo Fernando | spa |
dc.contributor.author | Cesar Hernando, Valencia | spa |
dc.contributor.author | Mendoza, Leonardo | spa |
dc.coverage.campus | CRAI-USTA Bogotá | spa |
dc.date.accessioned | 2019-08-06T17:48:45Z | spa |
dc.date.available | 2019-08-06T17:48:45Z | spa |
dc.date.issued | 2018-09-13 | spa |
dc.description.abstract | Artificial neural networks are being used in diagnosis support systems to detect different kind of diseases. As the design of multilayer perceptron is an open question, the present work shows a comparison between a traditional empirical way and neuroevolution method to find the best architecture to solve the disease detection problem. Tuberculosis and appendicitis databases were employed to test both proposals. Results show that neuroevolution offers a good alternative for the tuberculosis problem but there is lacks of performance in the appendicitis one. | spa |
dc.description.domain | http://unidadinvestigacion.usta.edu.co | spa |
dc.format.mimetype | application/pdf | spa |
dc.identifier.doi | https://doi.org/10.1007/978-3-030-00350-0_15 | spa |
dc.identifier.uri | http://hdl.handle.net/11634/17997 | |
dc.relation.references | Kalantari, A., Kamsin, A., Shamshirband, S., Gani, A., Alinejad-Rokny, H., Chronopoulos, A.T.: Computational intelligence approaches for classification of medical data: state-of-theart, future challenges and research directions. Neurocomputing 276, 2–22 (2017) | spa |
dc.relation.references | Amato, F., López, A., Peña-Méndez, E.M., Vavnhara, P., Hampl, A., Havel, J.: Artificial neural networks in medical diagnosis (2013) | spa |
dc.relation.references | Seera, M., Lim, C.P.: A hybrid intelligent system for medical data classification. Expert Syst. Appl. 41, 2239–2249 (2014) | spa |
dc.relation.references | Prabhudesai, S.G., Gould, S., Rekhraj, S., Tekkis, P.P., Glazer, G., Ziprin, P.: Artificial neural networks: useful aid in diagnosing acute appendicitis. World J. Surg. 32, 305–309 (2008) | spa |
dc.relation.references | Huang, R., Lee, S., Lai, C., Hsiao, Y., Ting, H.: Acute effects of obstructive sleep apnea on autonomic nervous system, arterial stiffness and heart rate in newly diagnosed untreated patients. Sleep Med. 14, e27 (2013) | spa |
dc.relation.references | Ting, H.-W., Wu, J.-T., Chan, C.-L., Lin, S.-L., Chen, M.-H.: Decision model for acute appendicitis treatment with decision tree technology - a modification of the alvarado scoring system. J. Chin. Med. Assoc. 73, 401–406 (2010) | spa |
dc.relation.references | Park, S.Y., Kim, S.M.: Acute appendicitis diagnosis using artificial neural networks. Technol. Health Care 23, S559–S565 (2015) | spa |
dc.relation.references | Yoldaş, Ö., Tez, M., Karaca, T.: Artificial neural networks in the diagnosis of acute appendicitis. Am. J. Emerg. Med. 30, 1245–1247 (2012) | spa |
dc.relation.references | Santos, A.M., Pereira, B.B., Seixas, J.M., Mello, F.C.Q., Kritski, A.L.: Neural networks: an application for predicting smear negative pulmonary tuberculosis. In: Auget, J.L., Balakrishnan, N., Mesbah, M., Molenberghs, G. (eds.) Advances in Statistical Methods for the Health Sciences, pp. 275–287. Springer, Boston (2007) | spa |
dc.relation.references | El-Solh, A.A., Hsiao, C.-B., Goodnough, S., Serghani, J., Grant, B.J.B.: Predicting active pulmonary tuberculosis using an artificial neural network. Chest J. 116, 968–973 (1999) | spa |
dc.relation.references | Elveren, E., Yumuvak, N.: Tuberculosis disease diagnosis using artificial neural network trained with genetic algorithm. J. Med. Syst. 35, 329–332 (2011) | spa |
dc.relation.references | Er, O., Temurtas, F., Tanrikulu, A.Ç.: Tuberculosis disease diagnosis using artificial neural networks. J. Med. Syst. 34, 299–302 (2010) | spa |
dc.relation.references | Stanley, K.O., Miikkulainen, R.: Evolving neural networks through augmenting topologies. Evol. Comput. 10, 99–127 (2002) | spa |
dc.relation.references | Floreano, D., Dürr, P., Mattiussi, C.: Neuroevolution: from architectures to learning. Evol. Intell. 1, 47–62 (2008) | spa |
dc.relation.references | Miikkulainen, R.: Neuroevolution. In: Encyclopedia of Machine Learning. Springer, New York (2010) | spa |
dc.relation.references | Weiss, S.M., Kapouleas, I.: An empirical comparison of pattern recognition, neural nets and machine learning classification methods. Read. Mach. Learn. 177–183 (1990) | spa |
dc.relation.references | Kohavi, R.: A Study of Cross-Validation and Bootstrap for Accuracy Estimation and Model Selection. Presented at the (1995) | spa |
dc.relation.references | Haykin, S.: Neural Networks and Learning Machines. Prentice Hall, Upper Saddle River (2009) | spa |
dc.relation.references | Riedmiller, M., Braun, H.: A direct adaptive method for faster backpropagation learning: the RPROP algorithm. In: 1993 IEEE International Conference on Neural Networks, pp. 586– 591 (1993) | spa |
dc.relation.references | Beale, M.H., Hagan, M.T., Demuth, H.B.: Neural network toolbox getting started guide R2011b (2011) | spa |
dc.rights | Atribución-NoComercial-CompartirIgual 2.5 Colombia | * |
dc.rights.uri | http://creativecommons.org/licenses/by-nc-sa/2.5/co/ | * |
dc.subject.keyword | Neuroevolution | spa |
dc.subject.keyword | Artificial neural networks | spa |
dc.subject.keyword | Diagnosis support systems | spa |
dc.subject.keyword | Tuberculosis | spa |
dc.subject.keyword | Appendicitis | spa |
dc.title | On the use of neuroevolutive methods as support tools for diagnosing appendicitis and tuberculosis | spa |
dc.type.category | Generación de Nuevo Conocimiento: Artículos publicados en revistas especializadas - Electrónicos | spa |
Archivos
Bloque original
1 - 1 de 1
Cargando...
- Nombre:
- On the use of neuroevolutive methods as support tools for diagnosing appendicitis and tuberculosis.pdf
- Tamaño:
- 973.56 KB
- Formato:
- Adobe Portable Document Format
- Descripción:
- Artículo SCOPUS
Bloque de licencias
1 - 1 de 1
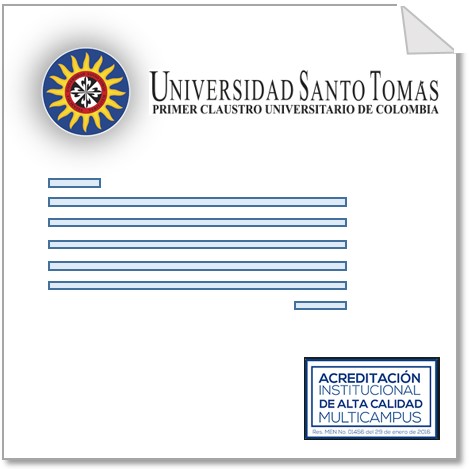
- Nombre:
- license.txt
- Tamaño:
- 807 B
- Formato:
- Item-specific license agreed upon to submission
- Descripción: