Aprendizaje Profundo para la Detección de Caídas en Personas Vulnerables
dc.contributor.advisor | Cruz Capador, Gerson David | |
dc.contributor.advisor | Guarnizo Marín, José Guillermo | |
dc.contributor.author | Saa Beltrán, María Paula | |
dc.contributor.corporatename | Universidad Santo Tomás | spa |
dc.contributor.cvlac | https://scienti.minciencias.gov.co/cvlac/visualizador/generarCurriculoCv.do?cod_rh=0001334709 | spa |
dc.contributor.cvlac | https://scienti.minciencias.gov.co/cvlac/visualizador/generarCurriculoCv.do?cod_rh=0000855847 | spa |
dc.contributor.cvlac | https://scienti.minciencias.gov.co/cvlac/visualizador/generarCurriculoCv.do?cod_rh=0001767650 | spa |
dc.contributor.googlescholar | https://scholar.google.com/citations?hl=es&user=fVo6U9MAAAAJ | spa |
dc.contributor.googlescholar | https://scholar.google.com/citations?hl=es&user=3JSJ0C4AAAAJ | spa |
dc.contributor.orcid | https://orcid.org/0000-0002-3723-7509 | spa |
dc.contributor.orcid | https://orcid.org/0000-0002-8401-4949 | spa |
dc.contributor.orcid | https://orcid.org/0000-0001-8509-2378 | spa |
dc.coverage.campus | CRAI-USTA Bogotá | spa |
dc.date.accessioned | 2023-04-19T16:31:33Z | |
dc.date.available | 2023-04-19T16:31:33Z | |
dc.date.issued | 2023-04-17 | |
dc.description | El proyecto de tesis, muestra el diseño, implementación y desarrollo de un algoritmo de aprendizaje profundo que permite realizar detección de caídas en especial en personal mayores que se encuentran viviendo solas, en espacios médicos o en centros de cuidados geriátricos. Se busca que este proyecto pueda realizar esta detección, sin la necesidad de emplear dispositivos corporales que pueden generar inconvenientes en los pacientes, razón por la cual se opta por emplear un algoritmo basado en redes neuronales recurrentes de tipo LSTM (Memoria prolongada de corto plazo), que tienen la capacidad de recordar información relevante en secuencias y preservarlo por varios instantes de tiempo. Se realizan las pruebas en ambientes controlados, junto con personas que emulen las caídas y que no cuenten con ningún inconveniente de salud, la evaluación del proyectó se realiza a través de distintas métricas y pruebas en tiempo real. | spa |
dc.description.abstract | The undergraduate project shows the design, implementation and development of a deep learning algorithm that allows fall detection, especially in older people who are living alone, in medical centres or in geriatric care centres. It is sought that this project can carry out this detection, without the need to use body devices that can generate inconveniences in patients, that is why it is chosen to use an algorithm based on recurrent neural networks of the LSTM type (Long short-term memory), that have the ability to remember relevant information in sequences and preserve it for several instants of time. The tests are carried out in controlled environments, with people who emulate the falls and do not have any health issues, the evaluation of the project is carried out through different metrics and tests in real time. | spa |
dc.description.degreelevel | Pregrado | spa |
dc.description.degreename | Ingeniero Electronico | spa |
dc.format.mimetype | application/pdf | spa |
dc.identifier.citation | Saa Beltrán, M. P. (2023). Aprendizaje Profundo para la Detección de Caídas en Personas Vulnerables. [Trabajo de Grado, Universidad Santo Tomás]. Repositorio Institucional. | spa |
dc.identifier.instname | instname:Universidad Santo Tomás | spa |
dc.identifier.reponame | reponame:Repositorio Institucional Universidad Santo Tomás | spa |
dc.identifier.repourl | repourl:https://repository.usta.edu.co | spa |
dc.identifier.uri | http://hdl.handle.net/11634/50378 | |
dc.language.iso | spa | spa |
dc.publisher | Universidad Santo Tomás | spa |
dc.publisher.faculty | Facultad de Ingeniería Electrónica | spa |
dc.publisher.program | Pregrado Ingeniería Electrónica | spa |
dc.relation.references | Ahmed Abobakr, Mohammed Hossny, Hala Abdelkader, and Saeid Nahavandi. Rgb-d fall detection via deep residual convolutional lstm networks. In 2018 Digital Image Computing: Techniques and Applications (DICTA), pages 1–7, 2018. | spa |
dc.relation.references | Charu C Aggarwal et al. Neural networks and deep learning. Springer, 10:978–3, 2018. | spa |
dc.relation.references | Xavier Basogain. Redes neuronales artificiales y sus aplicaciones. Dpto. Ingeniería de Sistemas y Automática, Escuela Superior de Ingeniería Bilbao, howpublished=http://ocw.ehu.es/ensenanzas-tecnicas/ redes-neuronales-artificiales-y-sus-aplicaciones/Course_ listing., year=2008. | spa |
dc.relation.references | Valentin Bazarevsky and Google Research Ivan Grishchenko, Research Engineers. On-device, real-time body pose tracking with mediapipe blazepose. https://ai.googleblog.com/2020/08/on-device-real-time-body-pose-tracking.html, aug 2020. | spa |
dc.relation.references | Avijeet Biswal. Convolutional neural network tutorial. https://www.simplilearn.com/tutorials/deep-learning-tutorial/convolutional-neural-network, jul 2022. | spa |
dc.relation.references | Avijeet Biswal. Recurrent neural network (rnn) tutorial: Types, examples, lstm and more. https://www.simplilearn.com/tutorials/deep-learning-tutorial/rnn, aug 2022. | spa |
dc.relation.references | Sebastian Bittrich, Marika Kaden, Christoph Leberecht, Florian Kaiser, Thomas Villmann, and Dirk Labudde. Application of an interpretable classification model on early folding residues during protein folding. BioData mining, 12(1):1–16, 2019. | spa |
dc.relation.references | Harsha Bommana. Introduction to neural networks — part 1. https://medium.com/deep-learning-demystified/introduction-to-neural-networks-part-1-e13f132c6d7e, may 2019. | spa |
dc.relation.references | Kabalan Chaccour, Rony Darazi, Amir Hajjam El Hassani, and Emmanuel Andrès. From fall detection to fall prevention: A generic classification of fall-related systems. IEEE Sensors Journal, 17(3):812–822, 2017. | spa |
dc.relation.references | Madhav Chamle, K. G. Gunale, and K. K. Warhade. Automated unusual event detection in video surveillance. In 2016 International Conference on Inventive Computation Technologies (ICICT), volume 2, pages 1–4, 2016. | spa |
dc.relation.references | Pongsatorn Chutimawattanakul and Pranchalee Samanpiboon. Fall detection for the elderly using yolov4 and lstm. In 2022 19th International Conference on Electrical Engineering/Electronics, Computer, Telecommunications and Information Technology (ECTI-CON), pages 1–5,2022. | spa |
dc.relation.references | Intel Corporation. Open source computer vision. https://docs.opencv.org/4.6.0/, jun 2022. | spa |
dc.relation.references | DANE. Adulto mayor en colombia - características generales. https://www.dane.gov.co/files/investigaciones/genero/presentacion-caracteristicas-generales-adulto-mayor-en-colombia.pdf, jan 2021. | spa |
dc.relation.references | Gobierno de Colombia. Misión de sabios - colombia - 2019. https://minciencias.gov.co/sites/default/files/libro_mision_de_sabios_digital_1_2_0.pdf, dec 2021. | spa |
dc.relation.references | Congreso de la República. Medidas de protección al adulto mayor en colombia. http://www.secretariasenado.gov.co/senado/basedoc/ley_1850_2017.html, jul 2017. | spa |
dc.relation.references | Congreso de la República. ConvenciÓn interamericana sobre la protección de los derechos humanos de las personas mayores. https://www.funcionpublica.gov.co/eva/gestornormativo/norma.php?i=141981, sep 2020. | spa |
dc.relation.references | Sonia Patricia de Santillana Hernández, Luis Eduardo Alvarado Moctezuma, Gustavo Rodrigo Medina Beltrán, Gricelda Gómez Ortega, Rosa María Cortés Gonzálezzuela Espinoza, and Rosa María Cortés González. Caídas en el adulto mayor. factores intrínsecos y extrínsecos. Revista Médica del Instituto Mexicano del Seguro Social, 40(6):489–493, 2002. | spa |
dc.relation.references | Riya Dichwalkar, Shreya Oak, Tania Rajabally, and Dhananjay Kalbande. Activity recognition and fall detection in elderly people. In 2020 11th International Conference on Computing, Communication and Networking Technologies (ICCCNT), pages 1–6, 2020. | spa |
dc.relation.references | Tran Duc Tan and Nguyen Van Tinh. Reliable fall detection system using an 3-dof accelerometer and cascade posture recognitions. In Signal and Information Processing AssociationAnnual Summit and Conference (APSIPA), 2014 Asia-Pacific, pages 1–6, 2014. | spa |
dc.relation.references | Nashwa El-Bendary, Qing Tan, Frédérique C Pivot, and Anthony Lam. Fall detection and prevention for the elderly: A review of trends and challenges. International Journal on Smart Sensing & Intelligent Systems, 6(3), 2013. | spa |
dc.relation.references | National Geografic España. Sinapsis, el lenguaje de las neuronas en el cerebro. https://n9.cl/6vjro9, apr 2019. | spa |
dc.relation.references | H Espínola. Caídas en el adulto mayor. Boletín de la pontificia Universidad Católica de Chile, 29:1–5, 2000. | spa |
dc.relation.references | M.M. Fadoul. Python Machine Learning: Machine Learning and Deep Learning from Scratch Illustrated with Python, Scikit-Learn, Keras, Theano and Tensorflow. Independently Published, 2020. | spa |
dc.relation.references | Yaxiang Fan, Martin D. Levine, Gongjian Wen, and Shaohua Qiu. A deep neural network for real-time detection of falling humans in naturally occurring scenes. Neurocomputing, 260:43–58, 2017. | spa |
dc.relation.references | Aditya Rio Fansdana, Aulia Khamas Heikhmakhtiar, and Satria Mandala. Real-time falling detection system for elderly using cnn. In 2021 International Conference on Data Science and Its Applications (ICoDSA), pages 194–197, 2021. | spa |
dc.relation.references | Shubham Garg, Aman Saxena, and Richa Gupta. Yoga pose classification: a cnn and mediapipe inspired deep learning approach for real-world application. Journal of Ambient Intelligence and Humanized Computing, pages 1–12, 2022. | spa |
dc.relation.references | Sparsh Gupta. The 7 most common machine learning loss functions. https://builtin.com/machine-learning/common-loss-functions, apr 2022. | spa |
dc.relation.references | Khadija Hanifi and M. Elif Karsligil. Elderly fall detection with vital signs monitoring using cw doppler radar. IEEE Sensors Journal, 21(15):16969–16978, 2021. | spa |
dc.relation.references | Md Mahedi Hasan, Md Shamimul Islam, and Sohaib Abdullah. Robust pose-based human fall detection using recurrent neural network. In 2019 IEEE International Conference on Robotics, Automation, Artificial-intelligence and Internet-of-Things (RAAICON), pages 48–51, 2019. | spa |
dc.relation.references | Kyaw Kyaw Htike, Othman O. Khalifa, Huda Adibah Mohd Ramli, and Mohammad A. M. Abushariah. Human activity recognition for video surveillance using sequences of postures. In The Third International Conference on e-Technologies and Networks for Development (ICeND2014), pages 79–82, 2014. | spa |
dc.relation.references | Madoka Inoue, Ryo Taguchi, and Taizo Umezaki. Bed-exit prediction applying neural network combining bed position detection and patient posture estimation. In 2019 41st Annual International Conference of the IEEE Engineering in Medicine and Biology Society (EMBC), pages 3208–3211, 2019. | spa |
dc.relation.references | Shruti Jadon. What is an activation function? what are commonly used activation functions? https://medium.com/@shrutijadon/survey-on-activation-functions-for-deep-learning9689331ba092, mar 2018. | spa |
dc.relation.references | Rose A Kenny, Cliodhna Ni Scanaill, and Michael McGrath. Falls prevention in the home: Challenges for new technologies. In Intelligent Technologies for Bridging the Grey Digital Divide, pages 46–64. IGI Global, 2011. | spa |
dc.relation.references | Sangyeon Kim, Gawon Lee, and Jihie Kim. Lightweight real-time fall detection using bi- directional recurrent neural network. In 2020 Joint 11th International Conference on Soft Computing and Intelligent Systems and 21st International Symposium on Advanced Intelligent Systems (SCIS-ISIS), pages 1–5, 2020. | spa |
dc.relation.references | Günter Klambauer, Thomas Unterthiner, Andreas Mayr, and Sepp Hochreiter. Selfnormalizing neural networks. Advances in neural information processing systems, 30, 2017. | spa |
dc.relation.references | Xuan-Hien Le, Hung Viet Ho, Giha Lee, and Sungho Jung. Application of long short-term memory (lstm) neural network for flood forecasting. Water, 11(7):1387, 2019. | spa |
dc.relation.references | Xiaogang Li, Tiantian Pang, Weixiang Liu, and Tianfu Wang. Fall detection for elderly person care using convolutional neural networks. In 2017 10th International Congress on Image and Signal Processing, BioMedical Engineering and Informatics (CISP-BMEI), pages 1–6, 2017. | spa |
dc.relation.references | Tsung-Yi Lin, Michael Maire, Serge Belongie, Lubomir Bourdev, Ross Girshick, James Hays, Pietro Perona, Deva Ramanan, C. Lawrence Zitnick, and Piotr Dollár. Microsoft coco: Common objects in context, 2014. | spa |
dc.relation.references | Google LLC. Mediapipe pose. https://google.github.io/mediapipe/solutions/pose.html, jun 2020. | spa |
dc.relation.references | Patricio Loncomilla. Deep learning: Redes convolucionales. Recuperado de https://ccc. inaoep. mx/ ̃ pgomez/deep/presentations, 2016. | spa |
dc.relation.references | Steven T Moore, Valentina Dilda, Bandar Hakim, and Hamish G MacDougall. Validation of 24-hour ambulatory gait assessment in parkinson’s disease with simultaneous video observation. Biomedical engineering online, 10(1):1–9, 2011. | spa |
dc.relation.references | Sarah Mroz, Natalie Baddour, Connor McGuirk, Pascale Juneau, Albert Tu, Kevin Cheung, and Edward Lemaire. Comparing the quality of human pose estimation with blazepose or openpose. pages 1–4, 12 2021. | spa |
dc.relation.references | Abdulmajid Murad and Jae-Young Pyun. Deep recurrent neural networks for human activity recognition. Sensors, 17(11):2556, 2017. | spa |
dc.relation.references | Brian Mwandau and Matunda Nyanchama. Investigating Keystroke Dynamics as a Two-Factor Biometric Security. PhD thesis, 06 2018. | spa |
dc.relation.references | Cristobal Olah. Introducción a la memoria a largo corto plazo (lstm). https://colah.github.io/posts/2015-08-Understanding-LSTMs/, aug 2015. | spa |
dc.relation.references | Artem Oppermann. Loss functionsin deep learning. jun 2021. | spa |
dc.relation.references | Jesús Pérez Guerrero. Redes recurrentes. 2020. | spa |
dc.relation.references | M Gestal Pose. Introducción a las redes de neuronas artificiales. Departamento de Tecnologías de la Información y las Comunicaciones. Universidad da Coruña, 2009. | spa |
dc.relation.references | Patryk Radzki. Detection of human body landmarks - mediapipe and openpose comparison. https://www.hearai.pl/post/14-openpose/, apr 2022. | spa |
dc.relation.references | Nicholas Renotte. Action detection for sign language. https://github.com/nicknochnack/ActionDetectionforSignLanguage, jun 2021. | spa |
dc.relation.references | Laura María Álvarez Rodríguez. Síndrome de caídas en el adulto mayor. Revista Médica de Costa Rica y Centroamérica, 72(617):807–810, 2016. | spa |
dc.relation.references | Ravi Kiran Selvam. Adagrad and adadelta optimizers - implementation and testing:-. https://www.sravikiran.com/GSOC18/page2/, jul 2018. | spa |
dc.relation.references | N Senthilkumar, M Manimegalai, S Karpakam, S.R Ashokkumar, and M Premkumar. Human action recognition based on spatial–temporal relational model and lstm-cnn framework. Materials Today: Proceedings, 57:2087–2091, 2022. International Conference on Innovation and Application in Science and Technology. | spa |
dc.relation.references | Sagar Sharma, Simone Sharma, and Anidhya Athaiya. Activation functions in neural networks. towards data science, 6(12):310–316, 2017. | spa |
dc.relation.references | Alex Sherstinsky. Fundamentals of recurrent neural network (rnn) and long short-term memory (lstm) network. Physica D: Nonlinear Phenomena, 404:132306, 2020. | spa |
dc.relation.references | simpl!learn. What is neural network: Overview, applications, and advantages. https://www.simplilearn.com/tutorials/deep-learning-tutorial/what-is-neural-network, jan 2022. | spa |
dc.relation.references | Ruoyu Sun. Optimization for deep learning: theory and algorithms. arXiv preprint ar Xiv:1912.08957, 2019. | spa |
dc.relation.references | M. Taylor. Make Your Own Neural Network: An In-Depth Visual Introduction for Beginners. Amazon Digital Services LLC - KDP Print US, 2017. | spa |
dc.relation.references | tutorialspoint. Evaluating model performance. https://www.tutorialspoint.com/deep_learning_with_keras/deep_learning_with_keras_evaluating_model_performance.htm#, feb 2022. | spa |
dc.relation.references | Pavan Vadapalli. Biological neural network: Importance, components & comparison. https://www.upgrad.com/blog/biological-neural-network/, feb 2021. | spa |
dc.relation.references | S Yan. Understanding lstm networks. Online). Accessed on August, 11, 2015. | spa |
dc.relation.references | Xiaokang Zhou, Wei Liang, Kevin I-Kai Wang, Hao Wang, Laurence T. Yang, and Qun Jin. Deep-learning-enhanced human activity recognition for internet of healthcare things. IEEE Internet of Things Journal, 7(7):6429–6438, 2020. | spa |
dc.rights | Atribución-NoComercial-SinDerivadas 2.5 Colombia | * |
dc.rights.accessrights | info:eu-repo/semantics/openAccess | |
dc.rights.coar | http://purl.org/coar/access_right/c_abf2 | spa |
dc.rights.local | Abierto (Texto Completo) | spa |
dc.rights.uri | http://creativecommons.org/licenses/by-nc-nd/2.5/co/ | * |
dc.subject.keyword | Neural Networks | spa |
dc.subject.keyword | Falls | spa |
dc.subject.keyword | Long-Short Term Memory | spa |
dc.subject.keyword | Deep Learning | spa |
dc.subject.lemb | Ingeniería Electrónica | spa |
dc.subject.lemb | Personas Vulnerables | spa |
dc.subject.lemb | Diseño-Algoritmos | spa |
dc.subject.proposal | Redes Neuronales | spa |
dc.subject.proposal | Caídas | spa |
dc.subject.proposal | Long-Short Term Memory (LSTM) | spa |
dc.subject.proposal | Aprendizaje Profundo | spa |
dc.title | Aprendizaje Profundo para la Detección de Caídas en Personas Vulnerables | spa |
dc.type | bachelor thesis | |
dc.type.coar | http://purl.org/coar/resource_type/c_7a1f | |
dc.type.coarversion | http://purl.org/coar/version/c_ab4af688f83e57aa | |
dc.type.drive | info:eu-repo/semantics/bachelorThesis | |
dc.type.local | Tesis de pregrado | spa |
dc.type.version | info:eu-repo/semantics/acceptedVersion |
Archivos
Bloque original
1 - 3 de 3
Cargando...
- Nombre:
- 2023mariapaulasaabeltran.pdf
- Tamaño:
- 5.36 MB
- Formato:
- Adobe Portable Document Format
- Descripción:
- Trabajo de Grado
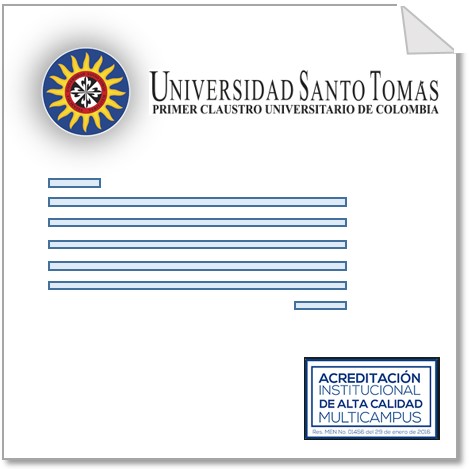
- Nombre:
- Carta_autorizacion_autoarchivo_autor_2023.pdf
- Tamaño:
- 892.39 KB
- Formato:
- Adobe Portable Document Format
- Descripción:
- Carta Derechos de Autor
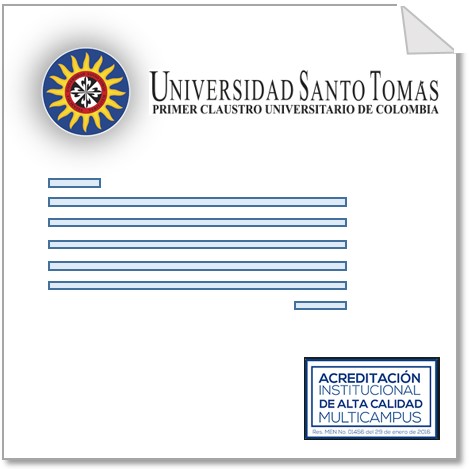
- Nombre:
- CARTA APROBACIÓN FACULTAD MPSB.pdf
- Tamaño:
- 379.4 KB
- Formato:
- Adobe Portable Document Format
- Descripción:
- Cara de Aprobación de la Facultad
Bloque de licencias
1 - 1 de 1
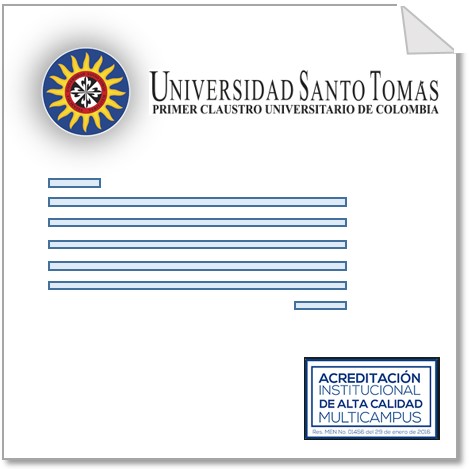
- Nombre:
- license.txt
- Tamaño:
- 807 B
- Formato:
- Item-specific license agreed upon to submission
- Descripción: