Methodology for learning multimodal instructions in the context of human-robot interaction using machine learning
dc.contributor.author | Rodríguez, Saith | spa |
dc.contributor.author | Quintero, Carlos A. | spa |
dc.contributor.author | Pérez, Andrea K. | spa |
dc.contributor.author | Rojas, Eyberth | spa |
dc.contributor.author | Peña, Oswaldo | spa |
dc.contributor.author | De La Rosa, Fernando | spa |
dc.coverage.campus | CRAI-USTA Bogotá | spa |
dc.date.accessioned | 2019-07-08T14:20:42Z | spa |
dc.date.available | 2019-07-08T14:20:42Z | spa |
dc.date.issued | 2018-02-17 | spa |
dc.description.abstract | This work shows the design, implementation and evaluation of a human-robot interaction system where a robot is capable of learning multimodal instructions through gestures and voice issued by a human user. The learning procedure can be performed in two ways: an instruction learning phase, where the human aims at teaching one instruction to the robot by performing several repetitions and an instruction receiving phase where the robot reacts to the instructions given by the human and possibly asks for feedback from the user to strengthen the instruction’s model. | spa |
dc.description.domain | http://unidadinvestigacion.usta.edu.co | spa |
dc.format.mimetype | application/pdf | spa |
dc.identifier.citation | Rodríguez, S., Quintero, C. A., Pérez, A. K., Rojas, E., Peña, O., & De La Rosa, F. (2018). Methodology for learning multimodal instructions in the context of human-robot interaction using machine learning. Bogotá: doi:10.1007/978-3-319-76261-6_4 | spa |
dc.identifier.doi | https://doi.org/10.1007/978-3-319-76261-6_4 | spa |
dc.identifier.uri | http://hdl.handle.net/11634/17504 | |
dc.relation.references | Tee, K.P., Yan, R., Chu, Y.: Gesture-based attention direction for a telepresence robot: design and experimental study. In: Proceedings 2014 IEEE/RSJ International Conference on Intelligent Robots and Systems, IEEE (2014) | spa |
dc.relation.references | Bhattacharya, S., Czejdo, B., Perez, N.: Gesture classification with machine learning using kinect sensor data. In: Third International Conference on Emerging Applications of Information Technology (EAIT) (2012) | spa |
dc.relation.references | Huang, J., Lee, C.W., Ma, J.: Gesture recognition and classification using the microsoft kinect. In: 5th International Conference on Automation, Robotics and Applications, ICARA 2011 (2009) | spa |
dc.relation.references | Zhang, H., Du, W.X., Li, H.: Kinect Gesture Recognition for Interactive System (2009) | spa |
dc.relation.references | Dhanalakshmi, P., Palanivel, S., Ramalingam, V.: Classification of audio signals using SVM and RBFNN. Expert Syst. Appl. 36(3), 6069–6075 (2009) | spa |
dc.relation.references | Sarchaga, G., Sartori, V., Vignoli, L.: Identificacin automtica de resumen en canciones (2006) | spa |
dc.relation.references | Suresh, V., Mohan, C.K., Swamy, R.K., Yegnanarayana, B.: Content-based video classification using support vector machines. In: Pal, N.R., Kasabov, N., Mudi, R.K., Pal, S., Parui, S.K. (eds.) ICONIP 2004. LNCS, vol. 3316, pp. 726–731. Springer, Heidelberg (2004). https://doi.org/10.1007/978-3-540-30499-9 111 | spa |
dc.relation.references | Subashini, K., Palanivel, S., Ramaligam, V.: Audio-video based segmentation and classification using SVM (2012) | spa |
dc.relation.references | Kittler, J., Hatef, M., Duin, R.P.W., Matas, J.: On combining classifiers. IEEE Trans. Pattern Anal. Mach. Intell. 20(3), 226–239 (1998) | spa |
dc.relation.references | Babushkin, V., Oudah, M., Chenlinangjia, T., Alshaer, A., Crandall, J.: Online learning in repeated human-robot interactions. In: Artificial Intelligence for Human-robot Interaction: Papers from the AAAI Fall Symposium, pp. 42–44. AAAI Press (2014 | spa |
dc.relation.references | Molau, S., Pitz, M., Schluter, R., Ney, H.: Computing mel-frequency cepstral coefficients on the power spectrum. In: Proceedings of IEEE International Conference on Acoustics, Speech, and Signal Processing, pp. 73–76. IEEE Press (2001) | spa |
dc.relation.references | Rodriguez, S., P´erez, K., Quintero, C., L´opez, J., Rojas, E., Calder´on, J.: Identification of multimodal human-robot interaction using combined kernels. In: Sn´aˇsel, V., Abraham, A., Kr¨omer, P., Pant, M., Muda, A.K. (eds.) Innovations in Bio-Inspired Computing and Applications. AISC, vol. 424, pp. 263–273. Springer, Cham (2016). https://doi.org/10.1007/978-3-319-28031-8 23 | spa |
dc.relation.references | Hsu, C.W., Chang, C.C., Lin, C.J.: A practical guide to support vector classification. Technical report, Department of Computer Science, National Taiwan University (2003) | spa |
dc.rights | Atribución-NoComercial-CompartirIgual 2.5 Colombia | * |
dc.rights.uri | http://creativecommons.org/licenses/by-nc-sa/2.5/co/ | * |
dc.subject.keyword | Human-robot interaction system | spa |
dc.subject.keyword | Learning multimodal | spa |
dc.subject.keyword | Machine Learning | spa |
dc.title | Methodology for learning multimodal instructions in the context of human-robot interaction using machine learning | spa |
dc.type.category | Generación de Nuevo Conocimiento: Artículos publicados en revistas especializadas - Electrónicos | spa |
Archivos
Bloque original
1 - 1 de 1
Cargando...
- Nombre:
- Methodology for learning multimodal instructions in the context of human-robot interaction using machine learning.pdf
- Tamaño:
- 2.89 MB
- Formato:
- Adobe Portable Document Format
- Descripción:
- Artículo SCOPUS
Bloque de licencias
1 - 1 de 1
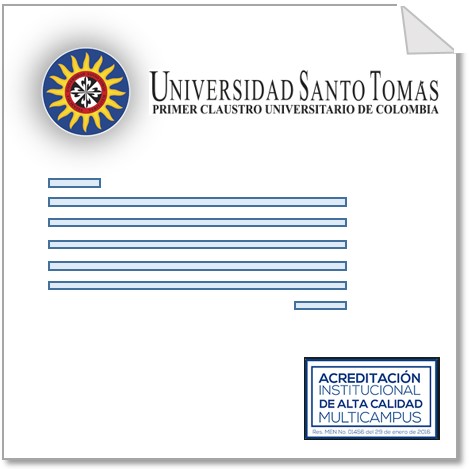
- Nombre:
- license.txt
- Tamaño:
- 807 B
- Formato:
- Item-specific license agreed upon to submission
- Descripción: