Functional SAR models: with application to spatial econometrics
dc.contributor.author | Pineda-Ríos, Wilmer | spa |
dc.contributor.author | Giraldo, Ramón | spa |
dc.contributor.author | Porcu, Emilio | spa |
dc.coverage.campus | CRAI-USTA Bogotá | spa |
dc.date.accessioned | 2019-06-18T22:07:06Z | spa |
dc.date.available | 2019-06-18T22:07:06Z | spa |
dc.date.issued | 2018-12-19 | spa |
dc.description.abstract | Simultaneous autoregressive (SAR) models have been extensively used for the analysis of spatial data in areas as diverse as demography, economy and geography. These are linear models with a scalar response, scalar explanatory variables and autoregressive errors. In this work we extend this modeling approach from scalar to functional covariates. Least squares and maximum likelihood are used as estimation methods of the parameters. A simulation study is considered for evaluating the performance of the proposed methodology. As an illustration, the model is used to establish the relationship between unsatisfied basic needs and curves of gross domestic product obtained in 32 departments of Colombia (districts of the country). | spa |
dc.description.domain | http://unidadinvestigacion.usta.edu.co | spa |
dc.format.mimetype | application/pdf | spa |
dc.identifier.citation | Pineda-Ríos, W., Giraldo, R., & Porcu, E. (2018). Functional SAR models: With application to spatial econometrics. Bogotá: doi:10.1016/j.spasta.2018.12.002 | spa |
dc.identifier.doi | https://doi.org/10.1016/j.spasta.2018.12.002 | spa |
dc.identifier.uri | http://hdl.handle.net/11634/17176 | |
dc.relation.references | Aguilera-Morillo, M.C., Durbán, M., Aguilera, A.M., 2017. Prediction of functional data with spatial dependence: a penalized approach. Stoch. Environ. Res. Risk Assess. 31 (1), 7–22. | spa |
dc.relation.references | Ahmed, M., 2017. Contribution à la statistique spatiale et l’analyse de données fonctionnelles (Ph.D. thesis), Department of Mathematics, University of Lille. | spa |
dc.relation.references | Bali, J.L., Boente, G., Tyler, D.E., Wang, J.-L., et al., 2011. Robust functional principal components: a projection-pursuit approach. Ann. Statist. 39 (6), 2852–2882. | spa |
dc.relation.references | Box, G.E., Jenkins, G.M., Reinsel, G.C., Ljung, G.M., 2015. Time series analysis: forecasting and control. John Wiley & Sons. Caballero, W., Giraldo, R., Mateu, J., 2013. A universal kriging approach for spatial functional data. Stoch. Environ. Res. Risk Assess. 27 (7), 1553–1563. | spa |
dc.relation.references | Cardot, H., Ferraty, F., Mas, A., Sarda, P., 2003. Testing hypotheses in the functional linear model. Scand. J. Stat. 30 (1), 241–255. | spa |
dc.relation.references | Chiou, J., Müller, H., Wang, J., 2004. Functional response models. Statist. Sinica 14, 675–693. | spa |
dc.relation.references | Dabo-Niang, S., Yao, F., 2007. Kernel regression estimation for continuous spatial processes. Math. Methods Statist. 16 (4), 298–317. | spa |
dc.relation.references | Delicado, P., Broner, S., 2008. Distance-based LISA Maps for Multivariate Lattice Data. In: Technical Report, Universitat Politècnica de Catalunya, pp. 1–20. | spa |
dc.relation.references | Fox, J., 1984. Linear Statistical Models and Related Methods with Applications to Social Research. John Wiley & Sons. | spa |
dc.relation.references | Giraldo, R., Caballero, W., Camacho-Tamayo, J., 2018. Mantel test for spatial functional data. Adv. Statist. Anal. 102 (1), 21–39. | spa |
dc.relation.references | Haining, R., 1993. Spatial Data Analysis in the Social and Environmental Sciences. Cambridge University Press. | spa |
dc.relation.references | Horváth, L., Kokoszka, P., 2012. Inference for Functional Data with Applications. Springer. | spa |
dc.relation.references | James, G., Hastie, T., 2001. Functional linear discriminant analysis for irregularly sampled curves. J. R. Stat. Soc. Ser. B Stat. Methodol. 63 (3), 533–550. | spa |
dc.relation.references | Ord, K., 1975. Estimation methods for models of spatial interaction. J. Amer. Statist. Assoc. 70 (349), 120–126. | spa |
dc.relation.references | Pineda-Ríos, W., Giraldo, R., 2016. Functional SAR Model, ArXiv e-prints, 1609.03680, http://adsabs.harvard.edu/abs/2016arXi v160903680P. | spa |
dc.relation.references | Ramsay, J., Hooker, G., Graves, S., 2009. Functional Data Analysis with R and MATLAB. Springer Science & Business Media. | spa |
dc.relation.references | Schabenberger, O., Gotway, C.A., 2005. Statistical Methods for Spatial Data Analysis. Chapman & Hall. | spa |
dc.relation.references | Tarpey, T., Kinateder, K., 2003. Clustering functional data. J. Classification 20 (1), 93–114. | spa |
dc.relation.references | Waller, L., Gotway, C., 2004. Applied Spatial Statistics for Public Health Data. John Wiley & Sons. | spa |
dc.relation.references | Zhang, J., 2013. Analysis of Variance for Functional Data. CRC Press. | spa |
dc.rights | Atribución-NoComercial-CompartirIgual 2.5 Colombia | * |
dc.rights.uri | http://creativecommons.org/licenses/by-nc-sa/2.5/co/ | * |
dc.subject.keyword | Autoregressive errors | spa |
dc.subject.keyword | Functional linear models | spa |
dc.subject.keyword | Spatial dependence | spa |
dc.subject.keyword | Spatial weight matrix | spa |
dc.title | Functional SAR models: with application to spatial econometrics | spa |
dc.type.category | Generación de Nuevo Conocimiento: Artículos publicados en revistas especializadas - Electrónicos | spa |
Files
Original bundle
1 - 1 of 1
Loading...
- Name:
- Functional SAR models: with application to spatial econometrics.pdf
- Size:
- 1.22 MB
- Format:
- Adobe Portable Document Format
- Description:
- Artículo SCOPUS
License bundle
1 - 1 of 1
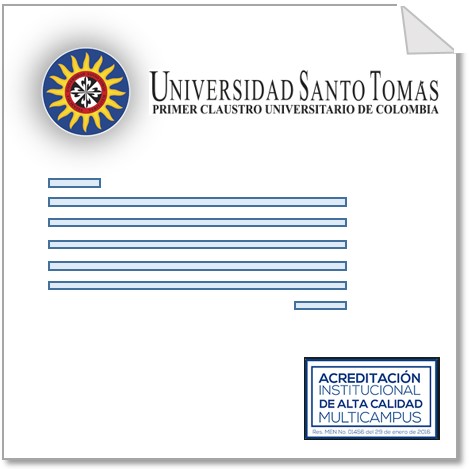
- Name:
- license.txt
- Size:
- 807 B
- Format:
- Item-specific license agreed upon to submission
- Description: