Sistema de Localización para Vehículos Autónomos Basado en Visión Artificial
dc.contributor.advisor | Calderón Chávez, Juan Manuel | |
dc.contributor.advisor | Amaya, Sindy Paola | |
dc.contributor.author | Puerto Hernández, Juan David | |
dc.contributor.corporatename | Universidad Santo Tomás | spa |
dc.contributor.cvlac | https://scienti.minciencias.gov.co/cvlac/visualizador/generarCurriculoCv.do?cod_rh=0000380938 | spa |
dc.contributor.cvlac | https://scienti.minciencias.gov.co/cvlac/visualizador/generarCurriculoCv.do?cod_rh=0000796425 | spa |
dc.contributor.googlescholar | https://scholar.google.com/citations?user=Gg2sofAAAAAJ&hl=es&oi=ao | spa |
dc.contributor.orcid | https://orcid.org/0000-0002-4471-3980 | spa |
dc.contributor.orcid | https://orcid.org/0000-0002-1714-1593 | spa |
dc.coverage.campus | CRAI-USTA Bogotá | spa |
dc.date.accessioned | 2024-12-05T14:54:55Z | |
dc.date.available | 2024-12-05T14:54:55Z | |
dc.date.issued | 2023 | |
dc.description | El presente trabajo de grado tiene como objetivo el desarrollo de un sistema para la georreferenciación de un vehículo autónomo basado en visión artificial, con un enfoque específico en la utilización de redes neuronales, aplicado en la plataforma DeepRacer de la empresa Amazon Web Services (AWS). Se exploró la aplicación innovadora de la Red Neuronal Convolucional (CNN) como regresor para abordar la desafiante tarea de localización en el vehículo autónomo AWS DeepRacer. La CNN, entrenada con un dataset integral de imágenes y coordenadas espaciales, demostró ser eficaz en la predicción precisa de ubicaciones en un entorno bidimensional. Además, se evaluó exhaustivamente el desempeño de la CNN en comparación con el sistema de posicionamiento Lighthouse Position Deck, destacando la importancia de este último como un marco de refe rencia crucial. Se identificaron modelos específicos de la CNN que exhibieron un rendimiento destacado, minimizando las discrepancias entre las predicciones y las coordenadas reales. La investigación proporcionó percepciones valiosas sobre la efectividad de la CNN en entornos es pecíficos, estableciendo una base para la optimización continua de sistemas de localización en el AWS DeepRacer. La comparación entre la CNN y el sistema Lighthouse Position Deck contri buyó a la comprensión integral de los enfoques, sus fortalezas y limitaciones, y señaló áreas para investigaciones futuras en el campo de vehículos autónomos. La aplicación de visión artificial y redes neuronales ocupa una posición central en este proyecto, capacitando al vehículo autónomo para procesar y interpretar su entorno mediante datos visuales. Estas tecnologías posibilitan la identificación de puntos de referencia y características locales, facilitando el cálculo preciso de la posición del vehículo en su entorno. Este enfoque representa una destacada aplicación de la inteligencia artificial y el aprendizaje profundo en el contexto de la navegación autónoma, subrayando la relevancia y el impacto de estas innovaciones en el desarrollo de vehículos autónomos. | spa |
dc.description.abstract | The present thesis aims to develop a system for the georeferencing of an autonomous vehicle based on computer vision, with a specific focus on the use of neural networks, applied on the Amazon Web Services (AWS) DeepRacer platform. An innovative application of Convolutional Neural Network (CNN) as a regressor was explored to address the challenging task of localization in the AWS DeepRacer autonomous vehicle. The CNN, trained with a comprehensive dataset of images and spatial coordinates, proved effective in accurately predicting locations in a two-dimensional environment. Additionally, the perfor mance of the CNN was thoroughly evaluated in comparison to the Lighthouse Position Deck positioning system, highlighting the latter’s importance as a crucial reference framework. Spe cific CNN models that exhibited outstanding performance, minimizing discrepancies between predictions and actual coordinates, were identified. The research provided valuable insights into the effectiveness of CNNs in specific environments, establishing a foundation for the continuous optimization of localization systems in AWS DeepRacer. The comparison between CNN and the Lighthouse Position Deck system contributed to a comprehensive understanding of the approa ches, their strengths and limitations, and pointed out areas for future research in the field of autonomous vehicles. The application of computer vision and neural networks plays a central role in this project, empowering the autonomous vehicle to process and interpret its environment through visual data. These technologies enable the identification of landmarks and local features, facilitating the precise calculation of the vehicle’s position in its surroundings. This approach represents a prominent application of artificial intelligence and deep learning in the context of autonomous navigation, emphasizing the relevance and impact of these innovations in the development of autonomous vehicles. | spa |
dc.description.degreelevel | Pregrado | spa |
dc.description.degreename | Ingeniero Electronico | spa |
dc.format.mimetype | application/pdf | spa |
dc.identifier.citation | Puerto Hernández, J. D. (2023). Sistema de Localización para Vehículos Autónomos Basado en Visión Artificial. [Trabajo de Grado, Universidad Santo Tomás]. Repositorio Institucional. | spa |
dc.identifier.instname | instname:Universidad Santo Tomás | spa |
dc.identifier.reponame | reponame:Repositorio Institucional Universidad Santo Tomás | spa |
dc.identifier.repourl | repourl:https://repository.usta.edu.co | spa |
dc.identifier.uri | http://hdl.handle.net/11634/58783 | |
dc.language.iso | spa | spa |
dc.publisher | Universidad Santo Tomás | spa |
dc.publisher.faculty | Facultad de Ingeniería Electrónica | spa |
dc.publisher.program | Pregrado Ingeniería Electrónica | spa |
dc.relation.references | https://aws.amazon.com/es/what-is-aws/. Accessed: 2023-11-21. | spa |
dc.relation.references | https://www.bitcraze.io/documentation/hardware/crazyradio_pa/ crazyradio_pa-datasheet.pdf.Accessed:2023-9-4. | spa |
dc.relation.references | https://aws.amazon.com/es/what-is/neural-network/.Accessed:2023-9- 4. | spa |
dc.relation.references | Revista INGENIERÍA UC. https://www.redalyc.org/pdf/707/70732640002.pdf. Accessed: 2023-11-21. | spa |
dc.relation.references | Dávila Martınez y Francisco Javier. Georreferenciación de documentos carto gráficos para la gestión de Archivos y Cartotecas. “Propuesta Metodológica”. https://www.ign.es/web/resources/docs/IGNCnig/CTC- Ibercarto- V Georreferenciacion.pdf. Accessed: 2023-11-21. | spa |
dc.relation.references | A Pozo-Ruz et al. SISTEMA DE POSICIONAMIENTO GLOBAL (GPS): DESCRIPCIÓN, ANÁLISIS DE ERRORES, APLICACIONES Y FUTURO. https://www.peoplematters.com/Archivos/Descargas/GPS.pdf. Accessed: 2023-11-21. | spa |
dc.relation.references | Diseño y Maquetación Dpto. Marketing y Comunicación Management Solu tions -España Fotografıas Archivo fotográfico de Management Solutions iS tock. https : / / www . managementsolutions . com / sites / default / files / publicaciones/esp/machine-learning.pdf. Accessed: 2023-11-21. | spa |
dc.relation.references | Timothy O Hodson. «Root mean square error (RMSE) or mean absolute error (MAE): when to use them or not». 2022. | spa |
dc.relation.references | R Suárez et al. Robot Operating System (ROS). https://www.aer-automation. com/wp-content/uploads/2022/03/ROS_articuloAER.pdf. Accessed: 2023- 11-21. | spa |
dc.relation.references | Muertos en accidentes de tránsito en 2021 en Colombia - Colombia - ELTIEMPO.COM. url: https://www.eltiempo.com/colombia/muertos-en accidentes-de-transito-en-2021-en-colombia-646470 | spa |
dc.relation.references | ARL SURA - Riesgos Laborales - ARL - La accidentalidad vial: un proble ma mundial. url: https : / / www . arlsura . com / index . php / centro - de - legislacion-sp-26862/73-centro-de-documentacion-anterior/seguridad vial/1474-la-accidentalidad-vial-un-problema-mundial. | spa |
dc.relation.references | Luis Montoro González Ana Martí-Belda Bertolín Ignacio Lijarcio Patricia Bo só Consuelo López y Raül Viladrich Castellanas José Suárez Reyes. «Coche Autónomo, seguridad vial y formación de conductores Realizado por: Grupo de investigadores INTRAS». En: (). | spa |
dc.relation.references | Mª Loreto et al. «"Implicaciones de la introducción del vehículo autónomo en España"». En: (2014). | spa |
dc.relation.references | Mayank Raj y Narendra V G. «Deep Neural Network approach for navigation of Autonomous Vehicles». En: 2021 6th International Conference for Conver gence in Technology (I2CT). 2021, págs. 1-4. doi: 10.1109/I2CT51068.2021. 9418189. | spa |
dc.relation.references | Julio Alfredo Capdevila. «El Sistema de Posicionamiento Global (GPS)». En: (2018). | spa |
dc.relation.references | Rathin Chandra Shit y Suraj Sharma. «Localization for Autonomous Vehicle: Analysis of Importance of IoT Network Localization for Autonomous Vehicle Applications». En: 2018 International Conference on Applied Electromagnetics, Signal Processing and Communication (AESPC). Vol. 1. 2018, págs. 1-6. doi: 10.1109/AESPC44649.2018.9033329. | spa |
dc.relation.references | Liang Li et al. «Hierarchical Neighborhood Based Precise Localization for Inte lligent Vehicles in Urban Environments». En: IEEE Transactions on Intelligent Vehicles 1.3 (2016), págs. 220-229. doi: 10.1109/TIV.2017.2654065. | spa |
dc.relation.references | Wei Liu. «Improvement of Navigation of Mobile Robotics Based on IoT Sys tem». En: 2021 IEEE International Conference on Robotics, Automation and Artificial Intelligence (RAAI). 2021, págs. 69-72. doi: 10.1109/RAAI52226. 2021.9507826. | spa |
dc.relation.references | Azhar Mahmood, Asim Baig y Qaisar Ahsan. «Real time localization of mo bile robotic platform via fusion of Inertial and Visual Navigation System». En: 2012 International Conference of Robotics and Artificial Intelligence. 2012, págs. 40-44. doi: 10.1109/ICRAI.2012.6413407. | spa |
dc.relation.references | Isao Parra-Tsunekawa, Javier Ruiz-del-Solar y Paul Vallejos. «A Kalman-filtering based Approach for Improving Terrain Mapping in off-road Autonomous Vehi cles». En: Journal of Intelligent & Robotic Systems 78.3 (jun. de 2015), págs. 577-591. issn: 1573-0409. doi: 10.1007/s10846-014-0087-9. url: https://doi.org/ 10.1007/s10846-014-0087-9. | spa |
dc.relation.references | J. S. Pershina, S. Ya. Kazdorf y A. V. Lopota. «Methods of Mobile Robot Visual Navigation and Environment Mapping». En: Optoelectronics, Instru mentation and Data Processing 55.2 (mar. de 2019), págs. 181-188. issn: 1934- 7944. doi: 10.3103/S8756699019020109. url: https://doi.org/10.3103/ S8756699019020109. | spa |
dc.relation.references | Kajal Sharma. «Improved visual SLAM: a novel approach to mapping and localization using visual landmarks in consecutive frames». En: Multimedia Tools and Applications 77.7 (abr. de 2018), págs. 7955-7976. issn: 1573-7721. doi: 10 . 1007 / s11042 - 017 - 4694 - x. url: https : / / doi . org / 10 . 1007 / s11042-017-4694-x. | spa |
dc.relation.references | Haoran Song. «The Application of Computer Vision in Responding to the Emergencies of Autonomous Driving». En: 2020 International Conference on Computer Vision, Image and Deep Learning (CVIDL). 2020, págs. 1-5. doi: 10.1109/CVIDL51233.2020.00008. | spa |
dc.relation.references | Pablo De Cristóforis et al. «Hybrid vision-based navigation for mobile robots in mixed indoor/outdoor environments». En: Pattern Recognition Letters 53 (feb. de 2015), págs. 118-128. issn: 01678655. doi: 10.1016/j.patrec.2014. 10.010. | spa |
dc.relation.references | L. Emmi et al. «A hybrid representation of the environment to improve auto nomous navigation of mobile robots in agriculture». En: Precision Agriculture 22.2 (abr. de 2021), págs. 524-549. issn: 1573-1618. doi: 10.1007/s11119- 020-09773-9. url: https://doi.org/10.1007/s11119-020-09773-9. | spa |
dc.relation.references | Taihú Pire, Javier Corti y Guillermo Grinblat. «Online Object Detection and Localization on Stereo Visual SLAM System». En: Journal of Intelligent & Robotic Systems 98.2 (mayo de 2020), págs. 377-386. issn: 1573-0409. doi:10.1007/s10846-019-01074-2. url: https://doi.org/10.1007/s10846- 019-01074-2. | spa |
dc.relation.references | Gui-Hai Li y Song-Lin Chen. «Visual Slam in Dynamic Scenes Based on Object Tracking and Static Points Detection». En: Journal of Intelligent Robotic Systems 104 (2 feb. de 2022), pág. 33. issn: 0921-0296. doi: 10.1007/s10846- 021- 01563- 3. url: https://link.springer.com/10.1007/s10846- 021- 01563-3. | spa |
dc.relation.references | Su-Yong An, Lae-Kyoung Lee y Se-Young Oh. «Ceiling vision-based active SLAM framework for dynamic and wide-open environments». En: Autonomous Robots 40.2 (feb. de 2016), págs. 291-324. issn: 1573-7527. doi: 10 . 1007 / s10514-015-9453-0. url: https://doi.org/10.1007/s10514-015-9453-0. | spa |
dc.relation.references | Seung-Hun Kim et al. «Outdoor Navigation of a Mobile Robot Using Diffe rential GPS and Curb Detection». En: Proceedings 2007 IEEE International Conference on Robotics and Automation. 2007, págs. 3414-3419. doi: 10.1109/ ROBOT.2007.364000. | spa |
dc.relation.references | Wei Wu y Wei Min. «The Mobile Robot GPS Position Based on Neural Net work Adaptive Kalman Filter». En: 2009 International Conference on Compu tational Intelligence and Natural Computing. Vol. 1. 2009, págs. 26-29. doi: 10.1109/CINC.2009.257. | spa |
dc.relation.references | Nicolas Souli et al. «GNSS Location Verification in Connected and Autonomous Vehicles Using in-Vehicle Multimodal Sensor Data Fusion». En: 2020 22nd International Conference on Transparent Optical Networks (ICTON). 2020, págs. 1-4. doi: 10.1109/ICTON51198.2020.9203087. | spa |
dc.relation.references | Andres Leonardo Bayona y Silvia Takahashi Rodriguez. Comparative study of SAC and PPO in multi-agent reinforcement learning using unity ML-agents. https://repositorio.uniandes.edu.co/server/api/core/bitstreams/ cadff679-f3f3-43fa-a543-d6313c0a4932/content. Accessed: 2023-11-19. | spa |
dc.relation.references | Bharathan Balaji et al. «DeepRacer: Autonomous Racing Platform for Expe rimentation with Sim2Real Reinforcement Learning». En: 2020 IEEE Interna tional Conference on Robotics and Automation (ICRA). 2020, págs. 2746-2754. doi: 10.1109/ICRA40945.2020.9197465. | spa |
dc.relation.references | Lydia A. Garza-Coello et al. «AWS DeepRacer: A Way to Understand and Apply the Reinforcement Learning Methods». En: 2023 IEEE Global Engi neering Education Conference (EDUCON). 2023, págs. 1-3. doi: 10 . 1109 / EDUCON54358.2023.10125166. | spa |
dc.relation.references | Sistema de control de navegación aplicado a vehículo autónomo. url: https: //rdu.unc.edu.ar/handle/11086/12888. | spa |
dc.relation.references | OPS/OMS | Nuevo informe de la OMS destaca que los progresos han sido insuficientes en abordar la falta de seguridad en las vías de tránsito del mundo. url: https : / / www3 . paho . org / hq / index . php ? option = com _ content & view = article & id = 14857 : new - who - report - highlights - insufficient - progress-to-tackle-lack-of-safety-on-the-world-s-roads&Itemid= 1926&lang=es. | spa |
dc.relation.references | Vehículos autónomos: Resultados de la encuesta Delphi sobre su impacto y adopción en ciudades de América Latina y el Caribe | Publications. url: https: //publications.iadb.org/publications/spanish/document/Vehiculos Autonomos-Resultados-de-la-encuesta-Delphi-sobre-su-impacto-y adopcion-en-ciudades-de-America-Latina-y-el-Caribe.pdf. | spa |
dc.relation.references | Abdulmajid Murad y Jae-Young Pyun. «Deep Recurrent Neural Networks for Human Activity Recognition». En: Sensors 17.11 (2017). issn: 1424-8220. doi: 10.3390/s17112556. url: https://www.mdpi.com/1424-8220/17/11/2556. | spa |
dc.relation.references | Ciudades - Desarrollo Sostenible. url: https://www.un.org/sustainabledevelopment/ es/cities/. | spa |
dc.relation.references | Edgar Andrés Toquica Giraldo, Fredy Yubeimar Pérez Mojica y Mauricio An dres Rincón Figueroa. «La seguridad vial en Bogotá DC Desde la teorıa de la organización y administración pública periodo 2010–2019». En: (). | spa |
dc.relation.references | Artificial Intelligence: A National Strategic Initiative. Palgrave Macmillan Sin gapore, 2021. | spa |
dc.relation.references | Nikita Silaparasetty. «Machine Learning vs. Deep Learning». En: Machine Learning Concepts with Python and the Jupyter Notebook Environment: Using Tensorflow 2.0. Berkeley, CA: Apress, 2020, págs. 21-23. isbn: 978-1-4842-5967- 2. doi: 10.1007/978-1-4842-5967-2_4. url: https://doi.org/10.1007/ 978-1-4842-5967-2_4. | spa |
dc.relation.references | Mukesh Ghimire. «A study of deep reinforcement learning in autonomous ra cing using DeepRacer car». Tesis doct. University of Mississippi, 2021 | spa |
dc.relation.references | Mohit Sewak. Deep reinforcement learning: Frontiers of artificial intelligence. Singapore: Springer Singapore, 2019. | spa |
dc.relation.references | Kuryati Kipli et al. «Deep learning applications for oil palm tree detection and counting». En: Smart Agricultural Technology 5 (2023). issn: 2772-3755. doi: https : / / doi . org / 10 . 1016 / j . atech . 2023 . 100241. url: https : //www.sciencedirect.com/science/article/pii/S2772375523000710. | spa |
dc.relation.references | Qué son las redes neuronales? Tipos y funciones. es. https://www.inesdi. com/blog/que- son- las- redes- neuronales/. Accessed: 2023-9-4. Abr. de 2022. | spa |
dc.relation.references | Siddharth Sharma, Simone Sharma y Anidhya Athaiya. ACTIVATION FUN CTIONS IN NEURAL NETWORKS. https://www.ijeast.com/papers/ 310-316,Tesma412,IJEAST.pdf. Accessed: 2023-9-4. | spa |
dc.relation.references | https://medium.com/@shrutijadon/survey-on-activation-functions for-deep-learning-9689331ba092. Accessed: 2023 | spa |
dc.relation.references | Jesus Alberto Valero-Matas y Angie de la Barrera. «The Autonomous Car: A better future?» En: Universidad Valladolid (2020). doi: https : / / doi . org / 10 . 24197 / st . 1 . 2020 . 136 - 158. url: https : / / www . google . com / url?sa=t&rct=j&q=&esrc=s&source=web&cd=&ved=2ahUKEwiGwq7L6Y BAxWnm2oFHd3RBXcQFnoECBkQAQ&url=https%3A%2F%2Fdialnet.unir. | spa |
dc.relation.references | Amazon Web Services). AWS DeepRacer - Guia para Desarrolladores. 2018. url: https://docs.aws.amazon.com/es_es/deepracer/latest/developerguide/ awsracerdg.pdf#what-is-deepracer. | spa |
dc.relation.references | ¿Qué es AWSDeepRacer. url: https : / / docs . aws . amazon . com / es _ es / deepracer/latest/developerguide/what-is-deepracer.html. | spa |
dc.relation.references | Bitcraze. en. https://www.bitcraze.io/about/bitcraze/. Accessed: 2023- 9-4. | spa |
dc.relation.references | Lighthouse positioning deck. en. https : / / www . bitcraze . io / products / lighthouse-positioning-deck/. Accessed: 2023-9-4. | spa |
dc.relation.references | https://www.bitcraze.io/documentation/hardware/lighthouse_deck/ lighthouse_deck-datasheet.pdf. Accessed: 2023-9-4. | spa |
dc.relation.references | https://www.bitcraze.io/products/crazyradio-pa/. Accessed: 2023-9-4. | spa |
dc.relation.references | Matěj Karásek y Feng Shan. Swarm. en. https://www.bitcraze.io/tag/ swarm/. Accessed: 2023-9-14. | spa |
dc.relation.references | Robotics O. Why ros. https://www.ros.org/blog/why-ros/. url: https://www. ros.org/blog/why-ros/. | spa |
dc.relation.references | O. Robotics. «ROS». En: (). url: https://www.ros.org/. | spa |
dc.relation.references | Fabien Moutarde et al. Vehicle absolute ego-localization from vision, using only pre-existing geo-referenced panoramas. https://minesparis-psl.hal. science/hal-02342259/document. Accessed: 2023-11-19. | spa |
dc.relation.references | Arthur Moreau et al. CoordiNet: uncertainty-aware pose regressor for reliable vehicle localization. https://openaccess.thecvf.com/content/WACV2022/ papers / Moreau _ CoordiNet _ Uncertainty - Aware _ Pose _ Regressor _ for _ Reliable_Vehicle_Localization_WACV_2022_paper.pdf. Accessed: 2023- 11-19. | spa |
dc.relation.references | https://docs.aws.amazon.com/es_es/deepracer/latest/developerguide/ deepracer-track-examples.html. Accessed: 2023-9-6. | spa |
dc.relation.references | Intel ® RealSense TM Product Family D400 Series Datasheet Intel ® RealSen se™ Vision Processor D4, Intel ® RealSense™ Vision. 2022. url: www.intel. com/design/literature.htm.. | spa |
dc.relation.references | Jetson Nano Developer Kit. https : / / developer . nvidia . com / embedded / jetson-nano-developer-kit. Accessed: 2023-11-13. | spa |
dc.relation.youtube | https://www.youtube.com/@juandavidpuerto4316/videos | spa |
dc.rights | Atribución-NoComercial-SinDerivadas 2.5 Colombia | * |
dc.rights.accessrights | info:eu-repo/semantics/openAccess | |
dc.rights.coar | http://purl.org/coar/access_right/c_abf2 | spa |
dc.rights.local | Abierto (Texto Completo) | spa |
dc.rights.uri | http://creativecommons.org/licenses/by-nc-nd/2.5/co/ | * |
dc.subject.keyword | AWS (Amazon Web Services) | spa |
dc.subject.keyword | Bluetooth LE (Low Energy) | spa |
dc.subject.keyword | CNN (Convolutional Neural Network) | spa |
dc.subject.keyword | Machine Learning | spa |
dc.subject.keyword | MAE (Mean Absolute Error) | spa |
dc.subject.keyword | ROS (Robot Operating System) | spa |
dc.subject.lemb | Ingeniería Electrónica | spa |
dc.subject.lemb | Ingeniería | spa |
dc.subject.lemb | Aprendizaje | spa |
dc.subject.proposal | EKF (Filtro de Kalman Extendido) | spa |
dc.subject.proposal | Georreferenciación | spa |
dc.subject.proposal | GPS (Sistema de Posicionamiento Global) | spa |
dc.subject.proposal | Aprendizaje Automático | spa |
dc.title | Sistema de Localización para Vehículos Autónomos Basado en Visión Artificial | spa |
dc.type.coar | http://purl.org/coar/resource_type/c_7a1f | |
dc.type.coarversion | http://purl.org/coar/version/c_ab4af688f83e57aa | |
dc.type.drive | info:eu-repo/semantics/bachelorThesis | |
dc.type.local | Trabajo de grado | spa |
dc.type.version | info:eu-repo/semantics/acceptedVersion |
Archivos
Bloque original
1 - 5 de 6
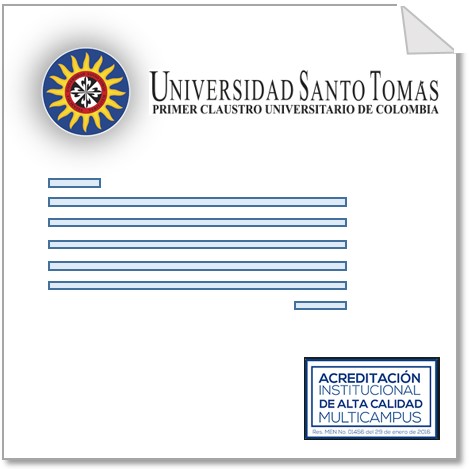
- Nombre:
- 2024cartadederechosdeautor.pdf
- Tamaño:
- 256.75 KB
- Formato:
- Adobe Portable Document Format
- Descripción:
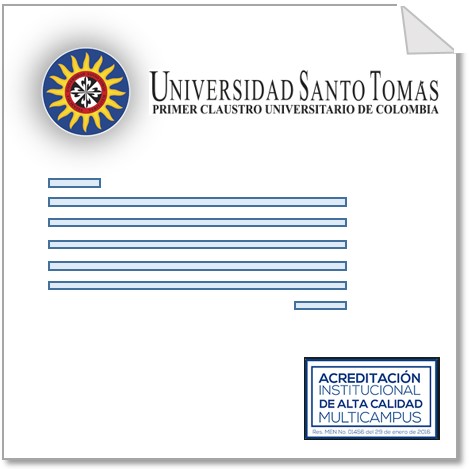
- Nombre:
- 2024cartadefacultad.pdf
- Tamaño:
- 36.3 KB
- Formato:
- Adobe Portable Document Format
- Descripción:
Cargando...
- Nombre:
- 2024juanpuerto.pdf
- Tamaño:
- 70.87 MB
- Formato:
- Adobe Portable Document Format
- Descripción:
Cargando...
- Nombre:
- 2024juanpuerto1.pdf
- Tamaño:
- 173.81 KB
- Formato:
- Adobe Portable Document Format
- Descripción:
Cargando...
- Nombre:
- 2024juanpuerto2.pdf
- Tamaño:
- 1.45 MB
- Formato:
- Adobe Portable Document Format
- Descripción:
Bloque de licencias
1 - 1 de 1
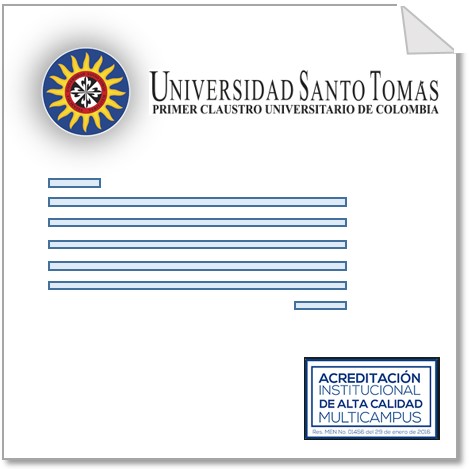
- Nombre:
- license.txt
- Tamaño:
- 807 B
- Formato:
- Item-specific license agreed upon to submission
- Descripción: