Estimación y caracterización de la cobertura vegetal basado en las aplicaciones de las aeronaves no tripuladas UAS
dc.contributor.advisor | Sierra Parada, Ronal Jackson | |
dc.contributor.author | Ordoñez Vanegas, Laura Camila | |
dc.contributor.author | Pedraza Torres, Ivan David | |
dc.contributor.corporatename | Universidad Santo Tomás | spa |
dc.contributor.cvlac | https://scienti.minciencias.gov.co/cvlac/visualizador/generarCurriculoCv.do?cod_rh=0001431760 | spa |
dc.contributor.cvlac | https://scienti.minciencias.gov.co/cvlac/visualizador/generarCurriculoCv.do?cod_rh=0002059055 | spa |
dc.contributor.googlescholar | https://scholar.google.com/citations?hl=es&user=0793qhcwBoMC | spa |
dc.contributor.orcid | https://orcid.org/0000-0002-9206-5682 | spa |
dc.coverage.campus | CRAI-USTA Bogotá | spa |
dc.date.accessioned | 2023-04-25T15:01:04Z | |
dc.date.available | 2023-04-25T15:01:04Z | |
dc.date.issued | 2023-04-24 | |
dc.description | En la actualidad, diversos estudios demuestran la eficacia de las aeronaves no tripuladas (UAS) en la estimación y caracterización de la cobertura vegetal, puesto que estas herramientas permiten la obtención de datos precisos a diferentes escalas espaciales y temporales, lo que las convierte en una valiosa herramienta para la monitorización de la vegetación. En este sentido, el propósito de esta investigación es destacar la importancia de los UAV en estudios ambientales, así como su desarrollo y avances en los últimos años. La metodología de estudio se basó en un análisis de correlación de palabras clave, países y años, a través de VOSviewer y bibliometrix, usando bases de datos como Scopus y ScienceDirect, y se centró en enfoques de aplicación, como la identificación y caracterización de la cobertura vegetal y sus especies mediante la captura de imágenes multiespectrales y el uso de inteligencia artificial con UAV. Con base en lo anterior, se encontró una correlación general de palabras clave que se enfocan en aplicaciones de UAV, como aprendizaje automático, sensores remotos y árboles de decisión. También se analizaron las marcas líderes en la suministración de tecnología, incluyendo cámaras, aeronaves y sensores utilizados, así como los modelos más frecuentemente empleados en los estudios revisados para analizar la tendencia del mercado en este campo tecnológico. | spa |
dc.description.abstract | Currently, several studies demonstrate the effectiveness of unmanned aerial vehicles (UAS) in the estimation and characterization of vegetation cover, since these tools allow obtaining accurate data at different spatial and temporal scales, which makes them a valuable tool for vegetation monitoring. In this sense, the purpose of this research is to highlight the importance of UAVs in environmental studies, as well as their development and advances in recent years. The study methodology was based on a correlation analysis of keywords, countries and years, through VOSviewer and bibliometrix, using databases such as Scopus and ScienceDirect, and focused on application approaches, such as the identification and characterization of vegetation cover and its species through the capture of multispectral images and the use of artificial intelligence with UAVs. Based on the above, a general correlation of keywords focusing on UAV applications, such as machine learning, remote sensing and decision trees, was found. The leading brands in the supply of technology, including cameras, aircraft and sensors used, as well as the most frequently used models in the reviewed studies were also analyzed to analyze the market trend in this technological field. | spa |
dc.description.degreelevel | Pregrado | spa |
dc.description.degreename | Ingeniero Ambiental | spa |
dc.format.mimetype | application/pdf | spa |
dc.identifier.citation | Ordoñez Vanegas, L. C., y Pedraza Torres, I. D. (2023). Estimación y caracterización de la cobertura vegetal basado en las aplicaciones de las aeronaves no tripuladas UAS. [Trabajo de Grado, Universidad Santo Tomás]. Repositorio Institucional. | spa |
dc.identifier.instname | instname:Universidad Santo Tomás | spa |
dc.identifier.reponame | reponame:Repositorio Institucional Universidad Santo Tomás | spa |
dc.identifier.repourl | repourl:https://repository.usta.edu.co | spa |
dc.identifier.uri | http://hdl.handle.net/11634/50495 | |
dc.language.iso | spa | spa |
dc.publisher | Universidad Santo Tomás | spa |
dc.publisher.faculty | Facultad de Ingeniería Ambiental | spa |
dc.publisher.program | Pregrado de Ingeniería Ambiental | spa |
dc.relation.references | Aragón, G. C., Cubillos Rodríguez, J. P., & Delgado-Niño, P. (2021). Uso de los vehículos aéreos no tripulados (drones) para el levantamiento de información primaria en los estudios ambientales por parte de las consultoras ambientales Use of Unmanned Aerial Vehicles (drones) for the Collection of Primary Information in Environmental Studies by Environmental Consultants. https://orcid.org/0000-0002-6166-4025 | spa |
dc.relation.references | Cuervo Martínez, K. X. (2020). Adquisición y análisis de imágenes UAV para la estimación de coberturas vegetales y biomasa. | spa |
dc.relation.references | De Alfonso Juliá, J. C. (2020). Dron de vuelo autónomo con reconocimiento basado en inteligencia artificial. | spa |
dc.relation.references | El Hoummaidi, L., Larabi, A., & Alam, K. (2021). Using unmanned aerial systems and deep learning for agriculture mapping in Dubai. Heliyon, 7(10). https://doi.org/10.1016/j.heliyon.2021.e08154 | spa |
dc.relation.references | Feng, Q., Liu, J., & Gong, J. (2018). UAV Remote sensing for urban vegetation mapping using random forest and texture analysis. Remote Sensing, 7(1), 1074–1094. https://doi.org/10.3390/rs70101074 | spa |
dc.relation.references | Gallmann, J., Schüpbach, B., Jacot, K., Albrecht, M., Winizki, J., Kirchgessner, N., & Aasen, H. (2022). Flower Mapping in Grasslands With Drones and Deep Learning. Frontiers in Plant Science, 12. https://doi.org/10.3389/fpls.2021.774965 | spa |
dc.relation.references | Guimbao, J. F. (2021). Inteligencia artificial: una carrera hacia el futuro tecnológico. bie3: Boletín IEEE, (23), 146-163. | spa |
dc.relation.references | Gutierrez-Alonso, G. (2016). Aplicaciones Geológicas de los Drones-Geological Applications of UAVs Assessing crustal recycling and granite magma generation in Central Iberia (IBERCRUST) View project Material Docente Cartografía Geológica y Geología Estructural View project. https://www.researchgate.net/publication/303696594 | spa |
dc.relation.references | Hartling, S., Sagan, V., & Maimaitijiang, M. (2021). Urban tree species classification using UAV-based multi-sensor data fusion and machine learning. GIScience and Remote Sensing, 58(8), 1250–1275. https://doi.org/10.1080/15481603.2021.1974275 | spa |
dc.relation.references | Kharuf-Gutierrez, S., Hernández-Santana, L., Orozco-Morales, R., de la Aday Díaz, O. C., & Delgado Mora, I. (2018). Análisis de imágenes multiespectrales adquiridas con vehículos aéreos no tripulados. In RIELAC (Issue 2). | spa |
dc.relation.references | Lee, G., Hwang, J., & Cho, S. (2021). A novel index to detect vegetation in urban areas using uav-based multispectral images. Applied Sciences (Switzerland), 11(8). https://doi.org/10.3390/app11083472 | spa |
dc.relation.references | Liang, D., Liu, W., & Zhao, Y. (2022). Optimal Models for Plant Disease and Pest Detection Using UAV Image. Nature Environment and Pollution Technology, 21(4), 1609–1617. https://doi.org/10.46488/NEPT.2022.v21i04.013 | spa |
dc.relation.references | Liu, D. zhong, Yang, F. fei, & Liu, S. ping. (2021). Estimating wheat fractional vegetation cover using a density peak k-means algorithm based on hyperspectral image data. Journal of Integrative Agriculture, 20(11), 2880–2891. https://doi.org/10.1016/S2095-3119(20)63556-0 | spa |
dc.relation.references | Marcial-Pablo, M. de J., Ontiveros-Capurata, R. E., Jiménez-Jiménez, S. I., & Ojeda-Bustamante, W. (2021). Maize crop coefficient estimation based on spectral vegetation indices and vegetation cover fraction derived from uav-based multispectral images. Agronomy, 11(4). https://doi.org/10.3390/AGRONOMY11040668 | spa |
dc.relation.references | Oddi, L., Cremonese, E., Ascari, L., Filippa, G., Galvagno, M., Serafino, D., & di Cella, U. M. (2021). Using UAV imagery to detect and map woody species encroachment in a subalpine grassland: Advantages and limits. Remote Sensing, 13(7). https://doi.org/10.3390/rs13071239 | spa |
dc.relation.references | Olariu, H. G., Malambo, L., Popescu, S. C., Virgil, C., & Wilcox, B. P. (2022). Woody Plant Encroachment: Evaluating Methodologies for Semiarid Woody Species Classification from Drone Images. Remote Sensing, 14(7). https://doi.org/10.3390/rs14071665 | spa |
dc.relation.references | Pérez Izquierdo, C. (2020). Uso de imágenes multiespectrales de drones para evaluar el estado del arbolado en la dehesa. Catálogo de investigación joven en Extremadura. Volumen III. | spa |
dc.relation.references | Pinzón, B. F. S., Ortega, J. R. T., & Rosa, P. (2016). Drones: General aspects and social applications. Visión electrónica, 10(2), 11. | spa |
dc.relation.references | Revathi, K., Tamilselvi, T., Arunkumar, R., & Samydurai, A. (2022). A SMART DRONE FOR ENSURING PRECISION AGRICULTURE WITH ARTIFICIAL NEURAL NETWORK. Indian Journal of Computer Science and Engineering, 13(3), 897–906. https://doi.org/10.21817/indjcse/2022/v13i3/221303025 | spa |
dc.relation.references | Rivera, L. B., Bonilla, B. M., & Obando-Vidal, F. (2021). Processing multispectral imaging captured by drones to evaluate the normalized difference vegetation index of Castillo coffee plantations. Ciencia Tecnología Agropecuaria, 22(1). https://doi.org/10.21930/RCTA.VOL22_NUM1_ART:1578 | spa |
dc.relation.references | Saravia, V., Moraes, W., Kelbouscas, A., & Grando, R. (2022). Drones e Inteligencia Artificial para Investigaci\'on y Competici\'on. arXiv preprint arXiv:2210.11199 | spa |
dc.relation.references | Sohrabi, H., Gao, Y., Bhardwaj, A., & Coomes, D. A. (2022). Monitoring early-successional trees for tropical forest restoration using low-cost UAV-based species classification. | spa |
dc.relation.references | Valente, J., Sari, B., Kooistra, L., Kramer, H., & Mücher, S. (2020). Automated crop plant counting from very high-resolution aerial imagery. Precision Agriculture, 21(6), 1366–1384. https://doi.org/10.1007/s11119-020-0972+5-3 | spa |
dc.relation.references | Wei, Y., Wang, W., Tang, X., Li, H., Hu, H., & Wang, X. (2022). Classification of Alpine Grasslands in Cold and High Altitudes Based on Multispectral Landsat-8 Images: A Case Study in Sanjiangyuan National Park, China. Remote Sensing, 14(15). https://doi.org/10.3390/rs14153714 | spa |
dc.relation.references | Xie, L., Meng, X., Zhao, X., Fu, L., Sharma, R. P., & Sun, H. (2022). Estimating Fractional Vegetation Cover Changes in Desert Regions Using RGB Data. Remote Sensing, 14(15). https://doi.org/10.3390/rs14153833 | spa |
dc.relation.references | Xu, Z., Shen, X., Cao, L., Coops, N. C., Goodbody, T. R. H., Zhong, T., Zhao, W., Sun, Q., Ba, S., Zhang, Z., & Wu, X. (2020). Tree species classification using UAS-based digital aerial photogrammetry point clouds and multispectral imageries in subtropical natural forests. International Journal of Applied Earth Observation and Geoinformation, 92. https://doi.org/10.1016/j.jag.2020.102173 | spa |
dc.relation.references | Yang, H., Hu, Y., Zheng, Z., Qiao, Y., Zhang, K., Guo, T., & Chen, J. (2022). Estimation of Potato Chlorophyll Content from UAV Multispectral Images with Stacking Ensemble Algorithm. Agronomy, 12(10). https://doi.org/10.3390/agronomy12102318 | spa |
dc.relation.references | Zhao, T., Huang, L., Wang, J., Cui, B., Li, X., & Zhu, X. (2022). Effective Screening and Texture Segmentation of Green Vegetation Cover Based on UAV Images. Traitement Du Signal, 39(4), 1435–1442. https://doi.org/10.18280/ts.39043 | spa |
dc.rights | Atribución-NoComercial-SinDerivadas 2.5 Colombia | * |
dc.rights.accessrights | info:eu-repo/semantics/openAccess | |
dc.rights.coar | http://purl.org/coar/access_right/c_abf2 | spa |
dc.rights.local | Abierto (Texto Completo) | spa |
dc.rights.uri | http://creativecommons.org/licenses/by-nc-nd/2.5/co/ | * |
dc.subject.keyword | Unmanned aerial vehicles | spa |
dc.subject.keyword | Multispectral imagery | spa |
dc.subject.keyword | Vegetation cover | spa |
dc.subject.keyword | Machine learning | spa |
dc.subject.keyword | Artificial intelligence | spa |
dc.subject.lemb | Ingeniería Ambiental | spa |
dc.subject.lemb | Aeronave | spa |
dc.subject.lemb | Vegetación | spa |
dc.subject.lemb | Bases de Datos | spa |
dc.subject.proposal | Vehículos aéreos no tripulados | spa |
dc.subject.proposal | Imágenes multiespectrales | spa |
dc.subject.proposal | Cobertura vegetal | spa |
dc.subject.proposal | Aprendizaje automático | spa |
dc.subject.proposal | Inteligencia artificial | spa |
dc.title | Estimación y caracterización de la cobertura vegetal basado en las aplicaciones de las aeronaves no tripuladas UAS | spa |
dc.type | bachelor thesis | |
dc.type.coar | http://purl.org/coar/resource_type/c_7a1f | |
dc.type.coarversion | http://purl.org/coar/version/c_ab4af688f83e57aa | |
dc.type.drive | info:eu-repo/semantics/bachelorThesis | |
dc.type.local | Tesis de pregrado | spa |
dc.type.version | info:eu-repo/semantics/acceptedVersion |
Archivos
Bloque original
1 - 3 de 3
Cargando...
- Nombre:
- 2023lauraordoñez-davidpedraza.pdf
- Tamaño:
- 457.43 KB
- Formato:
- Adobe Portable Document Format
- Descripción:
- Trabajo de Grado
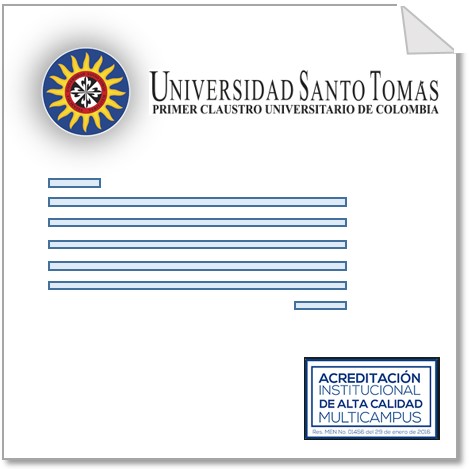
- Nombre:
- Carta_aprobacion_facultad_ ORDOÑEZ VANEGAS LAURA CAMILA - PEDRAZA TORRES IVAN DAVID.pdf
- Tamaño:
- 1.1 MB
- Formato:
- Adobe Portable Document Format
- Descripción:
- Carta de aprobación facultad
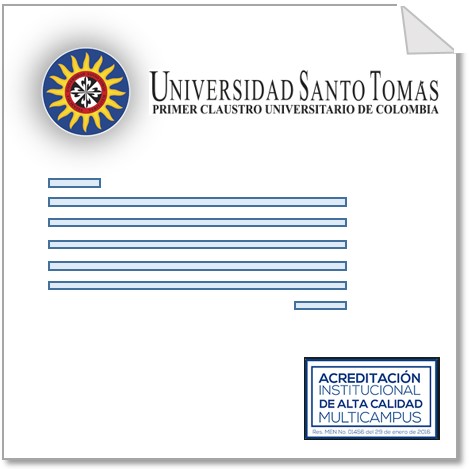
- Nombre:
- Carta_autorizacion_autoarchivo_autores.pdf
- Tamaño:
- 1.01 MB
- Formato:
- Adobe Portable Document Format
- Descripción:
- Carta Derechos de Autor
Bloque de licencias
1 - 1 de 1
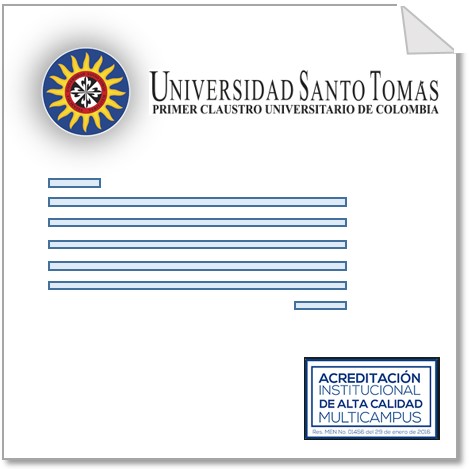
- Nombre:
- license.txt
- Tamaño:
- 807 B
- Formato:
- Item-specific license agreed upon to submission
- Descripción: