Characterization of postures to analyze people’s emotions using Kinect technology
dc.contributor.author | Monsalve-Pulido, Julián Alberto | spa |
dc.contributor.author | Parra-Rodríguez, Carlos Alberto | spa |
dc.coverage.campus | CRAI-USTA Bogotá | spa |
dc.date.accessioned | 2019-07-05T20:45:33Z | spa |
dc.date.available | 2019-07-05T20:45:33Z | spa |
dc.date.issued | 2018-04-01 | spa |
dc.description | El presente artículo sintetiza la investigación realizada en el uso de técnicas de clasificación para un proceso de caracterización de posturas de personas que tiene como objetivo la identificación de emociones (Asombro, Enfado, Felicidad y Tristeza). En este proyecto de investigación fue necesario utilizar una metodología de investigación exploratoria en tres fases donde el resultado es una apropiación tecnológica y un modelo de clasificación de emociones en personas en posición de pie, usando el algoritmo de Skeletal Tracking de Kinect basado en software libre. Se propuso un vector de características para el reconocimiento de patrones usando técnicas de clasificación como SVM, KNN y Redes Bayesianas en 17.882 datos obtenidos en una muestra de entrenamiento de 14 personas. Como resultado se evidenció que el algoritmo KNN tiene una efectividad máxima del 89.0466% superando a los demás algoritmos seleccionados. | spa |
dc.description.abstract | This article synthesizes the research undertaken into the use of classification techniques that characterize people's positions, the objective being to identify emotions (astonishment, anger, happiness and sadness). We used a three-phase exploratory research methodology, which resulted in technological appropriation and a model that classified people’s emotions (in standing position) using the Kinect Skeletal Tracking algorithm, which is a free software. We proposed a feature vector for pattern recognition using classification techniques such as SVM, KNN, and Bayesian Networks for 17,882 pieces of data that were obtained in a 14-person training sample. As a result, we found that that the KNN algorithm has a maximum effectiveness of 89.0466%, which surpasses the other selected algorithms. | spa |
dc.description.domain | http://unidadinvestigacion.usta.edu.co | spa |
dc.format.mimetype | application/pdf | spa |
dc.identifier.citation | Monsalve-Pulido, J. A., & Parra-Rodríguez, C. A. (2018). Characterization of postures to analyze people’s emotions using kinect technology. Bogotá: doi:10.15446/dyna.v85n205.69470 | spa |
dc.identifier.doi | https://doi.org/10.15446/dyna.v85n205.69470 | spa |
dc.identifier.uri | http://hdl.handle.net/11634/17475 | |
dc.relation.references | Mann, S., Intelligent image processing. IEEE, John Wiley & Sons, Inc., 2002. DOI: /10.1002/0471221635 | spa |
dc.relation.references | Valli, A., Natural interaction white paper, 2007. | spa |
dc.relation.references | Rivera-Mateos, M., El turismo experiencial como forma de turismo responsable e intercultural, en: García-Rodríguez, L., Roldán-Tapía, A.R., Eds., Relac. Intercult. en la Divers., 2013, pp. 199-217. | spa |
dc.relation.references | Smith, W.L., Experiential tourism around the world and at home: definitions and standards, Int. J. Serv. Stand., 2(1), 1 P, 2006. DOI: 10.1504/IJSS.2006.008156 | spa |
dc.relation.references | Dale, R., Moisl, H. and Somers, H.L., Handbook of natural language processing, Marcel Dekker, 2000. | spa |
dc.relation.references | Cambria, E. and Hussain, A., Sentic album: content-, concept-, and context-based online personal photo management system, Cognit. Comput., 4(4), pp. 477-496, 2012. DOI: 10.1007/s12559-012-9145-4ch | spa |
dc.relation.references | Simon-Kemp, W.A.S., Digital in 2016, 2016. | spa |
dc.relation.references | Cambria, E., Livingstone, A. and Hussain, A. The Hourglass of Emotions. In: Esposito, A., Esposito, A.M., Vinciarelli, A., Hoffmann, R. and, Müller, V.C., (eds.), Cognitive Behavioural Systems. Lecture Notes in Computer Science, vol 7403. Springer, Berlin, Heidelberg. 2012. DOI: 10.1007/978-3-642-34584-5_11 | spa |
dc.relation.references | Minsky, M., The emotion machine: commonsense thinking, artificial intelligence, and the future of the human mind, 2007. | spa |
dc.relation.references | Vesterinen, E., Affective computing. Pattern Analysis and Applications, 1(1), pp. 71-73, 1998. | spa |
dc.relation.references | Siegman, A.W. and Feldstein, S., Nonverbal behavior and communication. L. Erlbaum, 1987. | spa |
dc.relation.references | Pons, C., Comunicación no verbal. Barcelona: Editorial Kairós, 2015. | spa |
dc.relation.references | Kaur, A. and Gupta, V., A survey on sentiment analysis and opinion mining techniques, J. Emerg. Technol. Web Intell., 5(4), pp. 367-371, 2013. DOI: 10.4304/jetwi.5.4.367-3 | spa |
dc.relation.references | Gamon, M., Aue, A., Corston-Oliver, S. and Ringger, E., Pulse: mining customer opinions from free text, Springer, Berlin, Heidelberg, 2005, pp. 121-132. DOI: 10.1007/11552253_12 | spa |
dc.relation.references | Poria, S., Cambria, E., Hussain, A. and Bin Huang, G., Towards an intelligent framework for multimodal affective data analysis, Neural Networks, 63, pp. 104-116, 2015. DOI: 10.1016/j.neunet.2014.10.005 | spa |
dc.relation.references | Kapoor, A., Burleson, W. and R Picard, W., Automatic prediction of frustration, Int. J. Hum. Comput. Stud., 65(8), pp. 724-736, 2007. DOI: 10.1016/J.IJHCS.2007.02.003 | spa |
dc.relation.references | Lisetti, C.L., Pattern Analysis & Applic, 1, J. Wiley, 1998, 71 P. DOI: 10.1007/BF01238028 | spa |
dc.relation.references | Yan, J., Zheng, W., Xu, Q., Lu, G., Li, H. and Wang, B., Sparse Kernel reduced-rank regression for bimodal emotion recognition from facial expression and speech, IEEE Trans. Multimed., 18(7), pp. 1319-1329, 2016. DOI: 10.1109/TMM.2016.2557721 | spa |
dc.relation.references | Poria, S., Cambria, E., Howard, N., Bin Huang, G. and Hussain, A., Fusing audio, visual and textual clues for sentiment analysis from multimodal content, Neurocomputing, 174, pp. 50-59, 2016. DOI: 10.1016/j.neucom.2015.01.095 | spa |
dc.relation.references | Benhumea, H.S., Interfaz de lenguaje natural usando Kinect. Unidad Zacatenco, 2012. | spa |
dc.rights | Atribución-NoComercial-CompartirIgual 2.5 Colombia | * |
dc.rights.uri | http://creativecommons.org/licenses/by-nc-sa/2.5/co/ | * |
dc.subject.keyword | Analysis of emotions | spa |
dc.subject.keyword | Recognition of postures | spa |
dc.subject.keyword | Free software | spa |
dc.subject.keyword | Kinect | spa |
dc.subject.keyword | KNN | spa |
dc.subject.proposal | Análisis de emociones | spa |
dc.subject.proposal | Reconocimiento de posturas | spa |
dc.subject.proposal | Software libre | spa |
dc.subject.proposal | Kinect | spa |
dc.subject.proposal | KNN | spa |
dc.title | Characterization of postures to analyze people’s emotions using Kinect technology | spa |
dc.type.category | Generación de Nuevo Conocimiento: Artículos publicados en revistas especializadas - Electrónicos | spa |
Archivos
Bloque original
1 - 1 de 1
Cargando...
- Nombre:
- Characterization of postures to analyze people’s emotions using Kinect technology.pdf
- Tamaño:
- 839.32 KB
- Formato:
- Adobe Portable Document Format
- Descripción:
- Artículo SCOPUS
Bloque de licencias
1 - 1 de 1
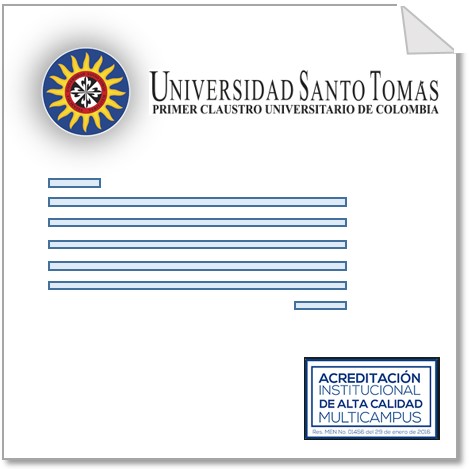
- Nombre:
- license.txt
- Tamaño:
- 807 B
- Formato:
- Item-specific license agreed upon to submission
- Descripción: