Estado del arte sobre metodologías el acotamiento de rondas hídricas.
dc.contributor.advisor | Román Botero, Ricardo | |
dc.contributor.author | Torres Albarracin, Jessica Alejandra | |
dc.contributor.corporatename | Universidad Santo Tomás | spa |
dc.contributor.orcid | https://orcid.org/ 0000-0002-5273-8395 | spa |
dc.coverage.campus | CRAI-USTA Bogotá | spa |
dc.date.accessioned | 2022-07-25T14:22:53Z | |
dc.date.available | 2022-07-25T14:22:53Z | |
dc.date.issued | 2022-07-21 | |
dc.description | La ronda hídrica es conocida como zona riparia o ribereña, región de transición y de interacciones entre los medios terrestre y acuático, es decir, un ecotono. En tal sentido, son las franjas contiguas a los cuerpos de agua naturales continentales, estén en movimiento (ríos, quebradas, arroyos) o relativamente estancados (lagos, lagunas, pantanos, esteros), y el flujo sea continuo, periódico o eventual durante el año hidrológico (Swanson et al., 1988). A partir de ello nace la necesidad de establecer parámetros para su acotamiento, función que deben cumplir las Autoridades Ambientales competentes, teniendo en cuenta los criterios geomorfológicos, hidrológicos y ecosistémicos, establecidos en la Guía Técnica de Criterios para el Acotamiento de las Rondas Hídricas en Colombia, establecida en el Decreto 2245 de 2017. Este estado del arte aborda diferentes metodologías utilizadas para el acotamiento de las rondas hídricas a nivel global y local. | spa |
dc.description.abstract | The watershed is known as a riparian zone, a region of transition and interaction between the terrestrial and aquatic environments, i.e., an ecotone. In this sense, they are the strips contiguous to inland natural water bodies, whether they are in movement (rivers, streams, creeks) or relatively stagnant (lakes, lagoons, marshes, estuaries), and whether the flow is continuous, periodic or eventual during the hydrological year (Swanson et al., 1988). From this arises the need to establish parameters for their delimitation, a function that must be fulfilled by the competent Environmental Authorities, taking into account the geomorphological, hydrological and ecosystemic criteria, established in the Technical Guide of Criteria for the Delimitation of Water Rounds in Colombia, established in Decree 2245 of 2017. This state of the art addresses different methodologies used for the delineation of water courses at the global and local levels. | spa |
dc.description.degreelevel | Pregrado | spa |
dc.description.degreename | Ingeniero Ambiental | spa |
dc.format.mimetype | application/pdf | spa |
dc.identifier.citation | Torres Albarracin, J. A. (2022). Estado del arte sobre metodologías el acotamiento de rondas hídricas. [Trabajo de grado, Universidad Santo Tomás]. Repositorio institucional. | spa |
dc.identifier.instname | instname:Universidad Santo Tomás | spa |
dc.identifier.reponame | reponame:Repositorio Institucional Universidad Santo Tomás | spa |
dc.identifier.repourl | repourl:https://repository.usta.edu.co | spa |
dc.identifier.uri | http://hdl.handle.net/11634/46029 | |
dc.language.iso | spa | spa |
dc.publisher | Universidad Santo Tomás | spa |
dc.publisher.faculty | Facultad de Ingeniería Ambiental | spa |
dc.publisher.program | Pregrado de Ingeniería Ambiental | spa |
dc.relation.references | Adnan, M. S. G., Dewan, A., Zannat, K. E., & Abdullah, A. Y. M. (2019). The use of watershed geomorphic data in flash flood susceptibility zoning: a case study of the Karnaphuli and Sangu River basins of Bangladesh. Natural Hazards, 99(1), 425–448. https://doi.org/10.1007/s11069-019-03749-3 | spa |
dc.relation.references | Alexander, G., & Mendivelso, R. (2020). Determinación de la superficie de inundación del río Bogotá en el sector de la Universidad Militar Nueva Granada - sede campus Cajicá. | spa |
dc.relation.references | Alipour, A., Jafarzadegan, K., & Moradkhani, H. (2022). Global sensitivity analysis in hydrodynamic modeling and flood inundation mapping. Environmental Modelling & Software, 152, 105398. https://doi.org/10.1016/J.ENVSOFT.2022.105398 | spa |
dc.relation.references | Avand, M., Moradi, H., & lasboyee, M. R. (2021a). Using machine learning models, remote sensing, and GIS to investigate the effects of changing climates and land uses on flood probability. Journal of Hydrology, 595, 125663. https://doi.org/10.1016/J.JHYDROL.2020.125663 | spa |
dc.relation.references | Avand, M., Moradi, H., & lasboyee, M. R. (2021b). Spatial modeling of flood probability using geo-environmental variables and machine learning models, case study: Tajan watershed, Iran. Advances in Space Research, 67(10), 3169–3186. https://doi.org/10.1016/J.ASR.2021.02.011 | spa |
dc.relation.references | Berghuijs, W. R., Woods, R. A., Hutton, C. J., & Sivapalan, M. (2016). Dominant flood generating mechanisms across the United States. Geophysical Research Letters, 43(9), 4382–4390. https://doi.org/10.1002/2016GL068070 | spa |
dc.relation.references | Bhatt, S., & Ahmed, S. A. (2014). Morphometric analysis to determine floods in the Upper Krishna basin using Cartosat DEM. Geocarto International, 29(8), 878–894. https://doi.org/10.1080/10106049.2013.868042 | spa |
dc.relation.references | Bhuiyan, S. R., & Baky, A. al. (2014). Digital elevation based flood hazard and vulnerability study at various return periods in Sirajganj Sadar Upazila, Bangladesh. International Journal of Disaster Risk Reduction, 10, 48–58. https://doi.org/10.1016/J.IJDRR.2014.06.001 | spa |
dc.relation.references | Bin, A., & Ali, M. D. (n.d.). Flood Inundation Modeling and Hazard Mapping under Uncertainty in the Sungai Johor Basin, Malaysia. | spa |
dc.relation.references | Contreras, J. L., & Contreras, Y. K. (2021). Determinación De Zonas Susceptibles A Inundación En El Municipio De Villapinzón – Cundinamarca, Mediante El Uso Del Sistema De Información Geográfica (SIG). http://www.cundinamarca.gov.co:10039/wcm/connect/9e96b703-852d-470f-9b64-3c8e601b3805/3.+Anexo+3.3+- | spa |
dc.relation.references | Costache, R., Pham, Q. B., Sharifi, E., Linh, N. T. T., Abba, S. I., Vojtek, M., Vojteková, J., Nhi, P. T. T., & Khoi, D. N. (2020). Flash-Flood Susceptibility Assessment Using Multi-Criteria Decision Making and Machine Learning Supported by Remote Sensing and GIS Techniques. Remote Sensing, 12(1). https://doi.org/10.3390/rs12010106 | spa |
dc.relation.references | Darabi, H., Choubin, B., Rahmati, O., Torabi Haghighi, A., Pradhan, B., & Kløve, B. (2019). Urban flood risk mapping using the GARP and QUEST models: A comparative study of machine learning techniques. Journal of Hydrology, 569, 142–154. https://doi.org/10.1016/J.JHYDROL.2018.12.002 | spa |
dc.relation.references | Eingrüber, N., & Korres, W. (2022). Climate change simulation and trend analysis of extreme precipitation and floods in the mesoscale Rur catchment in western Germany until 2099 using Statistical Downscaling Model (SDSM) and the Soil & Water Assessment Tool (SWAT model). Science of The Total Environment, 838, 155775. https://doi.org/10.1016/J.SCITOTENV.2022.155775 | spa |
dc.relation.references | Ernesto Hernández-Uribe, R., Barrios-Piña, H., Ramírez, A. I., & para correspondencia Resumen Hernández-Uribe, A. (2017). Análisis de riesgo por inundación: metodología y aplicación a la cuenca Atemajac. http://www.revistatyca.org.mx/ojs/index.php/tyca/article/view/1316 | spa |
dc.relation.references | Ghosh, S., Saha, S., & Bera, B. (2022). Flood susceptibility zonation using advanced ensemble machine learning models within Himalayan foreland basin. Natural Hazards Research. https://doi.org/10.1016/J.NHRES.2022.06.003 | spa |
dc.relation.references | González-Arqueros, M. L., Mendoza, M. E., Bocco, G., & Solís Castillo, B. (2018). Flood susceptibility in rural settlements in remote zones: The case of a mountainous basin in the Sierra-Costa region of Michoacán, Mexico. Journal of Environmental Management, 223, 685–693. https://doi.org/10.1016/J.JENVMAN.2018.06.075 | spa |
dc.relation.references | Haile, A. T., Tefera, F. T., & Rientjes, T. (2016). Flood forecasting in Niger-Benue basin using satellite and quantitative precipitation forecast data. INTERNATIONAL JOURNAL OF APPLIED EARTH OBSERVATION AND GEOINFORMATION, 52, 475–484. https://doi.org/10.1016/j.jag.2016.06.021 | spa |
dc.relation.references | Hosseini, F. S., Choubin, B., Mosavi, A., Nabipour, N., Shamshirband, S., Darabi, H., & Haghighi, A. T. (2020). Flash-flood hazard assessment using ensembles and Bayesian-based machine learning models: Application of the simulated annealing feature selection method. Science of The Total Environment, 711, 135161. https://doi.org/10.1016/J.SCITOTENV.2019.135161 | spa |
dc.relation.references | Jafarzadegan, K., Alipour, A., Gavahi, K., Moftakhari, H., & Moradkhani, H. (2021). Toward improved river boundary conditioning for simulation of extreme floods. Advances in Water Resources, 158, 104059. https://doi.org/10.1016/J.ADVWATRES.2021.104059 | spa |
dc.relation.references | Li, X., Rankin, C., Gangrade, S., Zhao, G., Lander, K., Voisin, N., Shao, M., Morales-Hernández, M., Kao, S. C., & Gao, H. (2021). Evaluating precipitation, streamflow, and inundation forecasting skills during extreme weather events: A case study for an urban watershed. Journal of Hydrology, 603, 127126. https://doi.org/10.1016/J.JHYDROL.2021.127126 | spa |
dc.relation.references | Lin, J. M., & Billa, L. (2021). Spatial prediction of flood-prone areas using geographically weighted regression. Environmental Advances, 6, 100118. https://doi.org/10.1016/J.ENVADV.2021.100118 | spa |
dc.relation.references | Ministerio de Ambiente y Desarrollo Sostenible. (2017). Decreto 2245 de 2017, Decreto Único Reglamentario del Sector Ambiente y Desarrollo Sostenible, en lo relacionado con el acotamiento de rondas hídricas. https://www.icbf.gov.co/cargues/avance/docs/decreto_2245_2017.htm | spa |
dc.relation.references | Ministerio de Ambiente y Desarrollo Sostenible. (2018a). Guía técnica de criterios para el acotamiento de las rondas hídricas en Colombia. http://www.mdba.gov.au/. | spa |
dc.relation.references | Ministerio de Ambiente y Desarrollo Sostenible. (2018b). Res. 957 de 2018. http://www.andi.com.co/Uploads/Res.%20957%20de%202018.pdf | spa |
dc.relation.references | Montané, A., Buffin-Bélanger, T., Vinet, F., & Vento, O. (2017). Mappings extreme floods with numerical floodplain models (NFM) in France. Applied Geography, 80, 15–22. https://doi.org/10.1016/J.APGEOG.2017.01.002 | spa |
dc.relation.references | Moreno, J. M., Sánchez, J. M., & Espitia, H. E. (2020). Use of computational intelligence techniques to predict flooding in places adjacent to the Magdalena River. Heliyon, 6(9), e04872. https://doi.org/10.1016/J.HELIYON.2020.E04872 | spa |
dc.relation.references | Mosquera-Machado, S., & Ahmad, S. (2007). Flood hazard assessment of Atrato River in Colombia. Water Resources Management, 21(3), 591–609. https://doi.org/10.1007/s11269-006-9032-4 | spa |
dc.relation.references | Nkeki, F. N., Bello, E. I., & Agbaje, I. G. (2022). Flood risk mapping and urban infrastructural susceptibility assessment using a GIS and analytic hierarchical raster fusion approach in the Ona River Basin, Nigeria. International Journal of Disaster Risk Reduction, 77, 103097. https://doi.org/10.1016/J.IJDRR.2022.103097 | spa |
dc.relation.references | Quirogaa, V. M., Kurea, S., Udoa, K., & Manoa, A. (2016). Application of 2D numerical simulation for the analysis of the February 2014 Bolivian Amazonia flood: Application of the new HEC-RAS version 5. Ribagua, 3(1), 25–33. https://doi.org/10.1016/j.riba.2015.12.001 | spa |
dc.relation.references | Rodríguez, E. (2016). Diseño metodológico para la evaluación del riesgo por inundación a nivel local con información escasa. | spa |
dc.relation.references | Samela, C., Albano, R., Sole, A., & Manfreda, S. (2018). A GIS tool for cost-effective delineation of flood-prone areas. Computers, Environment and Urban Systems, 70, 43–52. https://doi.org/10.1016/J.COMPENVURBSYS.2018.01.013 | spa |
dc.relation.references | Samela, C., Troy, T. J., & Manfreda, S. (2017). Geomorphic classifiers for flood-prone areas delineation for data-scarce environments. Advances in Water Resources, 102, 13–28. https://doi.org/10.1016/J.ADVWATRES.2017.01.007 | spa |
dc.relation.references | Semaw, F., Zeleke, G., & Balew, A. (2022). Evaluating flood risk management practices and vulnerability mapping in Alawuha watershed (North Wollo Zone, Ethiopia) using GIS and remote sensing. Applied Geomatics, 14(2), 347–367. https://doi.org/10.1007/s12518-022-00429-z | spa |
dc.relation.references | Wang, P., Li, Y., Yu, P., & Zhang, Y. (2021). The analysis of urban flood risk propagation based on the modified susceptible infected recovered model. Journal of Hydrology, 603, 127121. https://doi.org/10.1016/J.JHYDROL.2021.127121 | spa |
dc.relation.references | Werner, M., Loaiza, J. C., Rosero Mesa, M. C., Faneca Sànchez, M., de Keizer, O., & Sandoval, M. C. (2016). Developing Flood Forecasting Capabilities in Colombia (South America). Flood Forecasting: A Global Perspective, 349–368. https://doi.org/10.1016/B978-0-12-801884-2.00012-8 | spa |
dc.relation.references | Wijayarathne, D. B., & Coulibaly, P. (2020). Identification of hydrological models for operational flood forecasting in St. John’s, Newfoundland, Canada. Journal of Hydrology: Regional Studies, 27, 100646. https://doi.org/10.1016/J.EJRH.2019.100646 | spa |
dc.relation.references | Yin, J., Yu, D., Yin, Z., Wang, J., & Xu, S. (2013). Multiple scenario analyses of Huangpu River flooding using a 1D/2D coupled flood inundation model. Natural Hazards, 66(2), 577–589. https://doi.org/10.1007/s11069-012-0501-1 | spa |
dc.rights | Atribución-NoComercial-SinDerivadas 2.5 Colombia | * |
dc.rights.accessrights | info:eu-repo/semantics/openAccess | |
dc.rights.coar | http://purl.org/coar/access_right/c_abf2 | |
dc.rights.local | Abierto (Texto Completo) | spa |
dc.rights.uri | http://creativecommons.org/licenses/by-nc-nd/2.5/co/ | * |
dc.subject.keyword | Numerical models | spa |
dc.subject.keyword | Flood zones | spa |
dc.subject.keyword | Hydrodynamic models | spa |
dc.subject.keyword | Spatial statistics | spa |
dc.subject.keyword | Morphometric analysis | spa |
dc.subject.keyword | Hydrological models | spa |
dc.subject.keyword | Watershed | spa |
dc.subject.lemb | Ingeniería Ambiental | spa |
dc.subject.lemb | Ingeniería | spa |
dc.subject.lemb | Biología de agua dulce | spa |
dc.subject.proposal | Ronda hídrica | spa |
dc.subject.proposal | Modelos hidrológicos | spa |
dc.subject.proposal | Análisis morfométrico | spa |
dc.subject.proposal | Estadística espacial | spa |
dc.subject.proposal | Modelos hidrodinámicos | spa |
dc.subject.proposal | Zonas de inundación | spa |
dc.subject.proposal | Modelos numéricos | spa |
dc.title | Estado del arte sobre metodologías el acotamiento de rondas hídricas. | spa |
dc.type | bachelor thesis | |
dc.type.coar | http://purl.org/coar/resource_type/c_7a1f | |
dc.type.coarversion | http://purl.org/coar/version/c_ab4af688f83e57aa | |
dc.type.drive | info:eu-repo/semantics/bachelorThesis | |
dc.type.local | Tesis de pregrado | spa |
dc.type.version | info:eu-repo/semantics/acceptedVersion |
Archivos
Bloque original
1 - 3 de 3
Cargando...
- Nombre:
- 2022jessicaalejandratorresalbarracin.pdf
- Tamaño:
- 172.1 KB
- Formato:
- Adobe Portable Document Format
- Descripción:
- Trabajo de grado
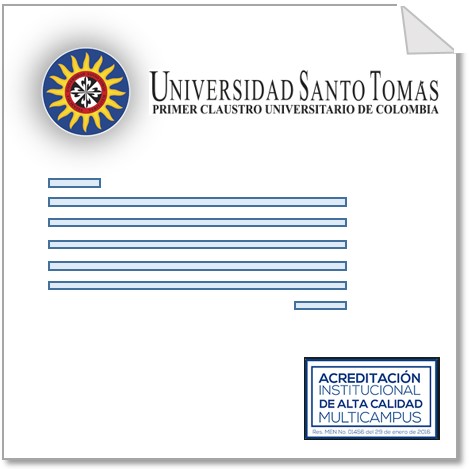
- Nombre:
- Carta_aprobacion_facultad_2021 - TORRES ALBARRACIN JESSICA ALEJANDRA - GRADO INDIVIDUAL.pdf
- Tamaño:
- 863.88 KB
- Formato:
- Adobe Portable Document Format
- Descripción:
- Carta aprobación facultad
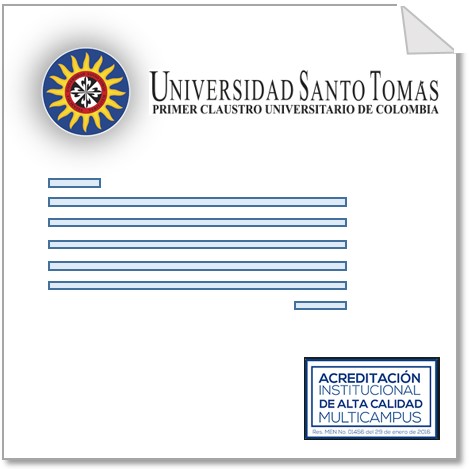
- Nombre:
- Carta_autorizacion_autoarchivo_autor_2021-páginas-eliminadas-firmado.pdf
- Tamaño:
- 928.61 KB
- Formato:
- Adobe Portable Document Format
- Descripción:
- Carta derechos de autor
Bloque de licencias
1 - 1 de 1
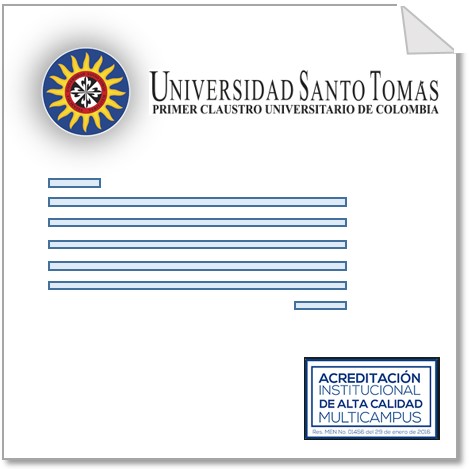
- Nombre:
- license.txt
- Tamaño:
- 807 B
- Formato:
- Item-specific license agreed upon to submission
- Descripción: